Enhancing the Protein Tertiary Structure Prediction by Multiple Sequence Alignment Generation
CoRR(2023)
摘要
The field of protein folding research has been greatly advanced by deep learning methods, with AlphaFold2 (AF2) demonstrating exceptional performance and atomic-level precision. As co-evolution is integral to protein structure prediction, AF2's accuracy is significantly influenced by the depth of multiple sequence alignment (MSA), which requires extensive exploration of a large protein database for similar sequences. However, not all protein sequences possess abundant homologous families, and consequently, AF2's performance can degrade on such queries, at times failing to produce meaningful results. To address this, we introduce a novel generative language model, MSA-Augmenter, which leverages protein-specific attention mechanisms and large-scale MSAs to generate useful, novel protein sequences not currently found in databases. These sequences supplement shallow MSAs, enhancing the accuracy of structural property predictions. Our experiments on CASP14 demonstrate that MSA-Augmenter can generate de novo sequences that retain co-evolutionary information from inferior MSAs, thereby improving protein structure prediction quality on top of strong AF2.
更多查看译文
关键词
protein tertiary structure prediction,alignment generation,multiple sequence
AI 理解论文
溯源树
样例
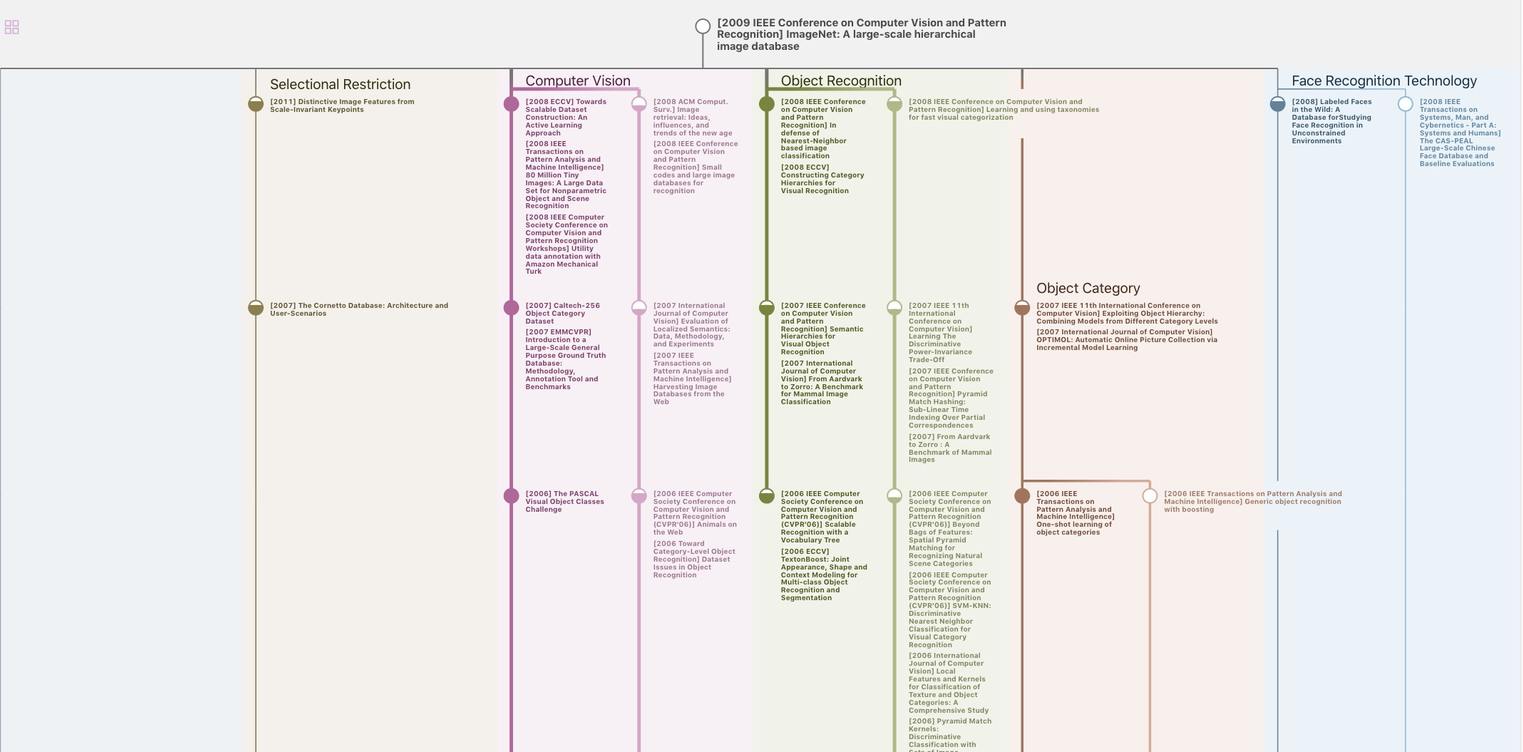
生成溯源树,研究论文发展脉络
Chat Paper
正在生成论文摘要