A Study of Situational Reasoning for Traffic Understanding.
KDD 2023(2023)
摘要
Intelligent Traffic Monitoring (ITMo) technologies hold the potential for improving road safety/security and for enabling smart city infrastructure. Understanding traffic situations requires a complex fusion of perceptual information with domain-specific and causal commonsense knowledge. Whereas prior work has provided benchmarks and methods for traffic monitoring, it remains unclear whether models can effectively align these information sources and reason in novel scenarios. To address this assessment gap, we devise three novel text-based tasks for situational reasoning in the traffic domain: i) BDD-QA, which evaluates the ability of Language Models (LMs) to perform situational decision-making, ii) TV-QA, which assesses LMs' abilities to reason about complex event causality, and iii) HDT-QA, which evaluates the ability of models to solve human driving exams. We adopt four knowledge-enhanced methods that have shown generalization capability across language reasoning tasks in prior work, based on natural language inference, commonsense knowledge-graph self-supervision, multi-QA joint training, and dense retrieval of domain information. We associate each method with a relevant knowledge source, including knowledge graphs, relevant benchmarks, and driving manuals. In extensive experiments, we benchmark various knowledge-aware methods against the three datasets, under zero-shot evaluation; we provide in-depth analyses of model performance on data partitions and examine model predictions categorically, to yield useful insights on traffic understanding, given different background knowledge and reasoning strategies.
更多查看译文
关键词
traffic understanding,zero-shot evaluation,language models,question answering
AI 理解论文
溯源树
样例
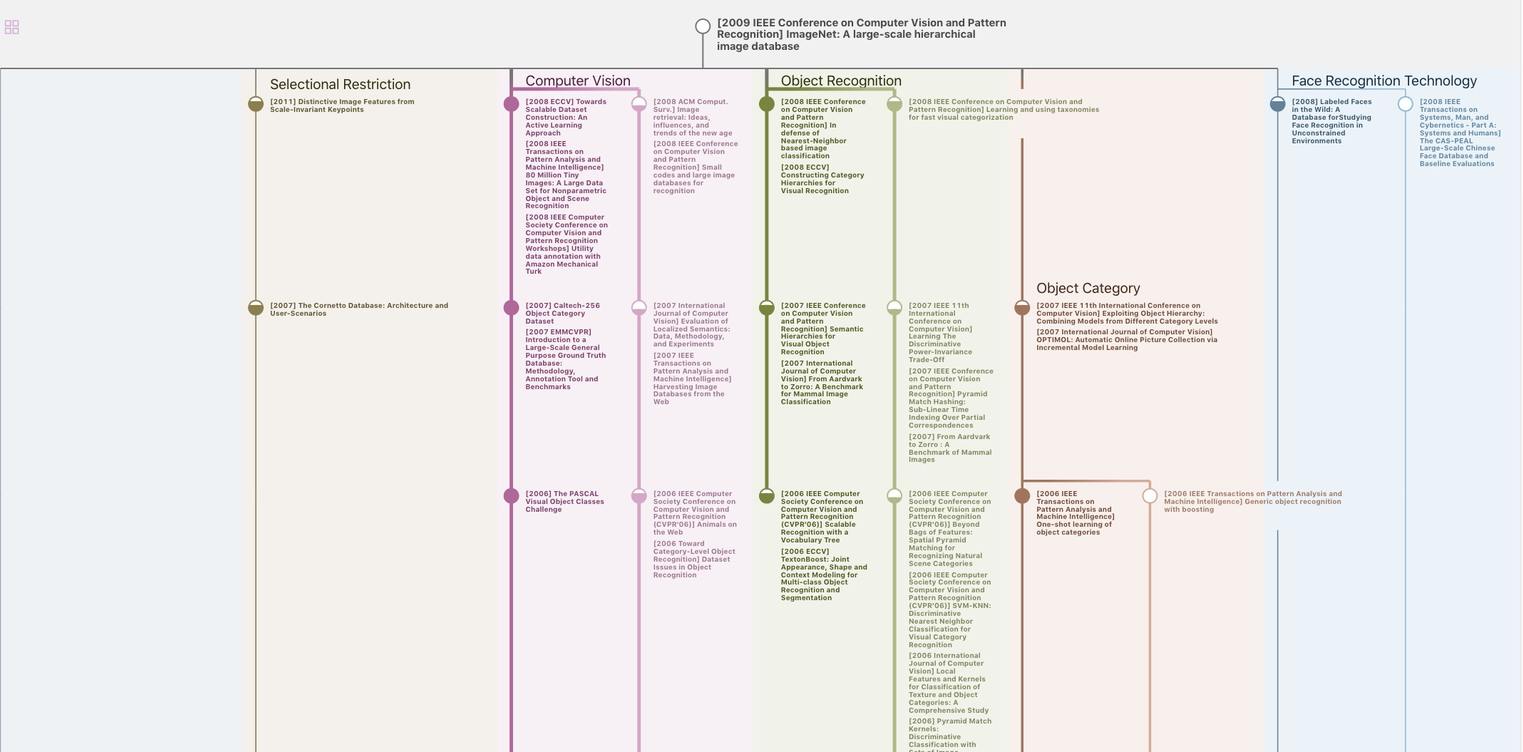
生成溯源树,研究论文发展脉络
Chat Paper
正在生成论文摘要