Spatial Implicit Neural Representations for Global-Scale Species Mapping
CoRR(2023)
摘要
Estimating the geographical range of a species from sparse observations is a challenging and important geospatial prediction problem. Given a set of locations where a species has been observed, the goal is to build a model to predict whether the species is present or absent at any location. This problem has a long history in ecology, but traditional methods struggle to take advantage of emerging large-scale crowdsourced datasets which can include tens of millions of records for hundreds of thousands of species. In this work, we use Spatial Implicit Neural Representations (SINRs) to jointly estimate the geographical range of 47k species simultaneously. We find that our approach scales gracefully, making increasingly better predictions as we increase the number of species and the amount of data per species when training. To make this problem accessible to machine learning researchers, we provide four new benchmarks that measure different aspects of species range estimation and spatial representation learning. Using these benchmarks, we demonstrate that noisy and biased crowdsourced data can be combined with implicit neural representations to approximate expert-developed range maps for many species.
更多查看译文
关键词
spatial implicit neural representations,mapping,species,global-scale
AI 理解论文
溯源树
样例
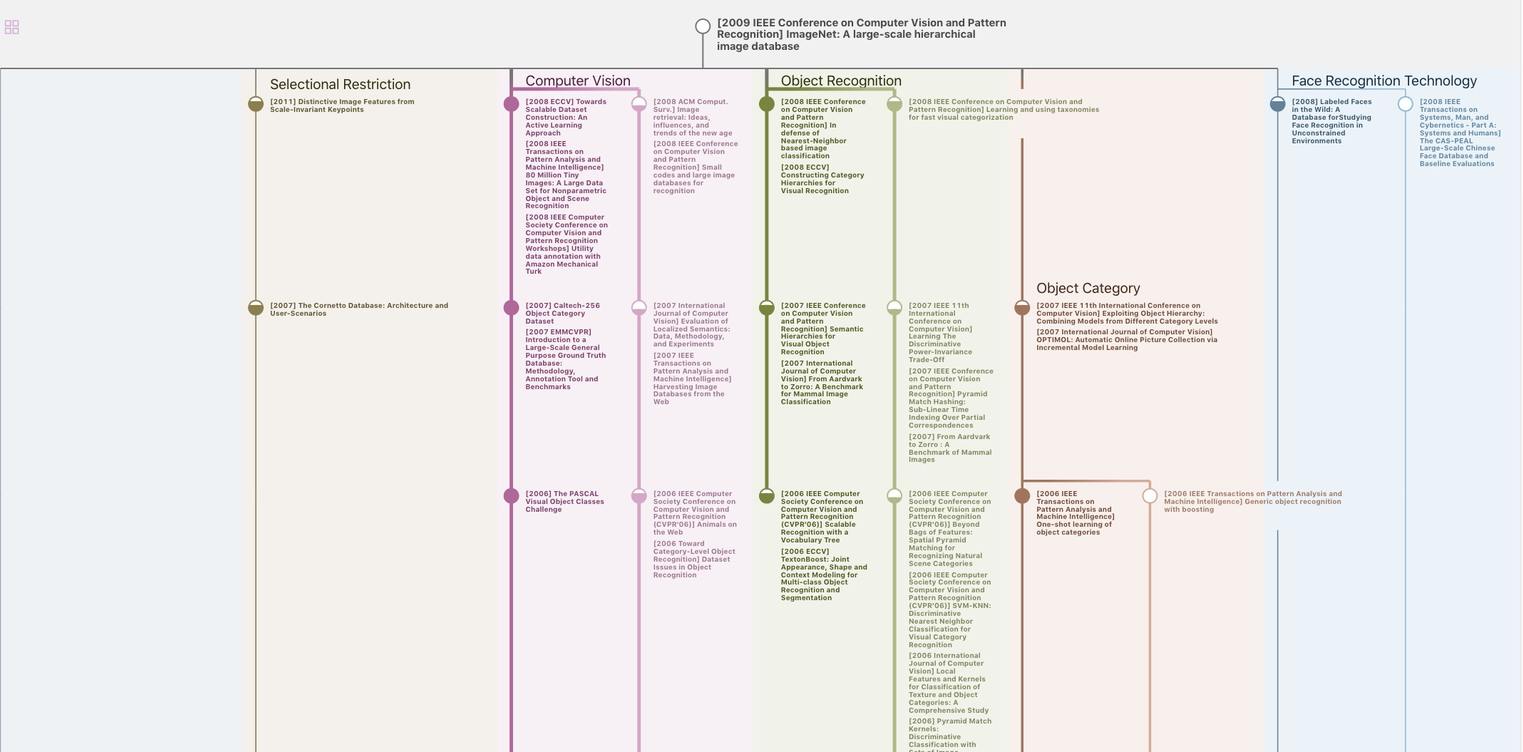
生成溯源树,研究论文发展脉络
Chat Paper
正在生成论文摘要