Team: PULSAR at ProbSum 2023: PULSAR: Pre-training with Extracted Healthcare Terms for Summarising Patients' Problems and Data Augmentation with Black-box Large Language Models.
CoRR(2023)
摘要
Medical progress notes play a crucial role in documenting a patient's hospital journey, including his or her condition, treatment plan, and any updates for healthcare providers. Automatic summarisation of a patient's problems in the form of a problem list can aid stakeholders in understanding a patient's condition, reducing workload and cognitive bias. BioNLP 2023 Shared Task 1A focuses on generating a list of diagnoses and problems from the provider's progress notes during hospitalisation. In this paper, we introduce our proposed approach to this task, which integrates two complementary components. One component employs large language models (LLMs) for data augmentation; the other is an abstractive summarisation LLM with a novel pre-training objective for generating the patients' problems summarised as a list. Our approach was ranked second among all submissions to the shared task. The performance of our model on the development and test datasets shows that our approach is more robust on unknown data, with an improvement of up to 3.1 points over the same size of the larger model.
更多查看译文
关键词
extracted healthcare terms,language models,data augmentation,summarising,patients,pre-training,black-box
AI 理解论文
溯源树
样例
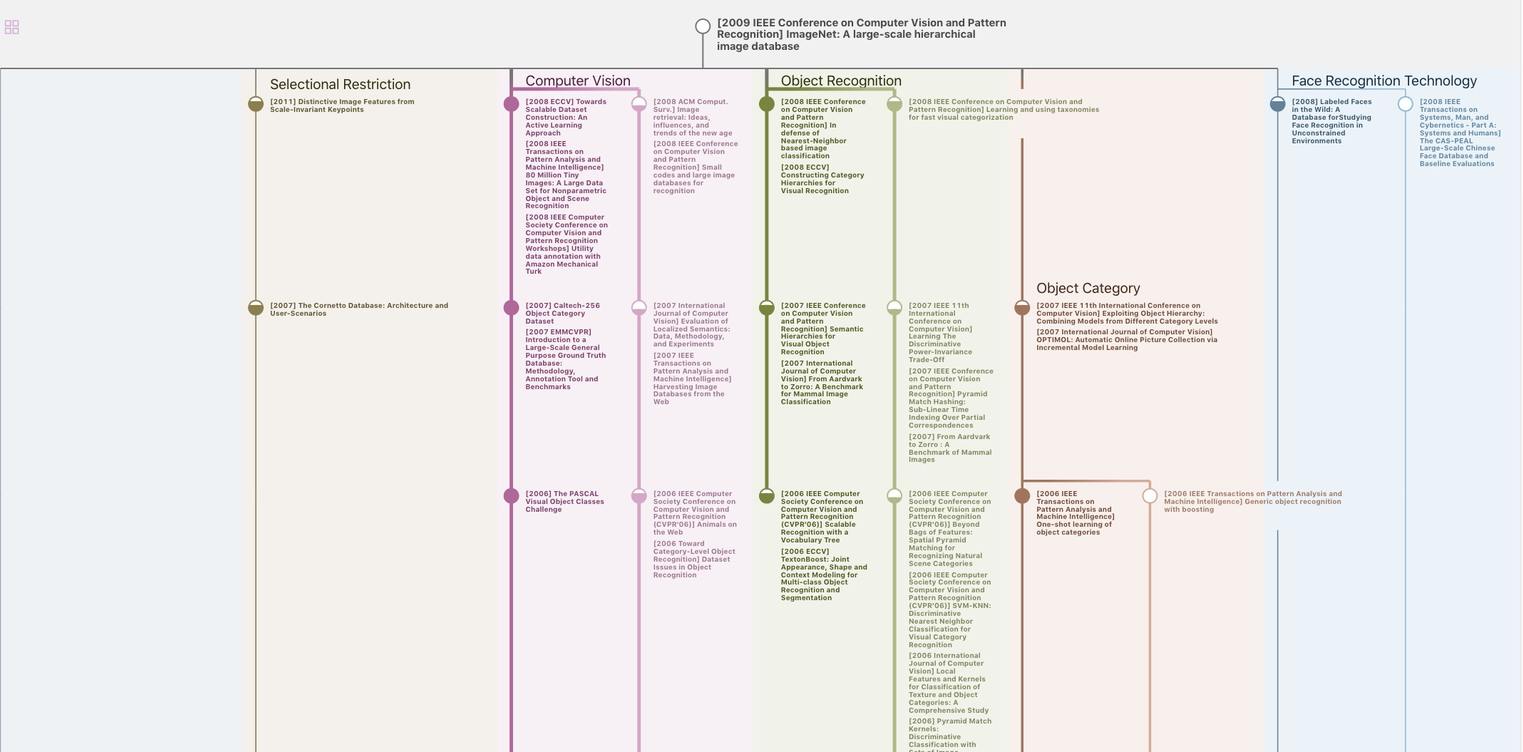
生成溯源树,研究论文发展脉络
Chat Paper
正在生成论文摘要