Sensitivity-Aware Finetuning for Accuracy Recovery on Deep Learning Hardware
CoRR(2023)
摘要
Existing methods to recover model accuracy on analog-digital hardware in the presence of quantization and analog noise include noise-injection training. However, it can be slow in practice, incurring high computational costs, even when starting from pretrained models. We introduce the Sensitivity-Aware Finetuning (SAFT) approach that identifies noise sensitive layers in a model, and uses the information to freeze specific layers for noise-injection training. Our results show that SAFT achieves comparable accuracy to noise-injection training and is 2x to 8x faster.
更多查看译文
关键词
accuracy recovery,deep learning
AI 理解论文
溯源树
样例
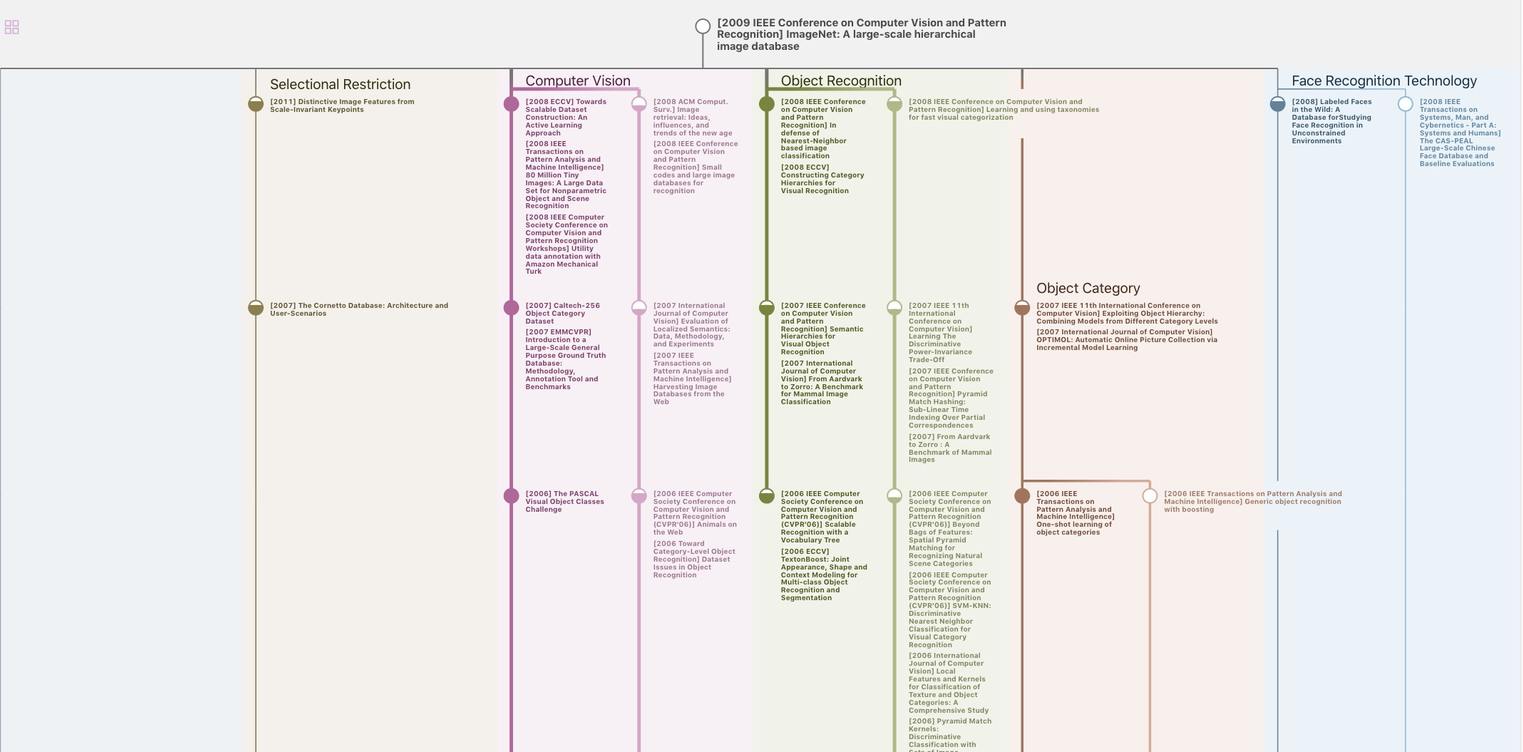
生成溯源树,研究论文发展脉络
Chat Paper
正在生成论文摘要