Arabic Sign Language Gesture Classification Using Deer Hunting Optimization with Machine Learning Model
CMC-COMPUTERS MATERIALS & CONTINUA(2023)
摘要
Sign language includes the motion of the arms and hands to communicate with people with hearing disabilities. Several models have been available in the literature for sign language detection and classification for enhanced outcomes. But the latest advancements in computer vision enable us to perform signs/gesture recognition using deep neural networks. This paper introduces an Arabic Sign Language Gesture Classification using Deer Hunting Optimization with Machine Learning (ASLGC-DHOML) model. The presented ASLGC-DHOML technique mainly concentrates on recognis-ing and classifying sign language gestures. The presented ASLGC-DHOML model primarily pre-processes the input gesture images and generates feature vectors using the densely connected network (DenseNet169) model. For ges-ture recognition and classification, a multilayer perceptron (MLP) classifier is exploited to recognize and classify the existence of sign language gestures. Lastly, the DHO algorithm is utilized for parameter optimization of the MLP model. The experimental results of the ASLGC-DHOML model are tested and the outcomes are inspected under distinct aspects. The comparison anal-ysis highlighted that the ASLGC-DHOML method has resulted in enhanced gesture classification results than other techniques with maximum accuracy of 92.88%.
更多查看译文
关键词
Machine learning,sign language recognition,multilayer perceptron,deer hunting optimization,densenet
AI 理解论文
溯源树
样例
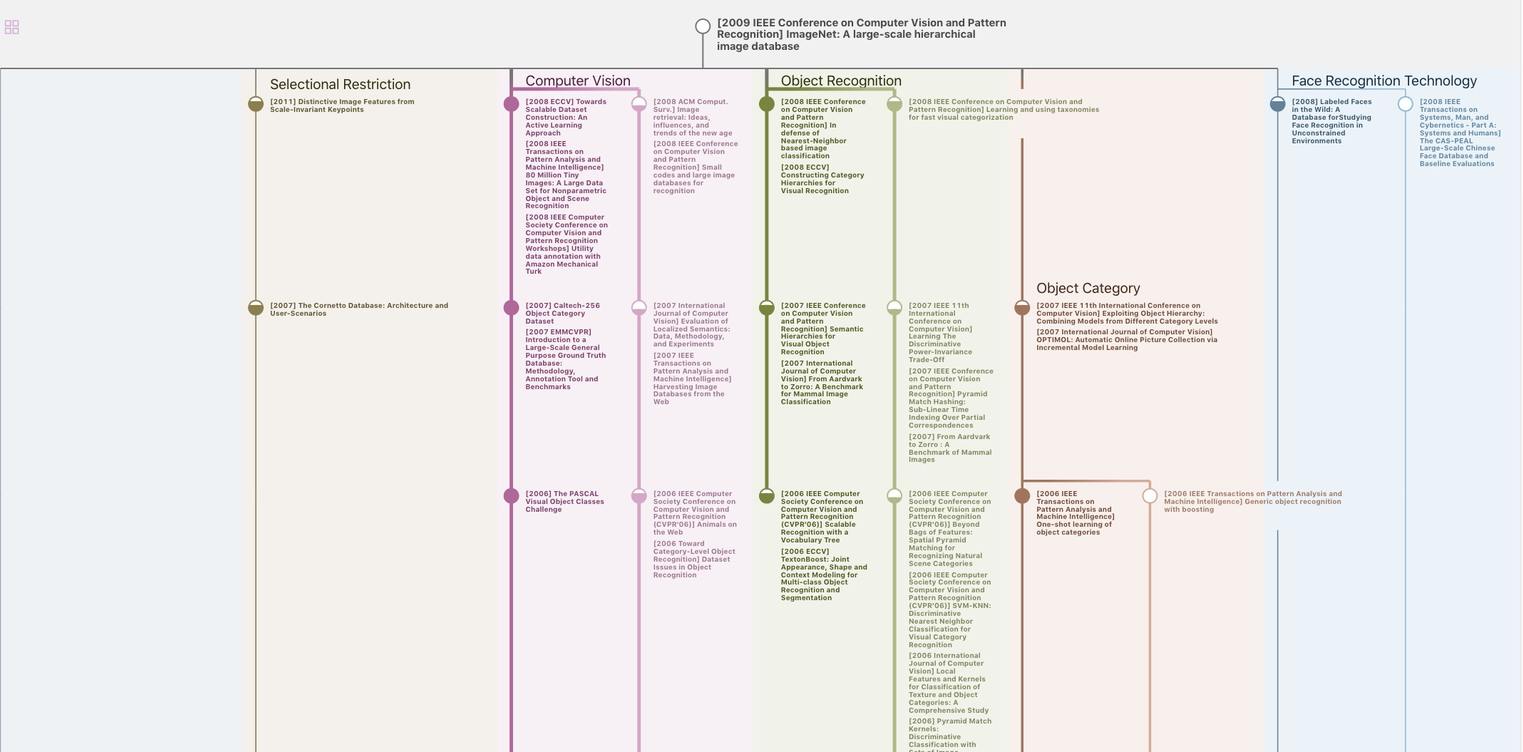
生成溯源树,研究论文发展脉络
Chat Paper
正在生成论文摘要