A Contrastive Learning Framework for Detecting Anomalous Behavior in Commodity Trading Platforms
APPLIED SCIENCES-BASEL(2023)
摘要
For bulk commodity, stock, and e-commerce platforms, it is necessary to detect anomalous behavior for the security of users and platforms. Anomaly-detection methods currently used on these platforms train a model for each user since different users have different habits. However, the model cannot be trained adequately due to insufficient individual user behavior data. In this study, to utilize information between users and avoid underfitting, we propose a contrastive learning framework to train a complete global model (GM) for anomaly detection in a trading platform. By confusing the data between different users to generate negative samples, the model can learn the differences between users by contrastive learning. To reduce the need for individual user behavior data, this framework uses a GM instead of a model for each user to learn similarities between users. Experiments on four datasets show that models trained using our framework achieve better area-under-the-curve (AUC) scores than do the original models, proving that contrastive learning and GM are useful for anomaly detection in trading platforms.
更多查看译文
关键词
anomalous behavior detection,contrastive learning,trading platforms
AI 理解论文
溯源树
样例
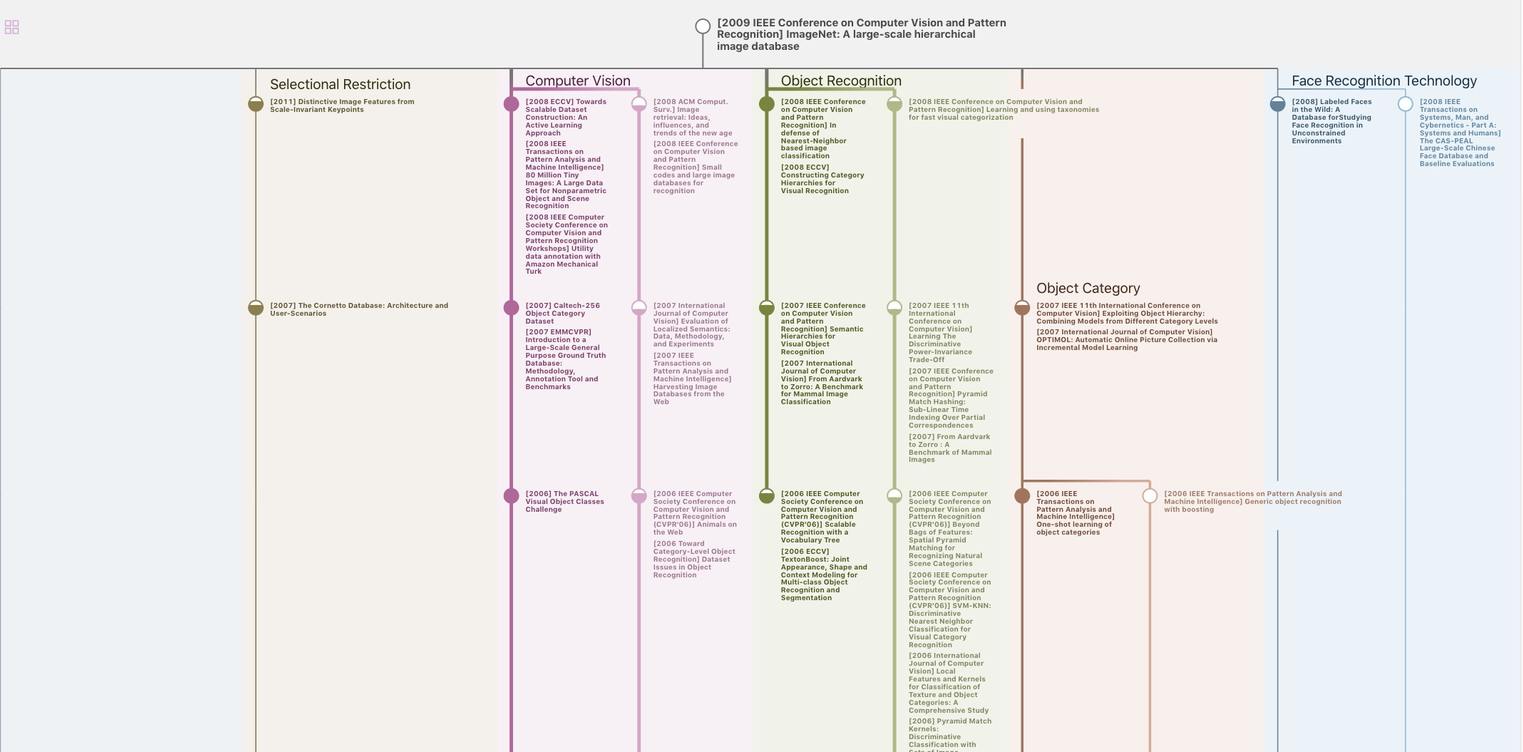
生成溯源树,研究论文发展脉络
Chat Paper
正在生成论文摘要