Yolo-Light: Remote Straw-Burning Smoke Detection Based on Depthwise Separable Convolution and Channel Attention Mechanisms
APPLIED SCIENCES-BASEL(2023)
摘要
Straw burning is a long-term environmental problem in China's agricultural production. At present, China relies mainly on satellite remote sensing positioning and manual patrol to detect straw burning, which are inefficient. Due to the development of machine learning, target detection technology can be used for the detection of straw burning, but the current research does not take into account the various scenarios of straw burning and the deployment of object detection models. Therefore, a lightweight network based on depthwise separable convolution and channel attention mechanisms is proposed to detect straw-burning smoke at a remote distance. Various regional and crop-burning smoke datasets were collected to make the algorithm more robust. The lightweight network was applied to automatically identify and detect straw-burning smoke in surveillance videos. The experiment showed that the amount of light network parameter was only 4.76 M, and the calculation performance was only 11.2 Gflops. For the intelligent detection of straw-burning smoke, performance verification accuracy was improved by 2.4% compared with Yolov5s. Meanwhile, the detection speed on the embedded Jetson Xavier NX device can reach 28.65 FPS, which is 24.67% better than the Yolov5s. This study proposes a lightweight target detection network, providing a possible method for developing low-cost, rapid straw-burning smoke detection equipment.
更多查看译文
关键词
straw-burning smoke detection,lightweight network,depthwise separable convolution,channel attention
AI 理解论文
溯源树
样例
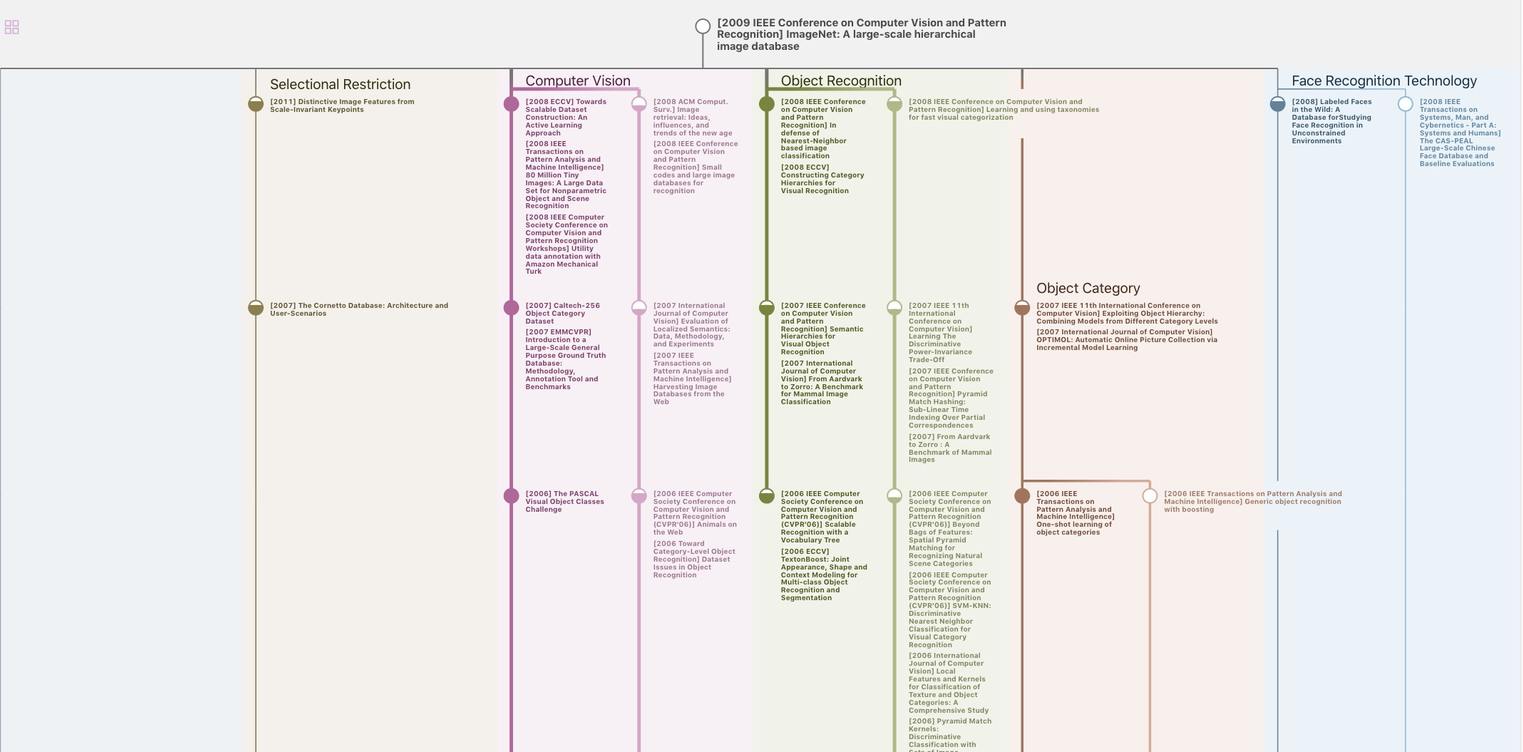
生成溯源树,研究论文发展脉络
Chat Paper
正在生成论文摘要