Machine Learning-Based Framework for Predicting Creep Rupture Life of Modified 9Cr-1Mo Steel
APPLIED SCIENCES-BASEL(2023)
摘要
Efficient and accurate predictions of creep rupture life are essential for ensuring the integrity of high-temperature components. In this work, a machine learning-based framework is developed for the quick screening of crucial features and accurate prediction of the creep rupture life of modified 9Cr-1Mo steels. A feature screening protocol based on correlation filtering and sequential feature selection techniques is established for identifying critical features that significantly affect the prediction performance from a set of numerous descriptors. Moreover, several machine learning algorithms are employed for model training to examine their ability to map the complex nonlinear interactions between multivariate features and creep life. The results show that the test stress, test temperature, tempering time, and the contents of S and Cr are identified as the crucial features that greatly influence the life prediction performance of modified 9Cr-1Mo steels. Moreover, the Gaussian process regression (GPR) model with these five selected crucial features exhibits the highest prediction accuracy among various machine learning strategies. Finally, an additional dataset out of model training and testing is used to further validate the efficacy of the constructed GPR model. The validated results demonstrate that most creep data are distributed inside the two-factor band lines. Results from this work show that the developed machine learning framework can offer high accuracy and excellent adaptability in predicting the creep life of modified 9Cr-1Mo steels under various environmental conditions.
更多查看译文
关键词
machine learning,creep,feature screening,life prediction,9Cr-1Mo steel
AI 理解论文
溯源树
样例
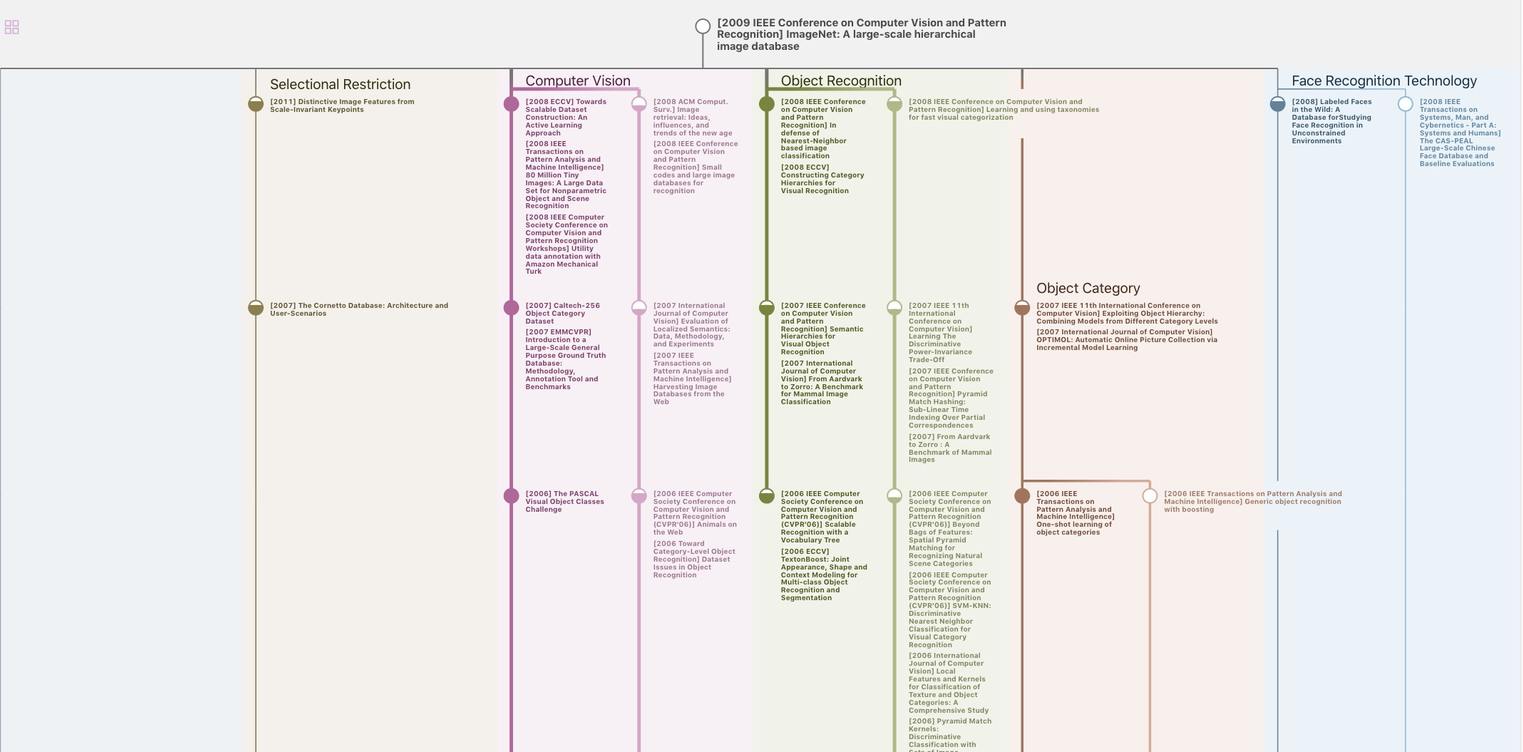
生成溯源树,研究论文发展脉络
Chat Paper
正在生成论文摘要