Use of stacked Gaussian processes regression method to improve prediction of groundwater solute transport model
JOURNAL OF HYDROLOGY(2023)
摘要
The complex groundwater solute transport model can generally be divided into several coupled submodels, the systematic error is inevitable in each submodel due to the unreasonable simplification or biased representation of the true groundwater environment system. The submodels' systematic errors and their propagation lead to great challenge for reliable model predictions. To improve the predictive performance of groundwater solute transport model, the Stacked Gaussian processes regression (GPR) method was proposed to quantify and reduce the error propagation among the submodels in this study. Stacked GPR corrects the structure errors of groundwater model submodel by submodel gradually. Two case studies are performed to demonstrate the effectiveness of Stacked GPR method, including a synthetical groundwater transport case and a laboratory NaCl solute transport experiment. The results demonstrated that Stacked GPR can reduce the error propagation among submodels and improve model's predictive performance apparently. Moreover, it is more feasible to correct the structure error of each submodel in sequence by Stacked GPR than to correct the lumped structure error by traditional GPR for a component-based groundwater solute transport model. The errors in prior submodels could aggravate the complexity of the error of the posterior submodel though error propagation, which increases the difficulty of constructing an effective error-correcting model.
更多查看译文
关键词
Stacked Gaussian processes regression,Model structure error,Systematic error,Groundwater solute transport model,Prediction
AI 理解论文
溯源树
样例
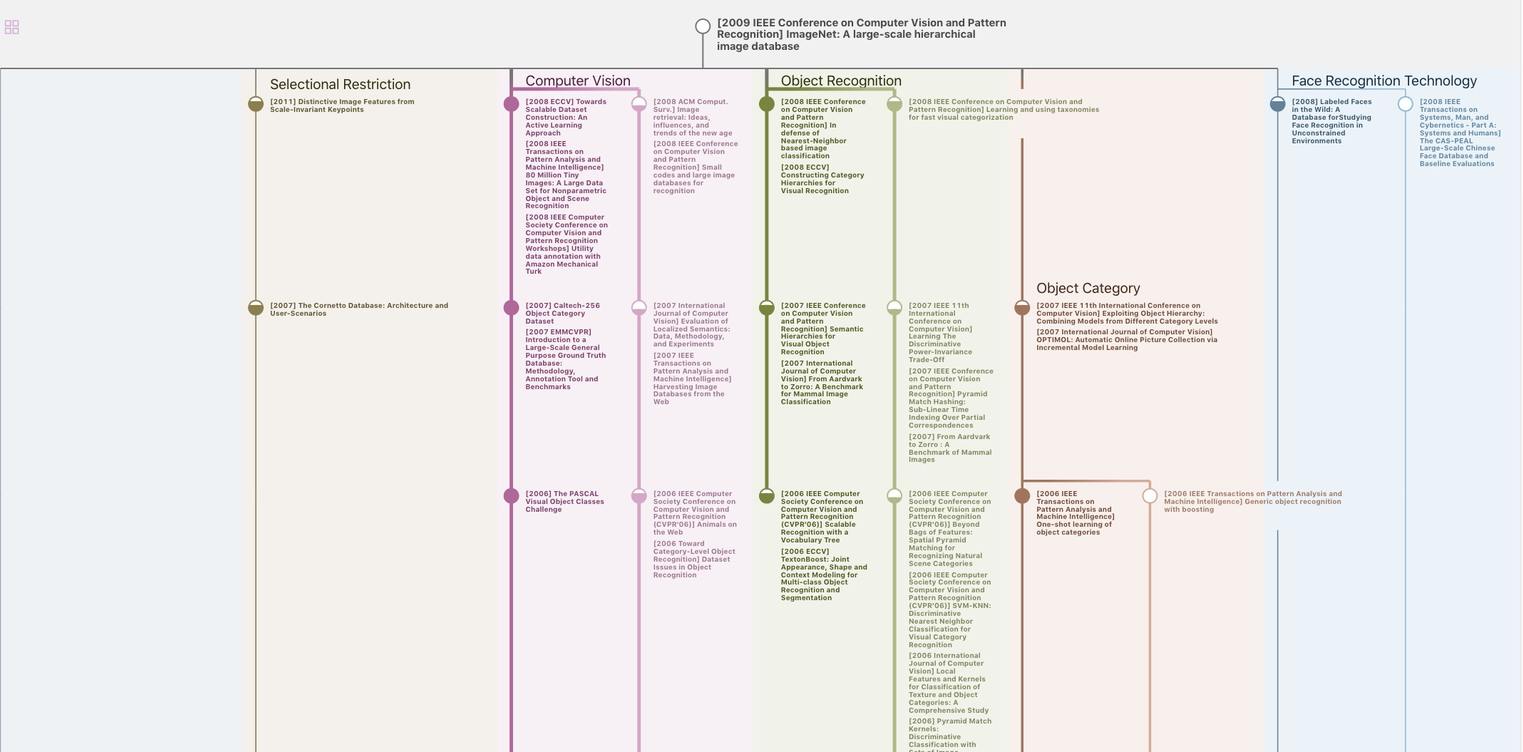
生成溯源树,研究论文发展脉络
Chat Paper
正在生成论文摘要