An improved temporal convolutional network with attention mechanism for photovoltaic generation forecasting
ENGINEERING APPLICATIONS OF ARTIFICIAL INTELLIGENCE(2023)
摘要
Forecasting renewable energy generation is challenging due to non-stationary and intricate stochastic prop-erties, with a significant impact on grid operation. Although various methods have been developed for this purpose, the abundance of noise in original data limits the models' ability to successfully model different features and make accurate predictions. Furthermore, current research tends to prioritize accuracy over usability. To address this, our paper proposes a novel multivariate time series forecasting model comprising temporal convolutions with residual structures, where the channel attention mechanism recalibrates important differences among features, and traditional convolution is improved to depth-wise convolution and the latest network architecture. The model enhances the accuracy of predictions while significantly lowering processing costs, making it suitable for deployment on various devices. The proposed model outperforms competing models in a variety of validation tests, according to experiments, with a significant 55% reduction in the mean squared error (MSE) compared to the best-competing model in the photovoltaic generation data set.
更多查看译文
关键词
Photovoltaic power forecast, Channel attention mechanism, Lightweight networks, Time series forecasting
AI 理解论文
溯源树
样例
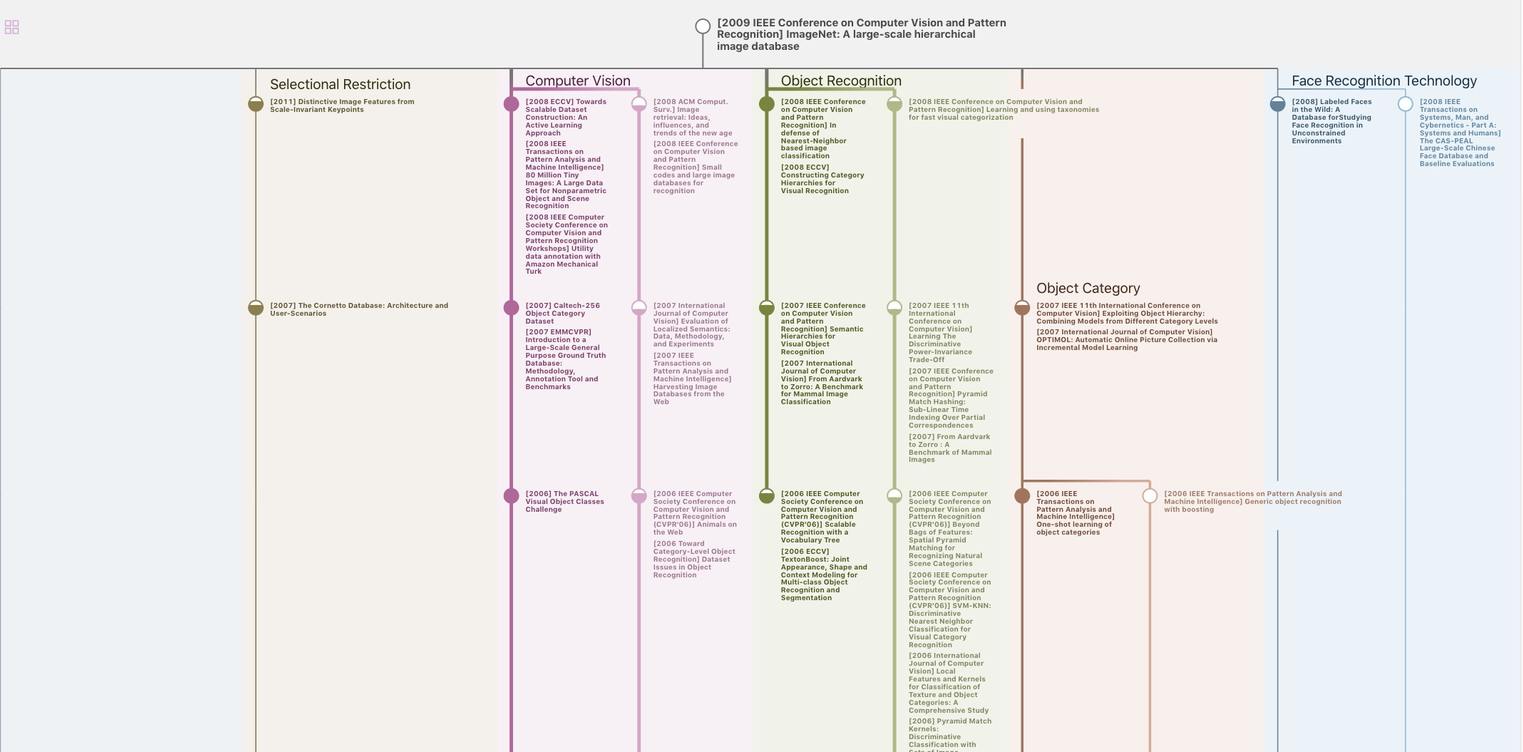
生成溯源树,研究论文发展脉络
Chat Paper
正在生成论文摘要