Influence Mechanism of Crucible Materials on Cleanliness and Inclusion Characteristics of High-Nitrogen Stainless Bearing Steel During Vacuum Carbon Deoxidation
METALLURGICAL AND MATERIALS TRANSACTIONS B-PROCESS METALLURGY AND MATERIALS PROCESSING SCIENCE(2023)
Abstract
High cleanliness is an important guarantee for the long-service of bearing steel. In this study, the effect of crucible materials on cleanliness and inclusion characteristics of high-nitrogen stainless bearing steel (HNSBS) during vacuum carbon deoxidation was comprehensively investigated by microstructure characterization and thermodynamic analysis. The results showed that the ultimate O contents using MgO·Al 2 O 3 , MgO, and ZrO 2 (MA, M, and Z) crucibles could be decreased from about 0.0060 to 0.0028, 0.0012, and 0.0022 wt pct, respectively. The ranking of crucible thermodynamic stability during vacuum carbon deoxidation was M < MA < Z, representing the decreasing of oxygen transfer rate due to crucible decomposition. The deoxidation rate of carbon–oxygen reaction increased with the decreasing of CO partial pressure, and the ranking of deoxidation rate using various crucibles was Z < MA < M. Meanwhile, the main oxide inclusions in steel using MA, M, and Z crucibles transformed from Al 2 O 3 to MgO·Al 2 O 3 , MgO, and ZrO 2 , respectively. The area and average size of inclusions in steel using M crucible were smaller than the others owing to the decreasing of large-size Al 2 O 3 inclusions and the increasing of percentage of low-density Mg-containing inclusions, while the existence of high-density ZrO 2 inclusions in steel using Z crucible restricted the floating and removal of inclusions. Therefore, MgO crucible was more appropriate to melt high-cleanliness HNSBS with lower O content and fewer deleterious inclusions.
MoreTranslated text
Key words
High Speed Steel
求助PDF
上传PDF
View via Publisher
AI Read Science
Must-Reading Tree
Example
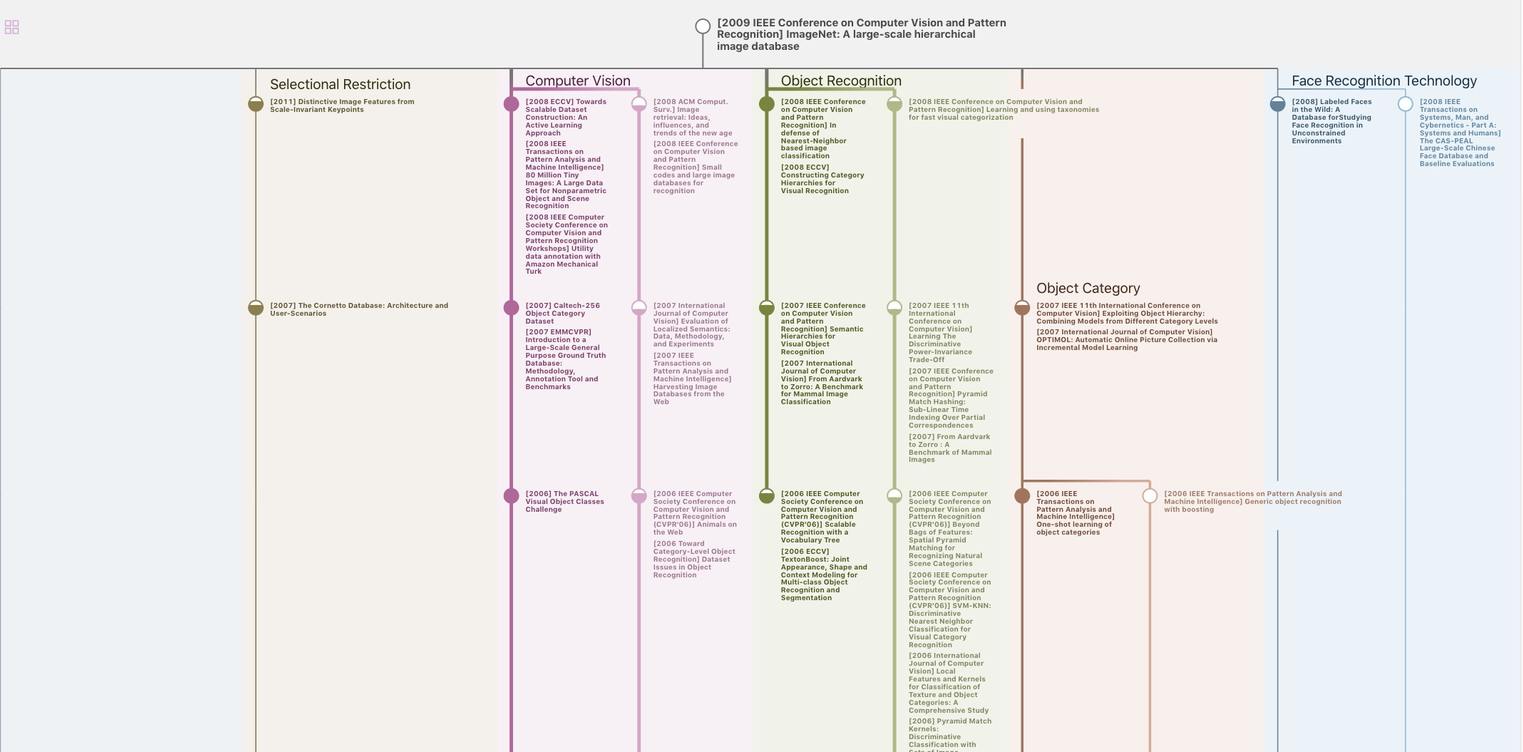
Generate MRT to find the research sequence of this paper
Data Disclaimer
The page data are from open Internet sources, cooperative publishers and automatic analysis results through AI technology. We do not make any commitments and guarantees for the validity, accuracy, correctness, reliability, completeness and timeliness of the page data. If you have any questions, please contact us by email: report@aminer.cn
Chat Paper
Summary is being generated by the instructions you defined