Deep learning predictions of unsteady aerodynamic loads on an airfoil model pitched over the entire operating range
PHYSICS OF FLUIDS(2023)
摘要
For the design and certification of wind turbines, it is essential to provide fast and accurate unsteady aerodynamic load prediction models for the whole operational range of angle of attack, up to 180 degrees for vertical-axis and 90 degrees for horizontal-axis wind turbines. This work describes a computationally efficient unsteady forces prediction model based on a deep learning approach, namely the bidirectional long short-term memory (BiLSTM) algorithm, for an airfoil pitched over the full operational range of angles of attack up to 180 degrees. No model has been developed to capture the unsteady forces at high angles of attack. Novel features based on operating conditions and the steady polars of the airfoil are used as inputs for the BiLSTM model. Direct measurements of steady and unsteady forces on a NACA 0021 airfoil model were conducted at reduced frequencies up to 0.075 and a Reynolds number of 120 000 in an open-jet wind tunnel for model learning and testing. The unsteady forces vary significantly from the steady values at high pitching amplitudes and post-stall angles, which, if not accounted for when simulating wind turbine performance, would result in inaccurate predictions. Furthermore, measurements revealed the effect of unsteady vorticity development and shedding on aerodynamic forces under forward and reverse flow conditions. The BiLSTM model is capable of capturing the underlying physics of unsteady aerodynamic forces under extreme operating conditions.
更多查看译文
关键词
unsteady aerodynamic loads,airfoil model,deep
AI 理解论文
溯源树
样例
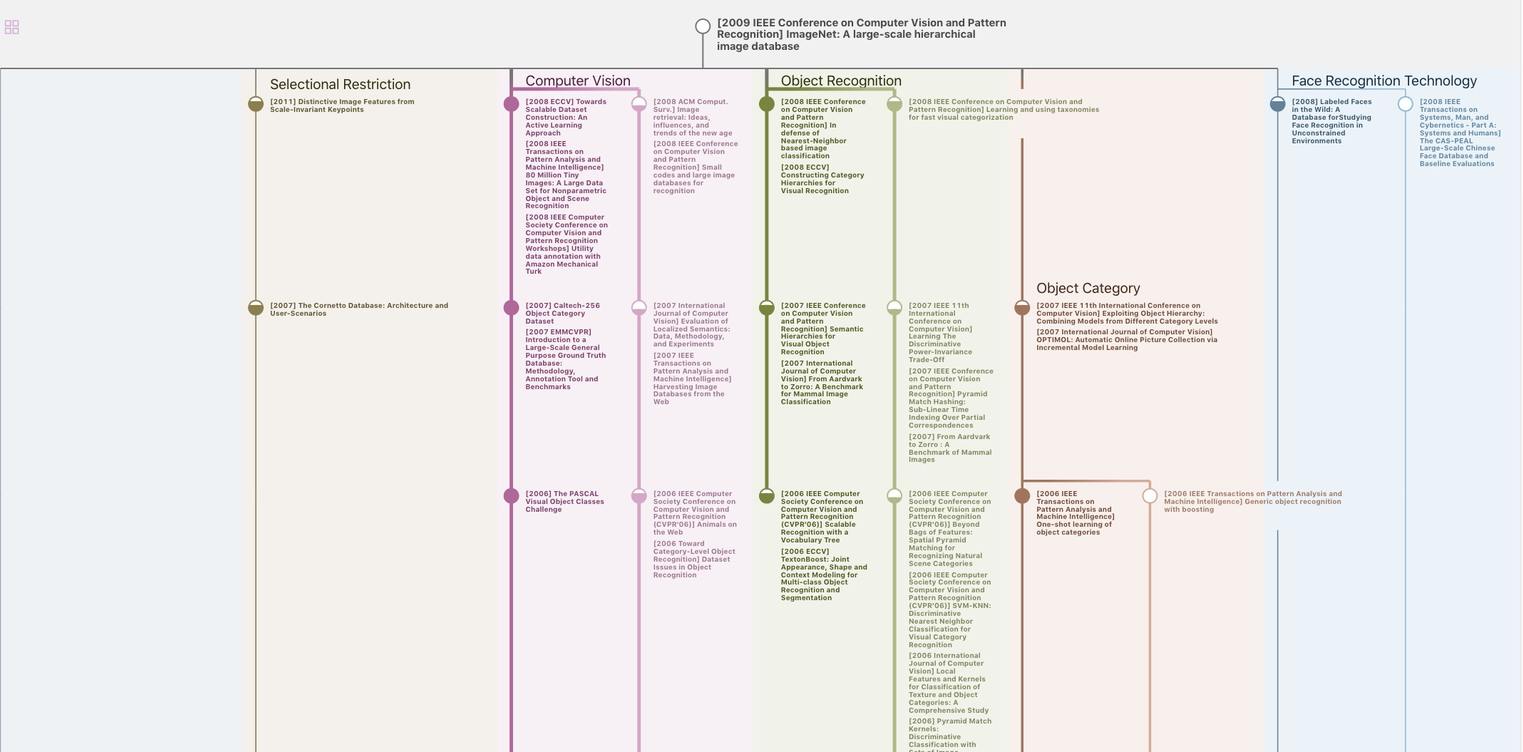
生成溯源树,研究论文发展脉络
Chat Paper
正在生成论文摘要