Uncertainty Quantification of Inflow on Passive Scalar Dispersion in an Urban Environment
ENVIRONMENTAL FLUID MECHANICS(2023)
摘要
Risk assessment, city planning, and emergency response are a few examples of potential applications of numerical simulations of scalar dispersion in urban environments. The complex flow fields and scalar dispersion are determined by the building layout and prevailing meteorological conditions that are highly uncertain. While the fidelity of a numerical model is important in providing an accurate prediction of flow and scalar fields, propagating the uncertain input through numerical models is imperative in those applications. However, it is uncommon to quantify input uncertainties due to expensive computational cost of high fidelity simulations such as large eddy simulations (LES) and Reynolds-averaged Navier–Stokes (RANS). In this work, the uncertain meteorological quantities viz., wind speed and its direction from field measurements are taken as inputs to RANS simulations that use realizable k-ε turbulence model, to investigate their effects on passive scalar dispersion in central London. The mean wind and scalar quantities from RANS are initially validated with wind-tunnel data and compared to large eddy simulations (LES). For comparison with field measurements, the deduced probability density function (pdf) for wind speed and direction from the field are used as inputs for RANS simulations. For a 3-min averaged concentration at a specific receiver location, LES with unsteady wind inputs showed better performance than LES with mean wind input and RANS whereas for 30-min averaged concentration at various receiver locations, performance measures indicated that RANS is better than LES. The latter certainly suggests the importance of considering such uncertainties. The flow variability in every street is quantified using RANS simulations. This demonstrated that approximations used in a fast, low-order street network model may not be necessarily valid for every street of heterogeneous urban canopies, which in turn affects scalar prediction.
更多查看译文
关键词
DAPPLE,Dakota,Large eddy simulation,Reynolds-averaged Navier–Stokes,SIRANE,Wind-tunnel
AI 理解论文
溯源树
样例
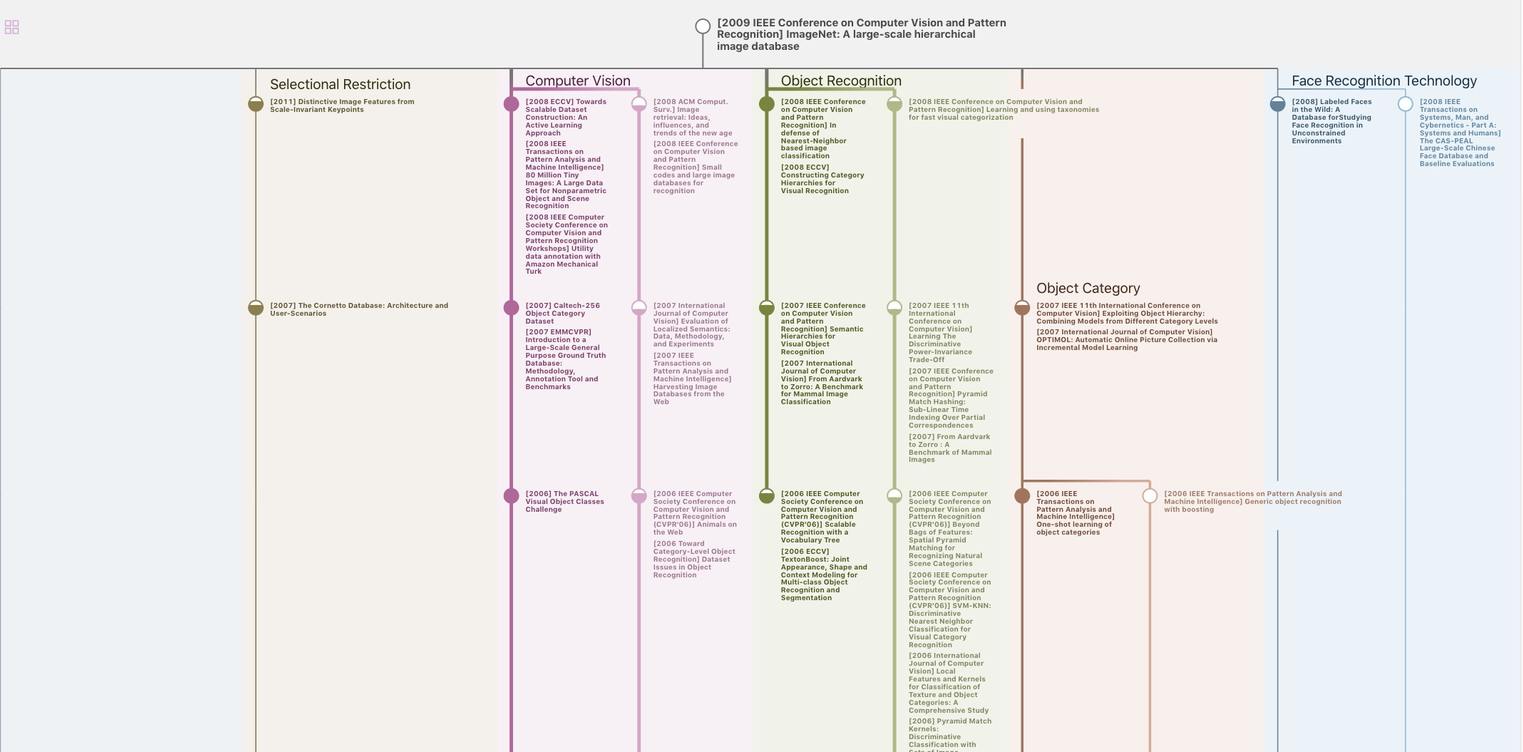
生成溯源树,研究论文发展脉络
Chat Paper
正在生成论文摘要