Sensitivity Analysis of Parameters Governing the Iceberg Draft Through Neural Network-Based Models
Journal of ocean engineering and marine energy(2023)
摘要
Precise estimation of the iceberg draft may significantly reduce the collision risk of deep keel icebergs with the offshore facilities comprising the submarine pipelines, wellheads, communication cables, and hydrocarbon loading equipment crossing the Arctic shallow waters. As such, in this study, the iceberg drafts were simulated using a self-adaptive machine learning (ML) algorithm entitled self-adaptive extreme learning machine (Sa-ELM) for the first time, to the best of our knowledge. Initially, the parameters governing the iceberg drafts were specified, and then nine Sa-ELM models were defined using these parameters. To test and train the Sa-ELM models, a comprehensive dataset was constructed, where 60% of the dataset was utilized for model training and 40% for model validation. In addition, several hyper parameters have been optimized during the training procedure to obtain the most accurate results. The superior Sa-ELM model and the most influencing input parameters were determined by conducting a sensitivity analysis. The comparison of the premium Sa-ELM model with the artificial neural network (ANN) and extreme learning machine (ELM) models demonstrated that the Sa-ELM model had the highest level of accuracy and correlation as well as the lowest degree of complexity. Ultimately, a Sa-ELM-based equation was presented to estimate the iceberg draft in practical applications. Graphical abstract
更多查看译文
关键词
Artificial neural network,Machine learning,Iceberg Draft,Subsea assets,Sensitivity analysis,Self-adaptive evolutionary extreme learning machine,Extreme learning machine
AI 理解论文
溯源树
样例
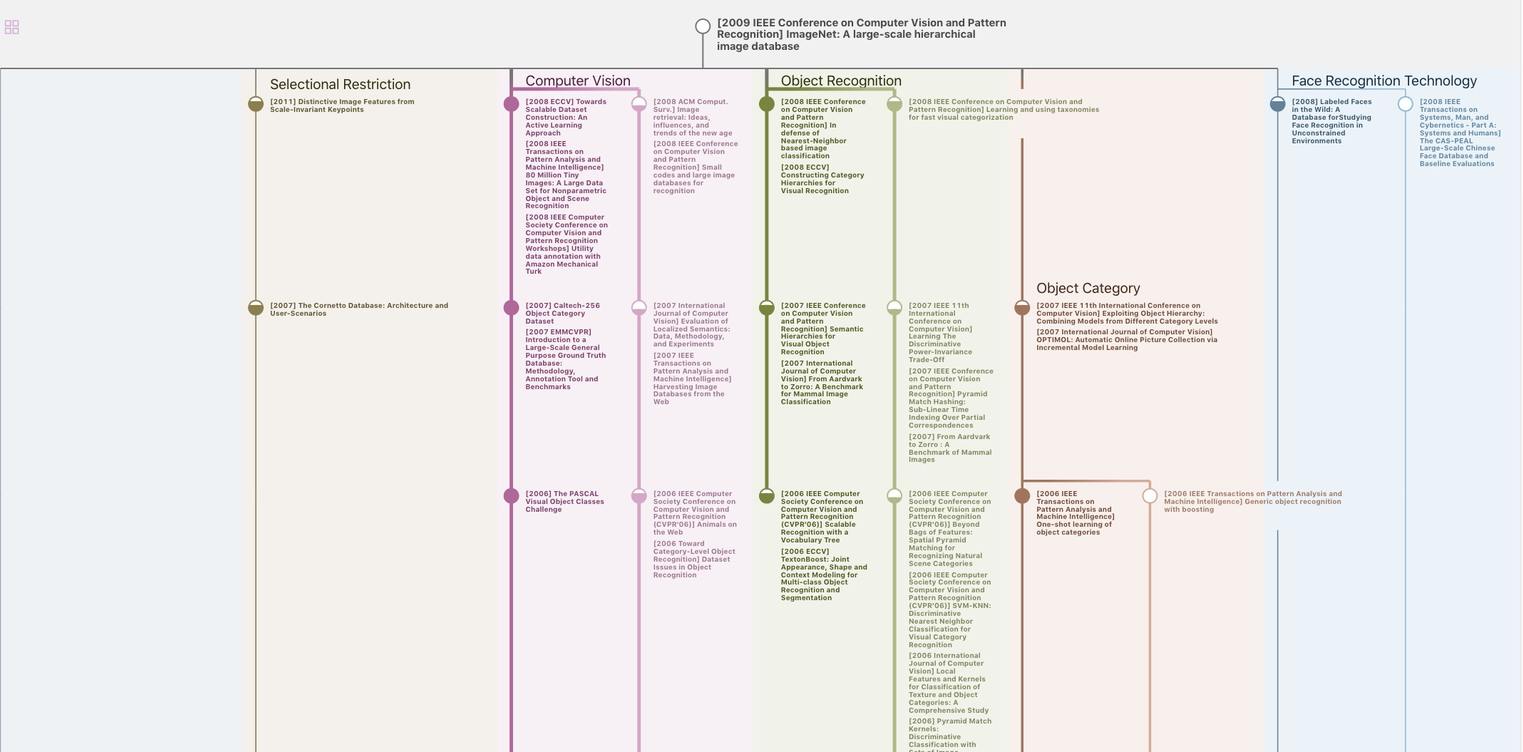
生成溯源树,研究论文发展脉络
Chat Paper
正在生成论文摘要