Self-Constructed Deep Fuzzy Neural Network for Traffic Flow Prediction
ELECTRONICS(2023)
摘要
Traffic flow prediction is a critical component of intelligent transportation systems, especially in the prevention of traffic congestion in urban areas. While significant efforts have been devoted to enhancing the accuracy of traffic prediction, the interpretability of traffic prediction also needs to be considered to enhance persuasiveness, particularly in the era of deep-learning-based traffic cognition. Although some studies have explored interpretable neural networks from the feature and result levels, model-level explanation, which explains the reasoning process of traffic prediction through transparent models, remains underexplored and requires more attention. In this paper, we propose a novel self-constructed deep fuzzy neural network, SCDFNN, for traffic flow prediction with model interpretability. By leveraging recent advances in neuro-symbolic computation for automatic rule learning, SCDFNN learns interpretable human traffic cognitive rules based on deep learning, incorporating two innovations: (1) a new fuzzy neural network hierarchical architecture constructed for spatial-temporal dependences in the traffic feature domain; (2) a modified Wang-Mendel method used to fuse regional differences in traffic data, resulting in adaptive fuzzy-rule weights without sacrificing interpretability. Comprehensive experiments on well-known traffic datasets demonstrate that the proposed approach is comparable to state-of-the-art deep models, and the SCDFNN's unique hierarchical architecture allows for transparency.
更多查看译文
关键词
intelligent transportation system (ITS), traffic flow prediction, hierarchical fuzzy inference systems, fuzzy neural network, modified Wang-Mendel (MWM) method
AI 理解论文
溯源树
样例
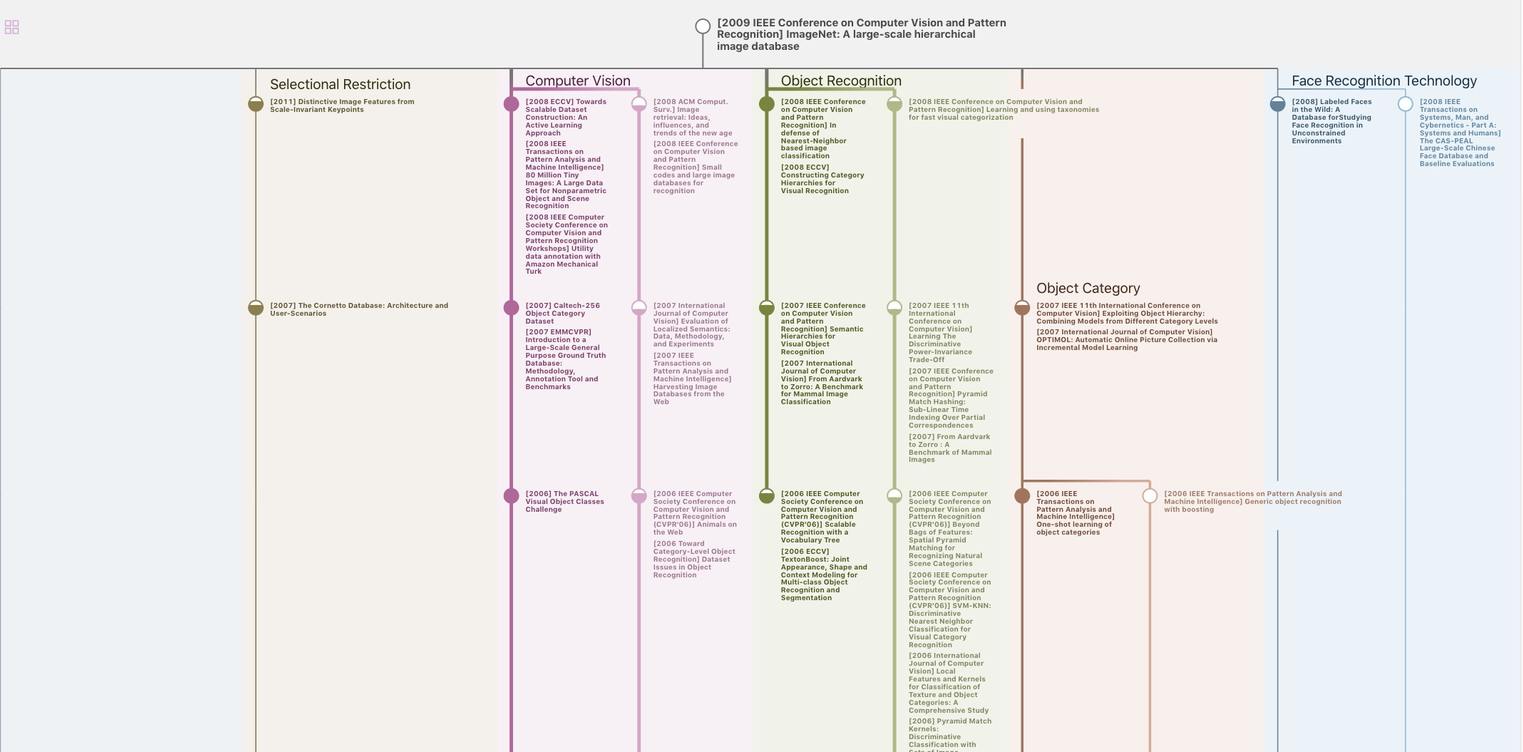
生成溯源树,研究论文发展脉络
Chat Paper
正在生成论文摘要