Computational analysis of performances for a hydrogen enriched compressed natural gas engine' by advanced machine learning algorithms
FUEL(2023)
摘要
The support vector machine along with particle swarm optimization and artificial neural network have already been implemented for the regression study of a hydrogen enriched compressed natural gas (HCNG) fueled spark ignition engine. The main issues were that the support vector machine along with particle swarm optimization requires more computational time while the artificial neural network is sensitive to the hidden layers. Therefore, these algorithms are not effectively suitable for online control of the electronic control unit. The theme of this study is to evaluate the multiple mathematical engine' models with respect to swiftness and accuracy. The experiments have been conducted under a wide range of operating conditions in a heavy-duty SI engine to obtain the data sets. The brake specific fuel consumption, engine torque and NOx emissions have been trained and analyzed by eight different machine learning algorithms along with nineteen sub-models. The effectiveness of these algorithms has been evaluated with respect to the root mean squared error and prediction time. The analysis indicate that the gaussian process regression (GPR) is optimal with respect to the computational efficiency (i.e., RMSE = 0.3823 g/kWh) and time (t < 16.019 sec). Furthermore, it operates without the iteration of the hidden layers. The mean simulation time for the gaussian process regression is 20,192 times lower as compared to the support vector machine along with particle swarm optimization algorithm. Since the gaussian process regression (GPR) is fast, optimal and insensitive to the hidden layers, so it is an ideal tool for online calibration of the electronic control unit.
更多查看译文
关键词
advanced machine learning algorithms,natural gas engine,machine learning algorithms,machine learning,hydrogen
AI 理解论文
溯源树
样例
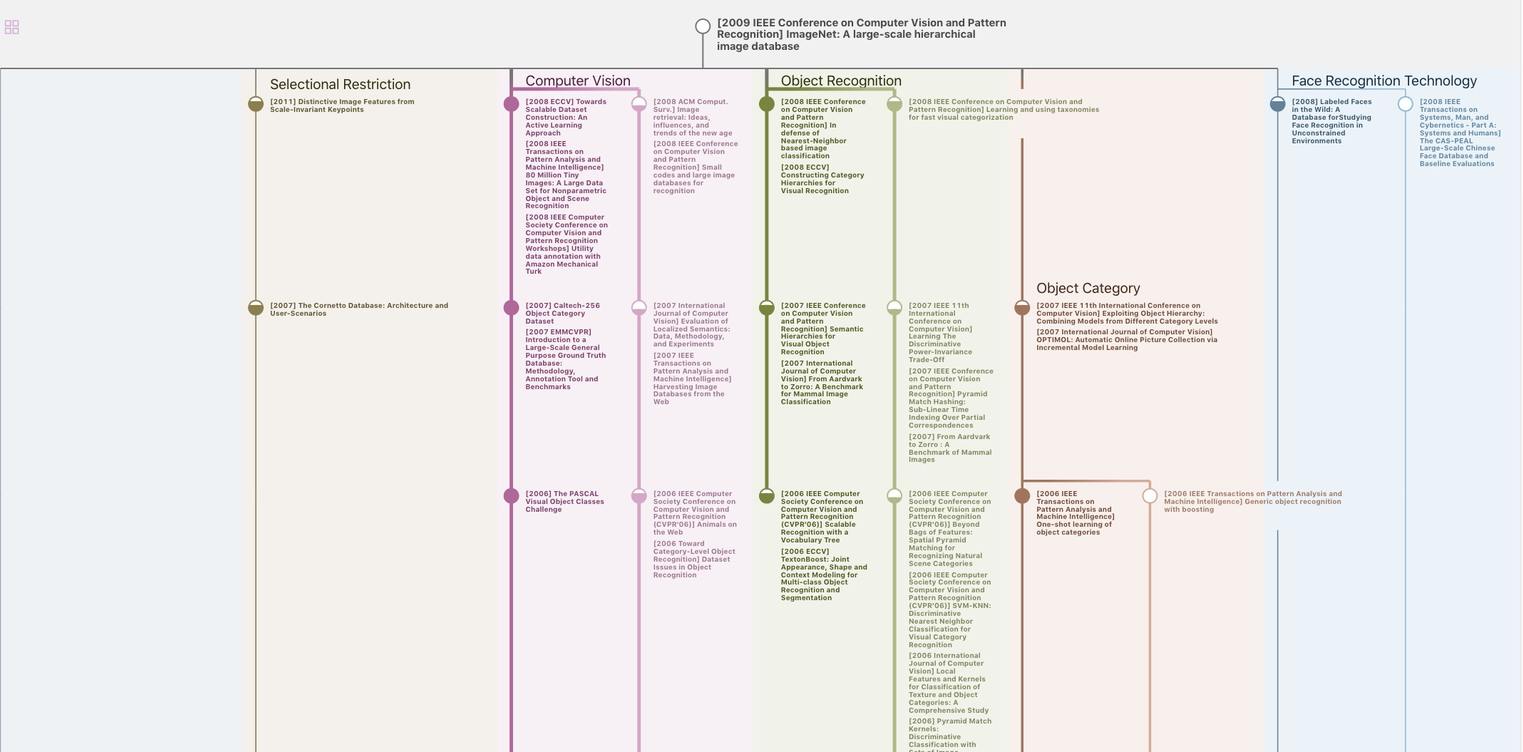
生成溯源树,研究论文发展脉络
Chat Paper
正在生成论文摘要