More than accuracy: end-to-end wind power forecasting that optimises the energy system
ELECTRIC POWER SYSTEMS RESEARCH(2023)
摘要
Weather forecast models are essential for sustainable energy systems. However, forecast accuracy may not be the best metric for developing forecast models. A more or less conservative forecast may be preferred over pure accuracy. For example, forecasting accurately in times of energy-deprived situations may be more important than in times of excess wind power generation. This is not accounted for when learning a wind power forecast without system knowledge. Wind power forecasts directly impact system energy schedules. Therefore, to optimise system costs, our paper proposes a neural network structure for wind power forecasts directly considering varying energy system conditions. To train this neural network optimally, this paper models the system dispatch and their costs as an optimisation problem. Then, this paper connects the neural network with the optimisation model. In this connection, the implicit function theorem and the Karush-Kuhn-Tucker (KKT) conditions provide the system cost gradients during the training of the neurons. A case study using onshore and offshore weather data from Germany and The Netherlands showed forecast errors of system costs reduced by up to 10% with high wind capacity. Wind curtailment was reduced by more than 20% for individual cases but increased overall. Modified cases of the optimal power flow (OPF) with tighter line constraints led to a higher advantage of the proposed method. The approach led to more consistent forecast performance and reduced error variance to 70%.
更多查看译文
关键词
wind,accuracy,end-to-end
AI 理解论文
溯源树
样例
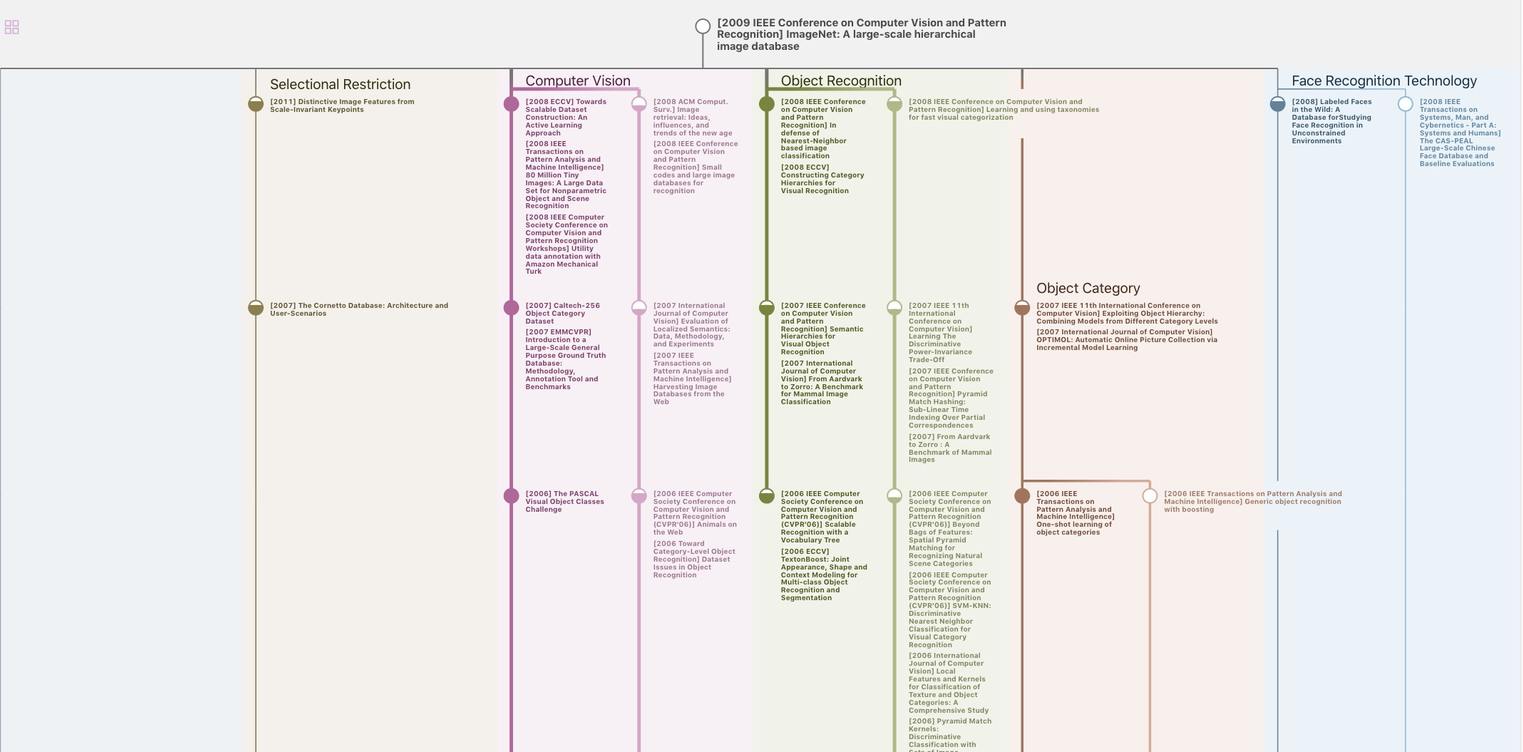
生成溯源树,研究论文发展脉络
Chat Paper
正在生成论文摘要