Predicting the elemental compositions of solid waste using ATR-FTIR and machine learning
FRONTIERS OF ENVIRONMENTAL SCIENCE & ENGINEERING(2023)
摘要
Elemental composition is a key parameter in solid waste treatment and disposal. This study has proposed a method based on infrared spectroscopy and machine learning algorithms that can rapidly predict the elemental composition (C, H, N, S) of solid waste. Both noise and moisture spectral interference that may occur in practical application are investigated. By comparing two feature selection methods and five machine learning algorithms, the most suitable models are selected. Moreover, the impacts of noise and moisture on the models are discussed, with paper, plastic, textiles, wood, and leather as examples of recyclable waste components. The results show that the combination of the feature selection and K -nearest neighbor (KNN) approaches exhibits the best prediction performance and generalization ability. Particularly, the coefficient of determination ( R 2 ) of the validation set, cross validation and test set are higher than 0.93, 0.89, and 0.97 for predicting the C, H, and N contents, respectively. Further, KNN is less sensitive to noise. Under moisture interference, the combination of feature selection and support vector regression or partial least-squares regression shows satisfactory results. Therefore, the elemental compositions of solid waste are quickly and accurately predicted under noise and moisture disturbances using infrared spectroscopy and machine learning algorithms.
更多查看译文
关键词
Elemental composition,Infrared spectroscopy,Machine learning,Moisture interference,Solid waste,Spectral noise
AI 理解论文
溯源树
样例
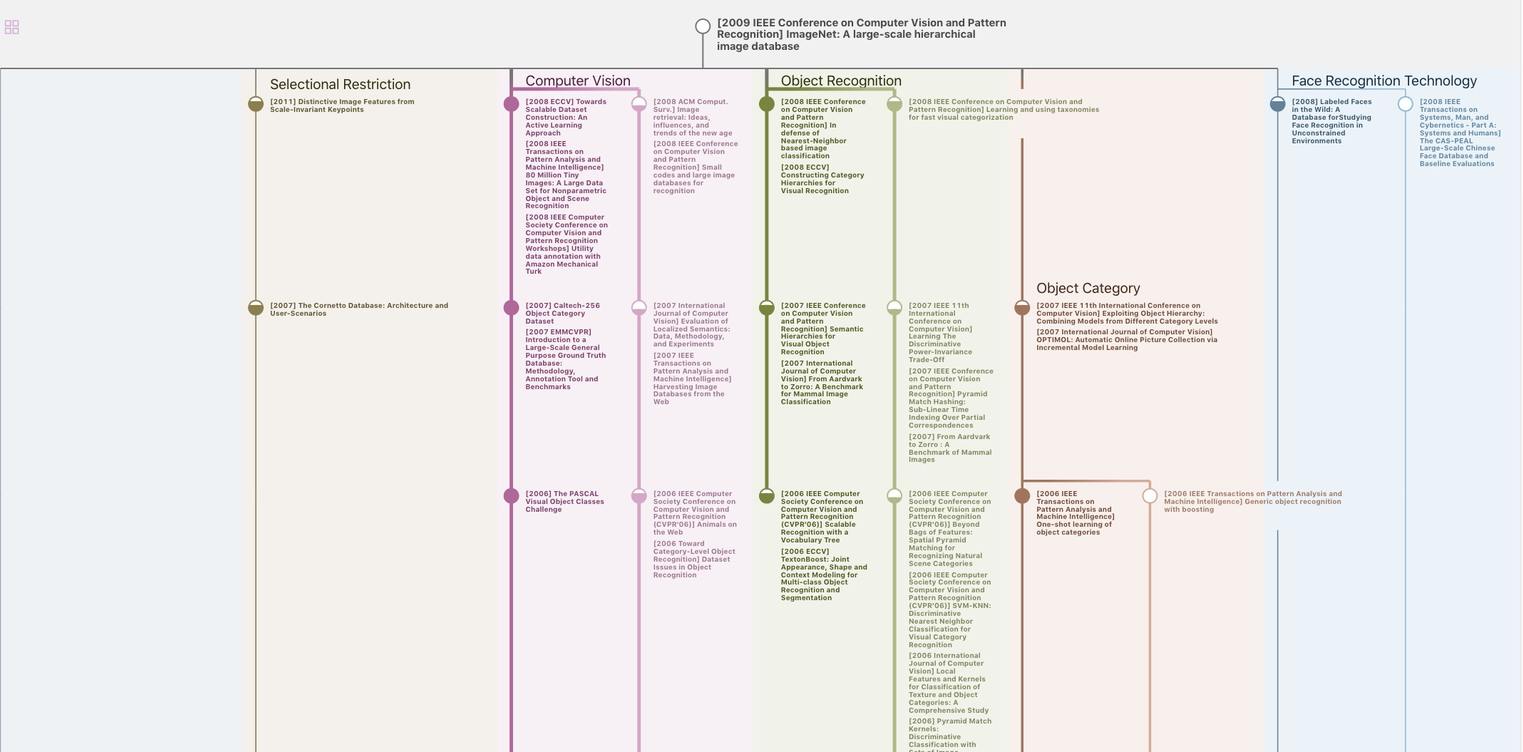
生成溯源树,研究论文发展脉络
Chat Paper
正在生成论文摘要