GSoANet: Group Second-Order Aggregation Network for Video Action Recognition
NEURAL PROCESSING LETTERS(2023)
摘要
In video action recognition, the existing methods mostly utilize global average pooling at the end of the network to aggregate spatio-temporal features of the video to generate global video representations, which are insufficient in modeling complex spatio-temporal feature distributions and capturing spatio-temporal dynamic information. To address the issue, we propose a novel group second-order aggregation network (GSoANet), the core of which is to integrate the group second-order aggregation module (GSoAM) at the end of the network to aggregate video spatio-temporal features. GSoAM first adopts the grouping strategy to decompose input features into a group of relatively low-dimensional vectors, and then aggregates video spatio-temporal features in the low-dimensional space. Then the subspaces represented by codewords are introduced, where in each subspace, differences between spatio-temporal features and codewords are aggregated with soft assignment refecting their proximity. Finally, the nonlinear geometric structure of the fused subspaces is modeled by using the iterative matrix square root normalized covariance. In addition, GSoANet also introduces a high-performance convolutional network ConvNeXt as a backbone to improve network accuracy at a lower computational cost. Extensive experimental results on four challenging video datasets demonstrate the effectiveness of the proposed method in aggregating spatio-temporal features as well as its competitive results.
更多查看译文
关键词
Video action recognition,Second-order pooling,Feature aggregation,Convolutional network
AI 理解论文
溯源树
样例
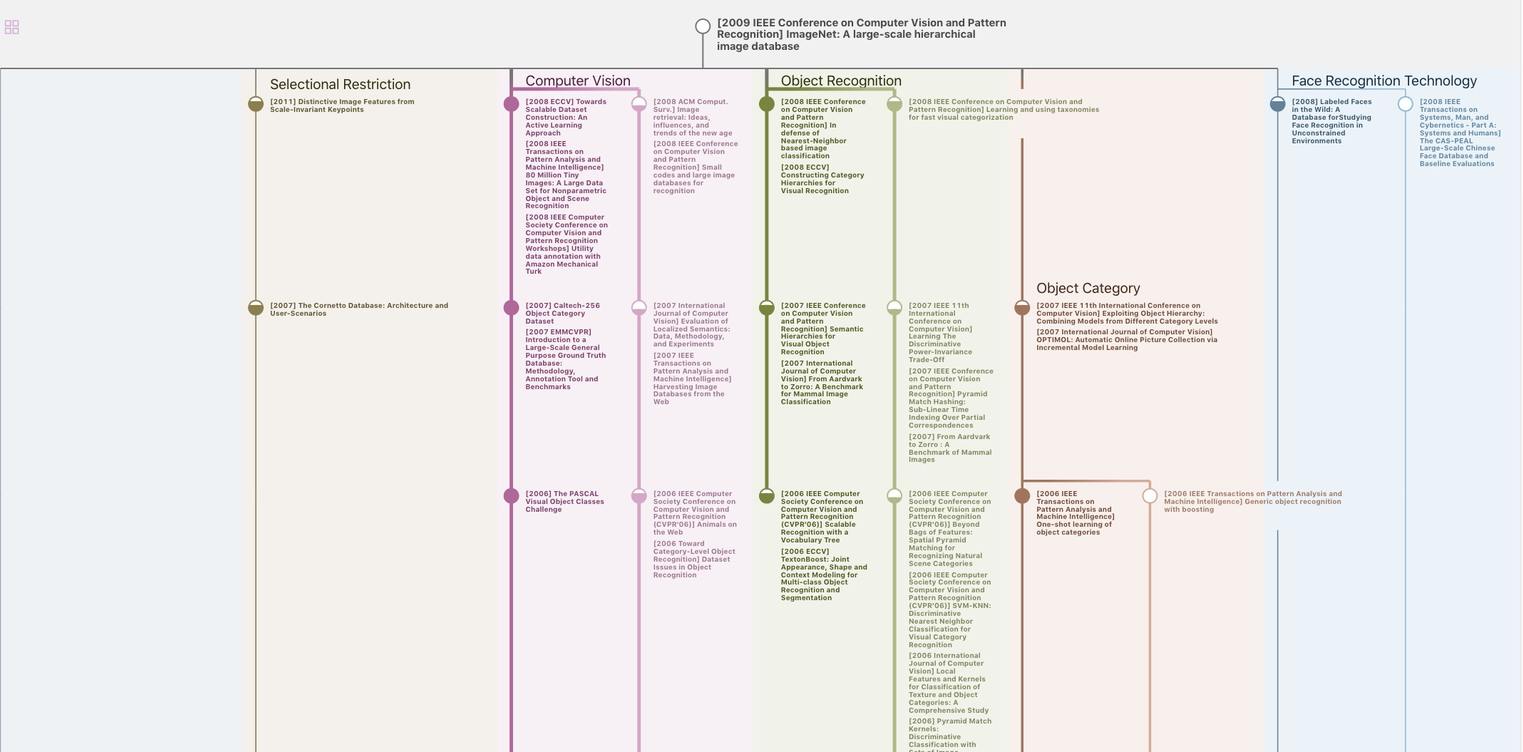
生成溯源树,研究论文发展脉络
Chat Paper
正在生成论文摘要