Discrete wavelet transform based branched deep hybrid network for environmental noise classification
COMPUTATIONAL INTELLIGENCE(2023)
摘要
With ever growing urbanization, the environmental noise is becoming hazardous. Vehicular traffic, locomotives, heavy machinery in industry, and construction processes are the major sources of noise pollution. It has adverse effects on the health of humans as well as that of the wild life. World Health Organization (WHO) puts noise pollution as the second major cause of illness due to environmental reasons. The effects of noise pollution on the quality of life are usually ignored. Due to this reason it is common, even in the first world countries, to have the WHO's peak noise standards violated in residential areas. Therefore, there is a need to have a real time, portable and easy to replicate, mechanism to monitor the noise sources. In this work, we propose a novel architecture of a deep neural network to classify a 10-class environmental noise data called URBANSOUND8K. This network is comprised of three components, (1) one dimensional two level Discrete Wavelet Transform (DWT) component, (2) branched component for feature extraction through auto-encoders, and (3) LSTM and fully-connected layers based classification component. With all components combined, we call this network DWTNet. By embedding the DWT component as a part of network, we eliminate the need of prior data conversion into spectral and/or spectro-temporal domains. The efficiency of DWTNet is comparable to the state of the art networks with significantly lower number of trainable parameters. We analyze the contribution of classification accuracy. We further study some of the classification results individually and show that some of the mis-classifications are actually multi-class classifications with distributed decision confidence.
更多查看译文
关键词
discrete wavelet transform,deep hybrid network,noise
AI 理解论文
溯源树
样例
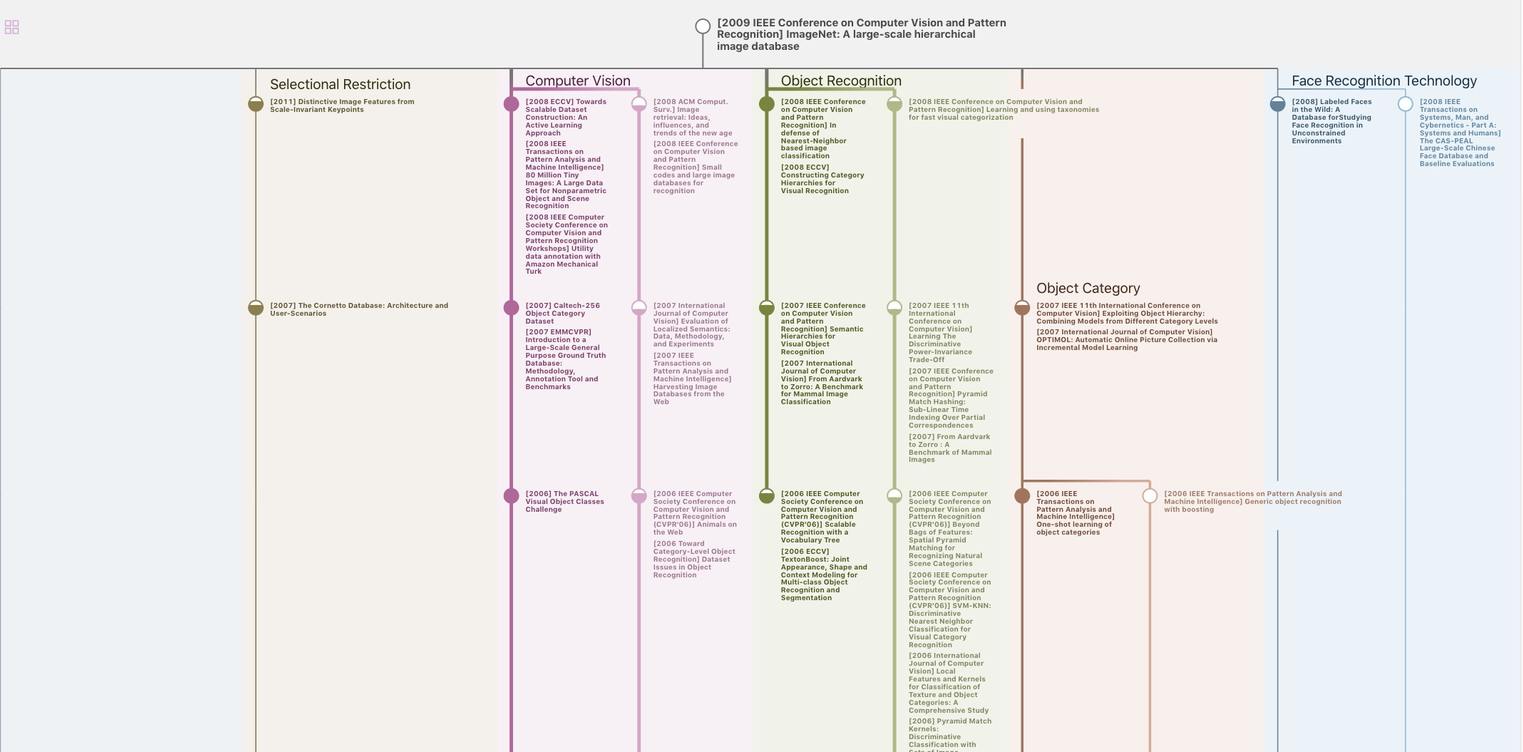
生成溯源树,研究论文发展脉络
Chat Paper
正在生成论文摘要