Accelerated Design of Architected Materials with Multifidelity Bayesian Optimization
Journal of engineering mechanics(2023)
摘要
In this work, we present a multifidelity Bayesian optimization framework for designing architected materials with optimal energy absorption during compression. Data from both physical experiments (high fidelity) and numerical simulations (low fidelity) are fed in parallel to train the surrogate model, which iteratively decides the next sets of experiments and simulations to run in order to find the optimal structural parameters. We show that having multifidelity data sources allows the optimization framework to find the optimum after fewer iterations relative to using a single high-fidelity source. This saves both material costs and time in the optimization process. Finally, we also apply constraints (on relative density and stress variations) to the optimization process, finding optimal structures within the bounds of the constraints. This framework can be translated to other problems that require complex, high-fidelity, labor-intensive experiments while automating low-fidelity simulations.
更多查看译文
AI 理解论文
溯源树
样例
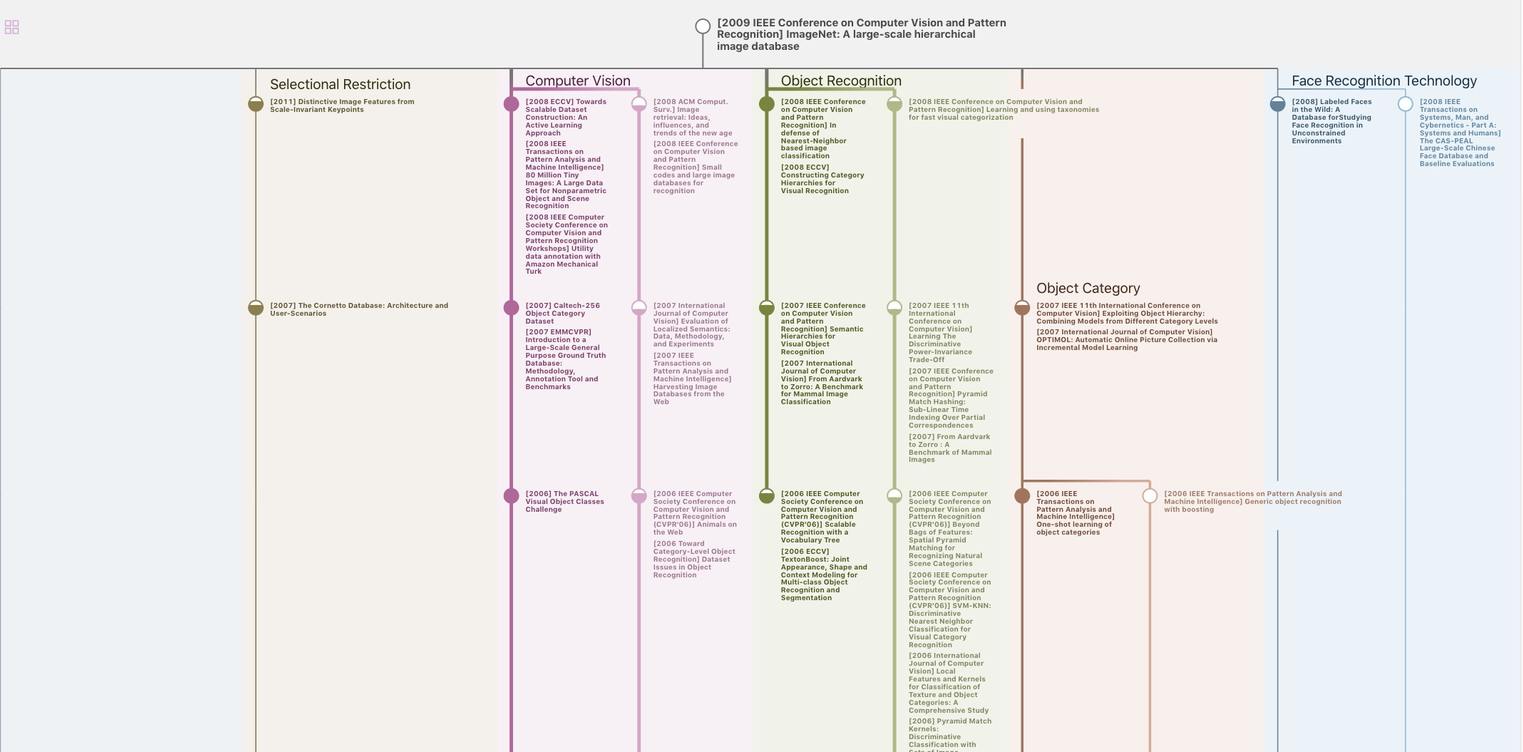
生成溯源树,研究论文发展脉络
Chat Paper
正在生成论文摘要