Low-rank approximation of Hankel matrices in denoising applications for statistical damage diagnosis of wind turbine blades
MECHANICAL SYSTEMS AND SIGNAL PROCESSING(2023)
摘要
Model order selection is a fundamental task in subspace identification for estimation of modal parameters, uncertainty propagation and damage diagnosis. However, the true model order and the related low-rank structure of the dynamic system are generally unknown. In this paper, a statistical methodology to actively select the dynamic signal subspace in covariance -driven subspace identification is developed on the basis of statistical analysis of the eigenvalue condition numbers of the output covariance Hankel matrix. It is shown that the condition numbers highly sensitive to random perturbations characterize the noise subspace. The signal subspace is separated from the noise subspace by analyzing two statistical parameters associated with the condition number sensitivity, whose thresholds are user-defined. A practical algorithm to retrieve the system dynamics is designed and demonstrated on a running example of a simulated wind turbine blade benchmark. The resultant framework is then applied in the context of damage detection on a medium-size wind turbine blade. It is demonstrated that the detectability of small damage is enhanced compared to the classic approaches and robustness of damage diagnosis is increased by reducing the number of false alarms.
更多查看译文
关键词
hankel matrices,wind turbine blades,statistical damage diagnosis,low-rank
AI 理解论文
溯源树
样例
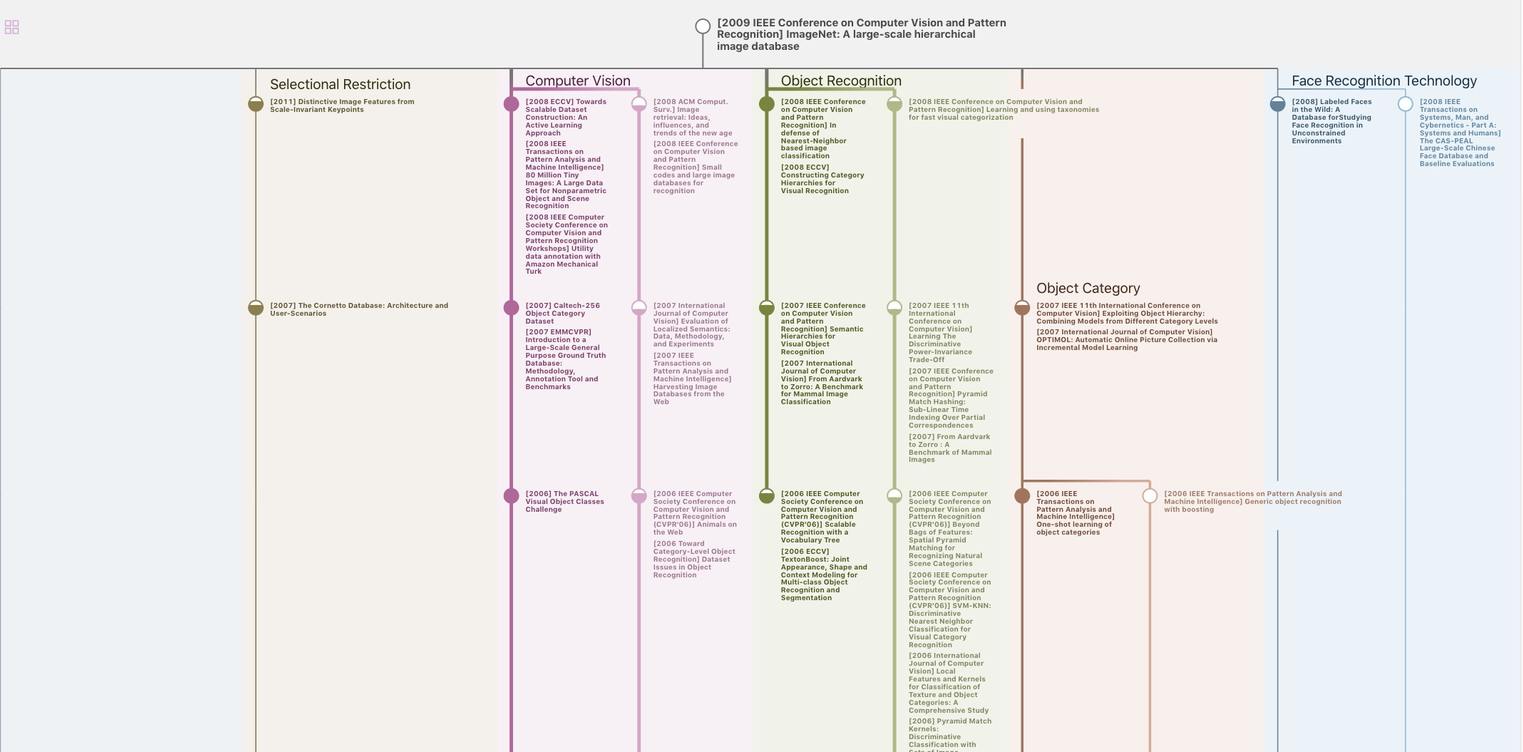
生成溯源树,研究论文发展脉络
Chat Paper
正在生成论文摘要