A novel lightweight multi-dimension feature fusion network for single-image super-resolution reconstruction
VISUAL COMPUTER(2024)
摘要
In recent years, due to the powerful feature extraction capabilities of convolutional neural networks (CNNs), many single-image super-resolution (SISR) methods based on CNN have achieved remarkable results. However, more model parameters and higher computational cost make these methods unsuitable for devices with limited computing power. In this paper, a lightweight multi-dimension feature fusion network (LMDFFN) is proposed to reduce model parameters. In nonlinear mapping module of the LMDFFN, the lightweight feature extraction block (LFEB) and the multi-dimensional feature extraction block (MDFEB) embedded in each LFEB are designed to significantly improve the reconstruction performance. The channel split operation can group the features. Combined with square-kernel convolution kernel and asymmetric convolution kernel, different types of convolution kernels can focus on different types of features, and the grouping mechanism can effectively reduce the complexity of the model. In addition, a channel and spatial attention module (CSAM) dedicated to SISR is proposed to focus on image details and different semantic level in feature maps. Moreover, a lightweight reconstruction module is designed with depth-wise separable convolution, and the designed spatial attention module is integrated into the reconstruction module to focus on more important features in the reconstruction stage. Compared with recent lightweight SISR methods, LMDFFN has better reconstruction accuracy with lower model parameters and computational complexity, and the reconstructed images have higher visual quality.
更多查看译文
关键词
Multi-dimension feature fusion,Single-image super-resolution reconstruction,Channel and spatial attention module
AI 理解论文
溯源树
样例
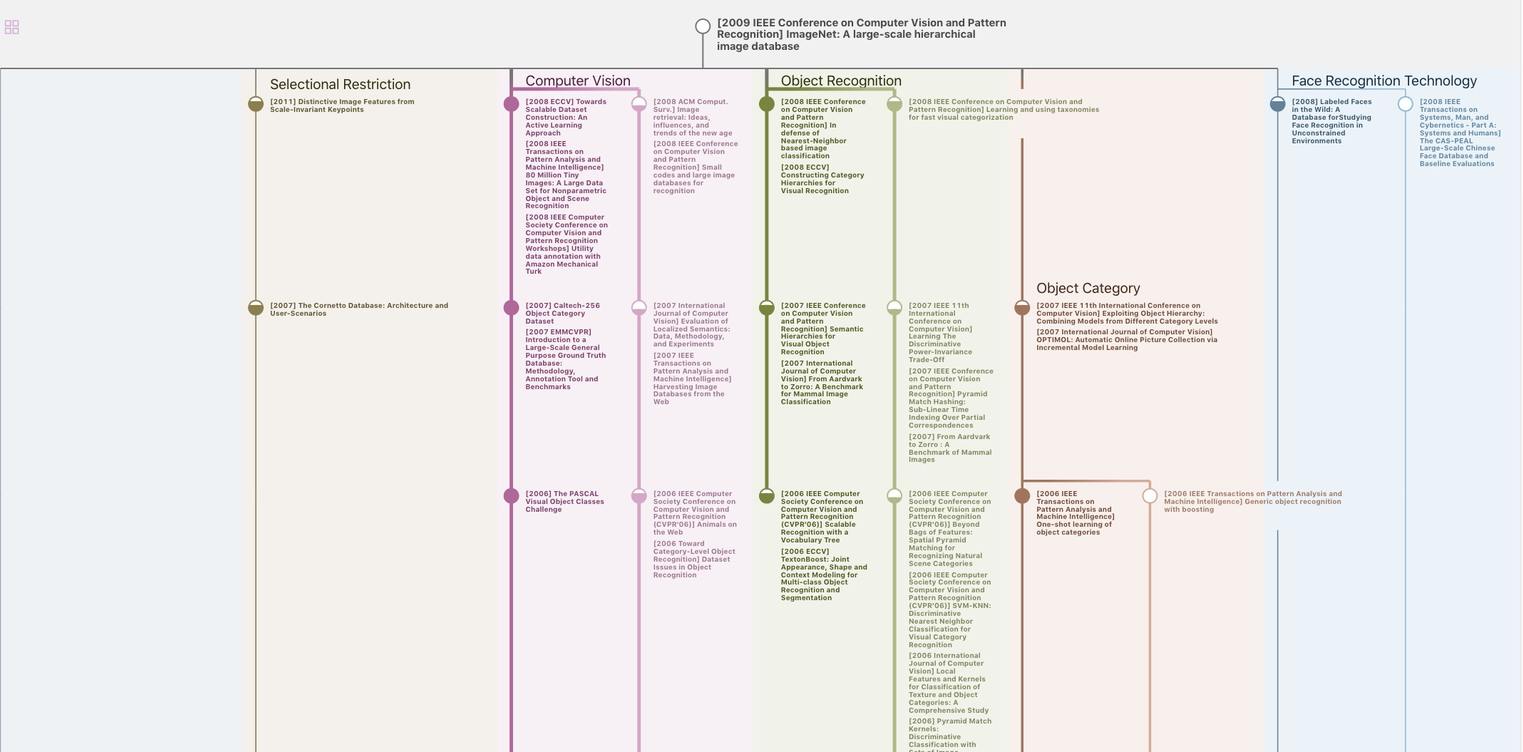
生成溯源树,研究论文发展脉络
Chat Paper
正在生成论文摘要