Data-driven State of Health Monitoring for Maritime Battery Systems – a Case Study on Sensor Data from Ships in Operation
Ships and offshore structures(2023)
摘要
IMO is implementing a stricter GHG strategy to reduce emissions from shipping. Battery powered ships, whether hybrid or fully electric, are a flexible solution with existing marine systems that can lower fuel consumption and emissions. However, relying on battery power for propulsion and maneuvering introduces new risks related to the available energy, which can be controlled by monitoring the SOH and SOC states. This paper conducts a detailed case study on applying Battery AI to operational data from real battery systems onboard ships, introducing state-of-the-art data-driven modelling and estimation of battery SOH, outlining the Battery AI, and providing corresponding recommendations to address issues detected during the test implementation. The performance of Battery AI is evaluated by comparing its results to annual SOH test results, with a maximum deviation underestimated by 3.21%. The paper concludes with a discussion of the results and recommendations for utilizing this method in risk assessment.
更多查看译文
关键词
Green shipping,battery,bigdata,machine learning
AI 理解论文
溯源树
样例
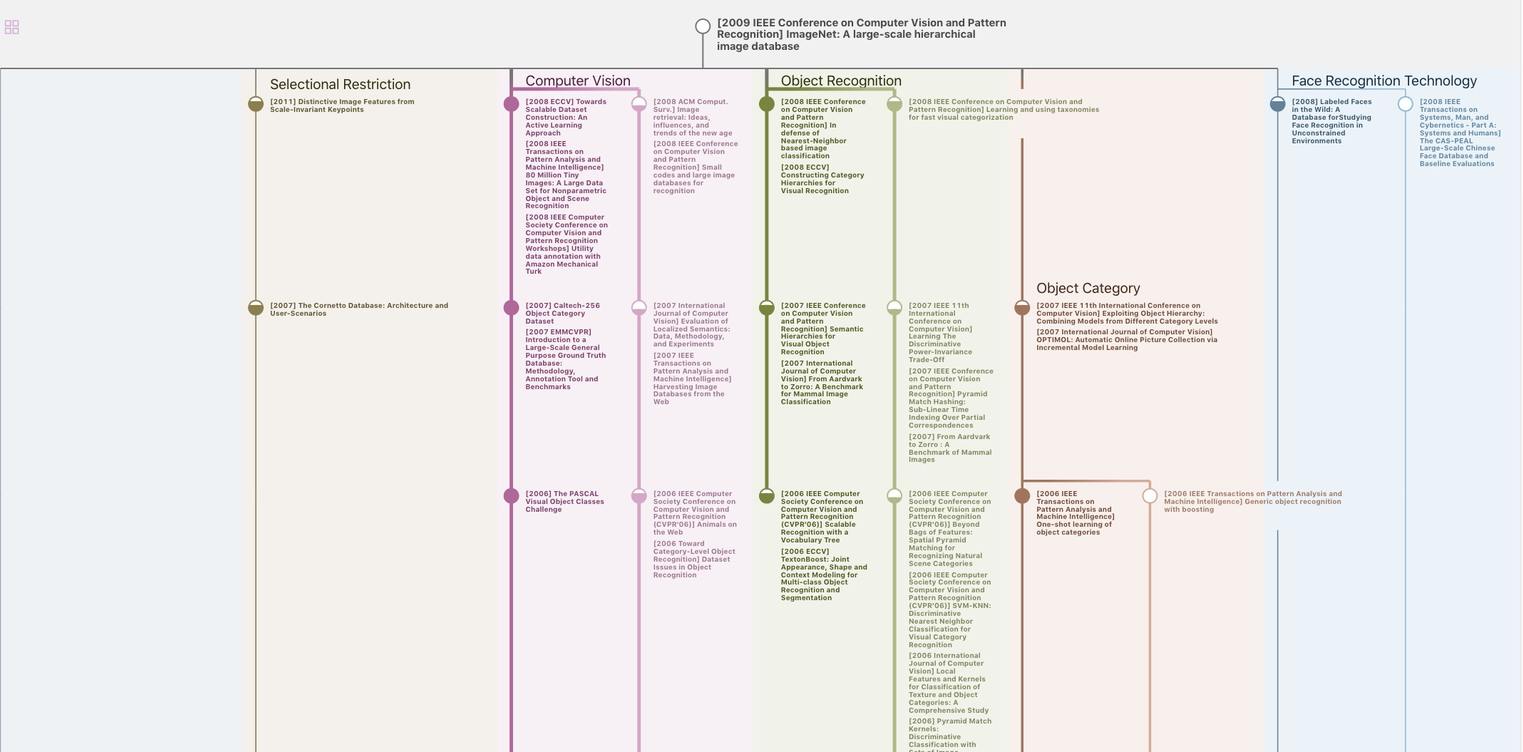
生成溯源树,研究论文发展脉络
Chat Paper
正在生成论文摘要