Exploratory Factor Analysis Trees: Evaluating Measurement Invariance Between Multiple Covariates
STRUCTURAL EQUATION MODELING-A MULTIDISCIPLINARY JOURNAL(2023)
摘要
Measurement invariance (MI) describes the equivalence of a construct across groups. To be able to meaningfully compare latent factor means between groups, it is crucial to establish MI. Although methods exist that test for MI, these methods do not perform well when many groups have to be compared or when there are no hypotheses about them. We suggest a method called Exploratory Factor Analysis Trees (EFA trees) that are an extension to SEM trees. EFA trees combine EFA with a recursive partitioning algorithm that can uncover non-invariant subgroups in a data-driven manner. An EFA is estimated and then tested for parameter instability on multiple covariates (e.g., age, education, etc.) by a decision tree based method. Our goal is to provide a method with which MI can be addressed in the earliest stages of questionnaire development or prior to analyses between groups. We show how EFA trees can be implemented in the software R using lavaan and partykit. In a simulation, we demonstrate the ability of EFA trees to detect a lack of MI under various conditions. Our online material contains a template script that can be used to apply EFA trees on one's own questionnaire data. Limitations and future research ideas are discussed.
更多查看译文
关键词
factor,measurement invariance,trees
AI 理解论文
溯源树
样例
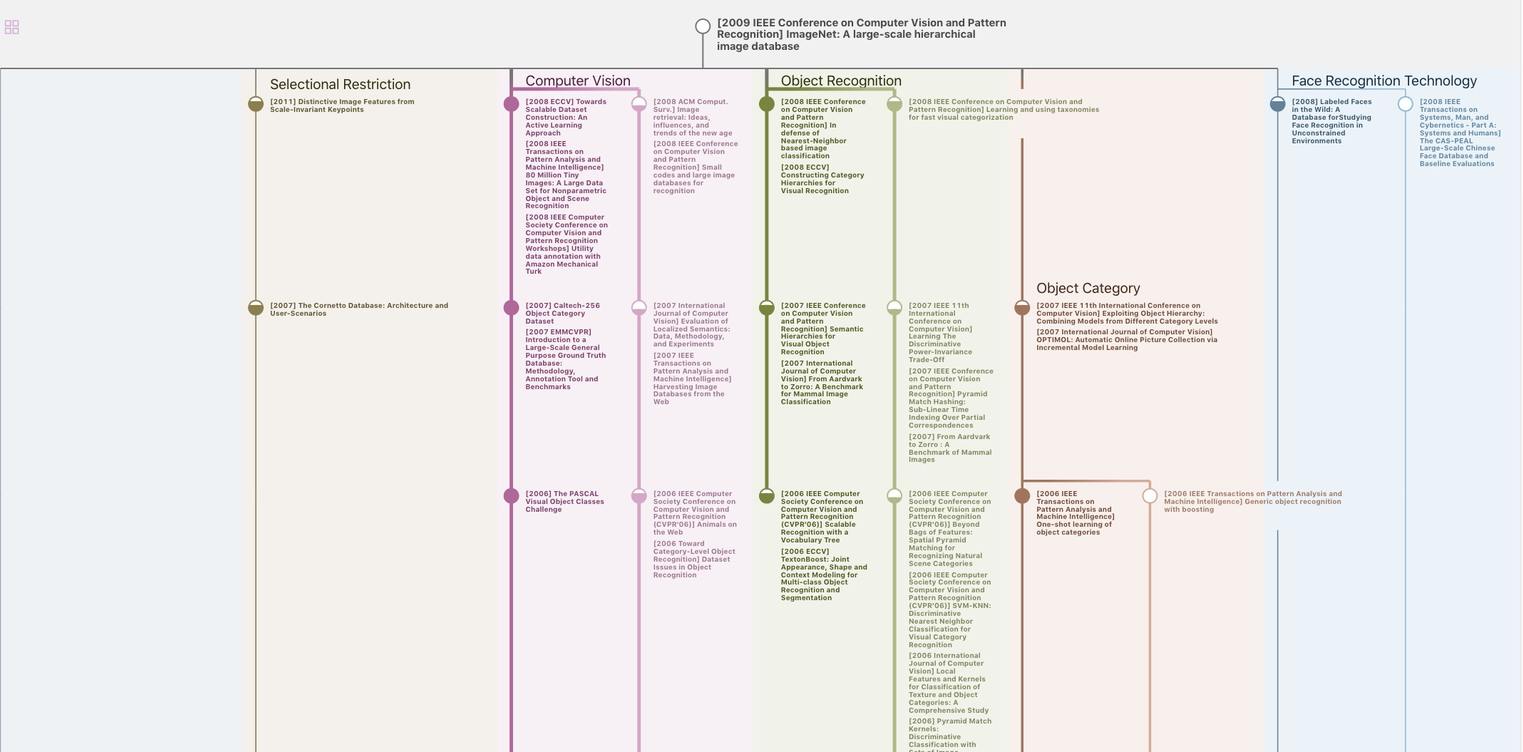
生成溯源树,研究论文发展脉络
Chat Paper
正在生成论文摘要