Multi-channel EEG-based BCI Using Regression and Classification Methods for Attention Training by Serious Game
Biomedical signal processing and control(2023)
摘要
Background and Objective: Most of the existing brain-computer interfaces (BCIs) for attention cognitive training provide feedback only according to the classifier's output, instead of biomarker of interest. This work aims to develop an electroencephalogram (EEG)-based BCI for sustained attention training, which monitors the user's attention level by acquiring his/her neural information from specific brain regions, and translates it into control commands for a game, enhancing the modulation of theta (O: 4-7 Hz) and beta (fi: 13-30 Hz) rhythms over the attention network.Methods: The proposed BCI filters short-time EEG periods in a frequency range from 1 to 30 Hz, to later calculate covariance matrices and immediately extract feature vectors onto the Riemannian geometry space. Then, the support vector machine with the radial basic function kernel is trained to classify the attention and non-attention states. As a novelty, the designed BCI provides visual feedback more correlated with the user's attention level and Olfi modulation, using a control strategy that combines the classifier's output and the output of a cubic regression model. This model is fitted by weighting the feature vectors by considering the Olfi power ratios, in order to translate the neural information into control commands for the serious game. Twenty healthy subjects were divided into three groups to test the proposed BCI with different control strategies. The collected data were also used to evaluate both the BCI performance and the effect of adding Olfi in the control model.Results: The BCI reached accuracy up to 98% recognizing both attention and non-attention states. The average Olfi distribution on the user's scalp showed reduction of this ratio in brain regions that play an important role during sustained attention tasks, such as frontal, temporal, parietal, and occipital lobes. This finding confirms that the control strategy considering Olfi from the frontotemporal cortex forced the modulation of this biomarker.Conclusions: Our approach and findings can contribute to the development of more effective EEG technologies for cognitive attention training.
更多查看译文
关键词
Attention training,Brain-computer interface,Cognition,Neurofeedback,Sustained attention
AI 理解论文
溯源树
样例
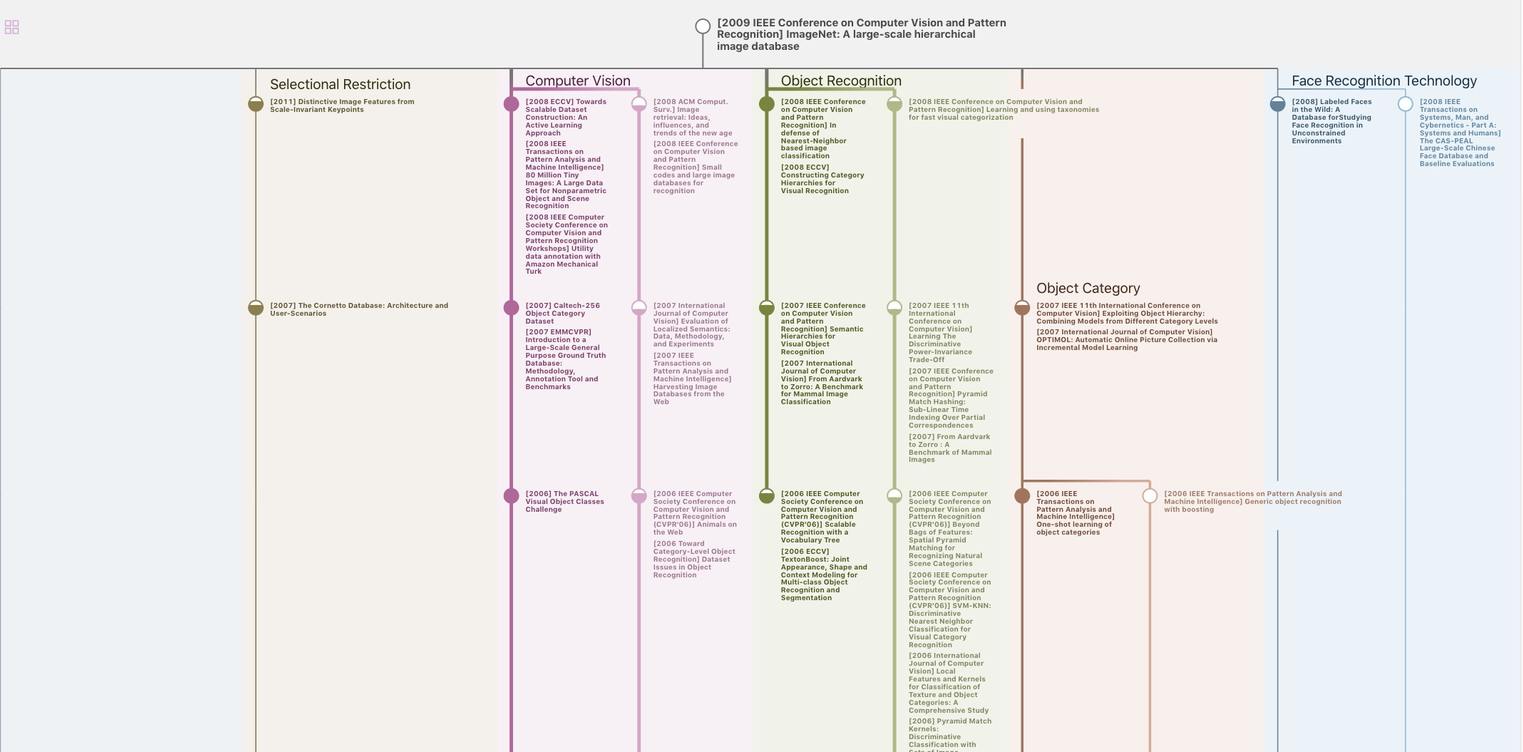
生成溯源树,研究论文发展脉络
Chat Paper
正在生成论文摘要