Sparse Bayesian learning with automatic-weighting Laplace priors for sparse signal recovery
COMPUTATIONAL STATISTICS(2023)
摘要
The least absolute shrinkage and selection operator (LASSO) and its variants are widely used for sparse signal recovery. However, the determination of the regularization factor requires cross-validation strategy, which may obtain a sub-optimal solution. Motivated by the self-regularization nature of sparse Bayesian learning (SBL) approach and the framework of generalized LASSO, we propose a new hierarchical Bayesian model using automatic-weighting Laplace priors in this paper. In the proposed hierarchical Bayesian model, the posterior distributions of all the parameters can be approximated using variational Bayesian inference, resulting in closed-form solutions for all parameters updating. Moreover, the space alternating variational estimation strategy is used to avoid matrix inversion, and a fast algorithm (SAVE-WLap-SBL) is proposed. Comparing to existed SBL methods, the proposed method encourages the sparsity of signals more efficiently. Numerical experiments on synthetic and real data illustrate the benefit of these advances.
更多查看译文
关键词
Sparse signal recovery,Sparse Bayesian leaning,Space alternative variational estimation
AI 理解论文
溯源树
样例
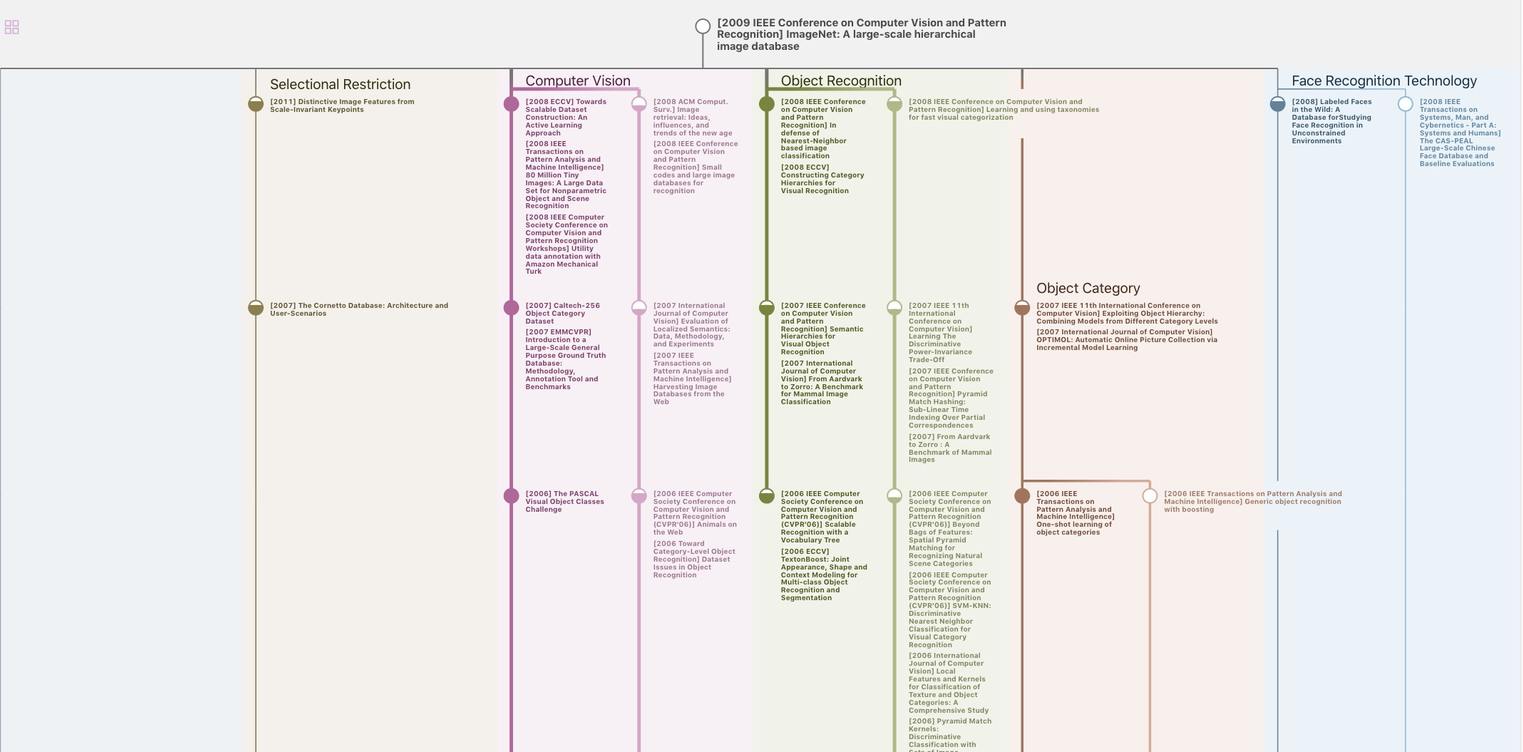
生成溯源树,研究论文发展脉络
Chat Paper
正在生成论文摘要