Deep learning-based pedestrian trajectory prediction and risk assessment at signalized intersections using trajectory data captured through roadside LiDAR
JOURNAL OF INTELLIGENT TRANSPORTATION SYSTEMS(2023)
摘要
In recent years, rapid advancements in the Autonomous Vehicles (AVs) industry have greatly motivated the research and development in pedestrian trajectory prediction and risk assessment. One of the critical requirements for AVs is to predict the future trajectories of pedestrians and provide collision warnings in an accurate and prompt manner. Nevertheless, accurate prediction of pedestrian trajectories remains a technical challenge, mainly caused by the heterogeneity of pedestrian crossing behavior and uncertainties in vehicle-pedestrian interactions. This paper proposes a deep learning-based method for pedestrian trajectory prediction and risk assessment, using trajectory data extracted from roadside LiDAR data and corresponding signal phasing information at MLK and Georgia Avenue in Chattanooga, TN. Meanwhile, a set of criteria referred to as the risk factor is established to quantitatively evaluate the risk of the pedestrian crossing behavior, which also serves as a learnable feature. A Long Short-Term Memory (LSTM) network is proposed, which takes the following data as the input: the pedestrian trajectory data, signal phasing data, and risk factors from the past 10 steps. Meanwhile, the network predicts the pedestrian trajectory and risk factor at the future time step. In the experimental study, the root-mean-square errors between the predicted and ground truth x and y coordinates are 0.225 meters and 0.377 meters, respectively, and the F1 score value for the risk factor is 99.6%, demonstrating the efficacy of the proposed LSTM-based methodology on pedestrian trajectory prediction and risk assessment.
更多查看译文
关键词
Deep learning,Long Short-Term Memory (LSTM) network,pedestrian trajectory prediction,risk assessment,roadside LiDAR data,vulnerable road user (VRU)
AI 理解论文
溯源树
样例
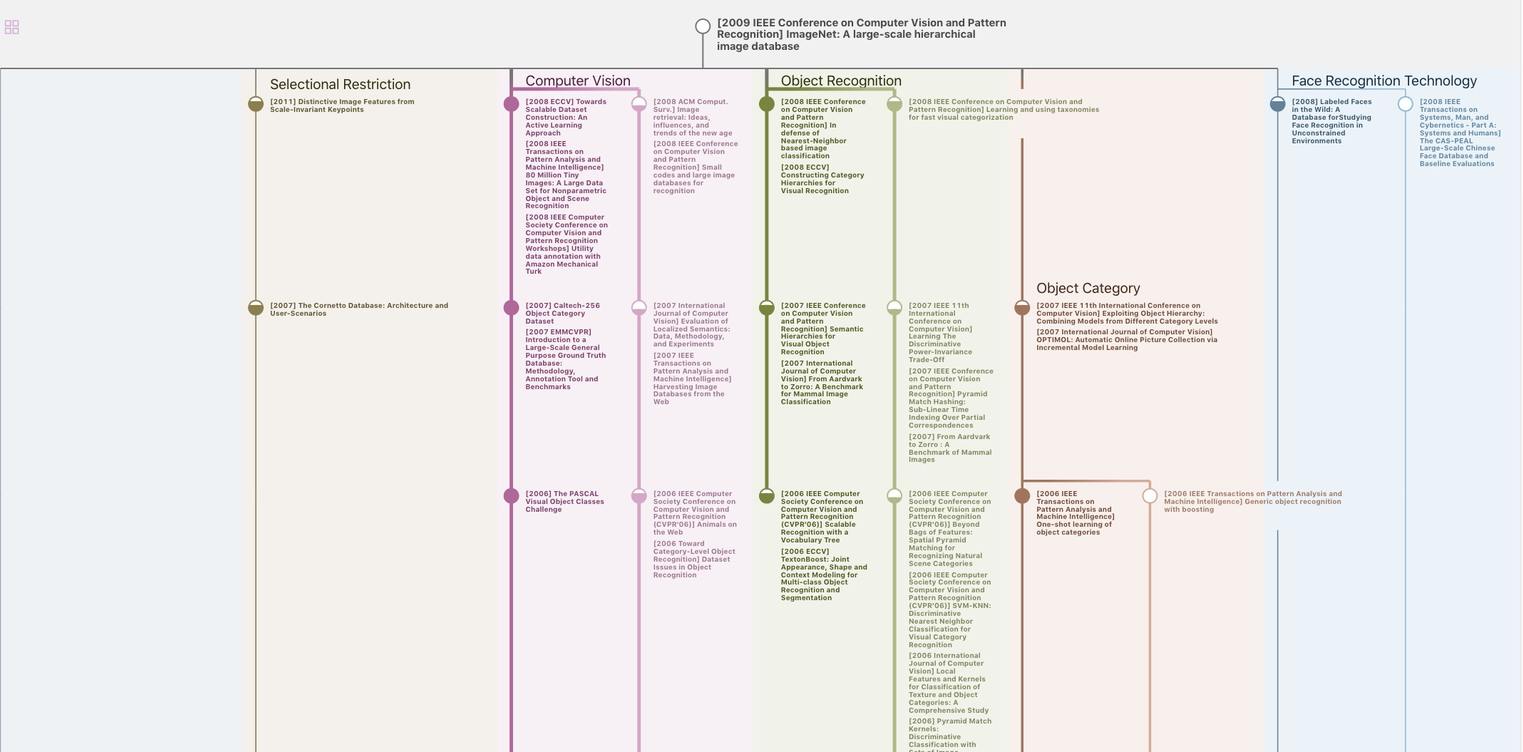
生成溯源树,研究论文发展脉络
Chat Paper
正在生成论文摘要