Analog ensemble data assimilation in a quasigeostrophic coupled model
QUARTERLY JOURNAL OF THE ROYAL METEOROLOGICAL SOCIETY(2023)
摘要
The ensemble forecast dominates the computational cost of many data assimilation methods, especially for high-resolution and coupled models. In situations where the cost is prohibitive, one can either use a lower-cost model or a lower-cost data assimilation method, or both. Ensemble optimal interpolation (EnOI) is a classical example of a lower-cost ensemble data assimilation method that replaces the ensemble forecast with a single forecast and then constructs an ensemble about this single forecast by adding perturbations drawn from climatology. This research develops lower-cost ensemble data assimilation methods that add perturbations to a single forecast, where the perturbations are obtained from analogs of the single model forecast. These analogs can either be found from a catalog of model states, constructed using linear combinations of model states from a catalog, or constructed using generative machine-learning methods. Four analog ensemble data assimilation methods, including two new ones, are compared with EnOI in the context of a coupled model of intermediate complexity: Q-GCM. Depending on the method and on the physical variable, analog methods can be up to 40% more accurate than EnOI.
更多查看译文
关键词
ensemble data assimilation
AI 理解论文
溯源树
样例
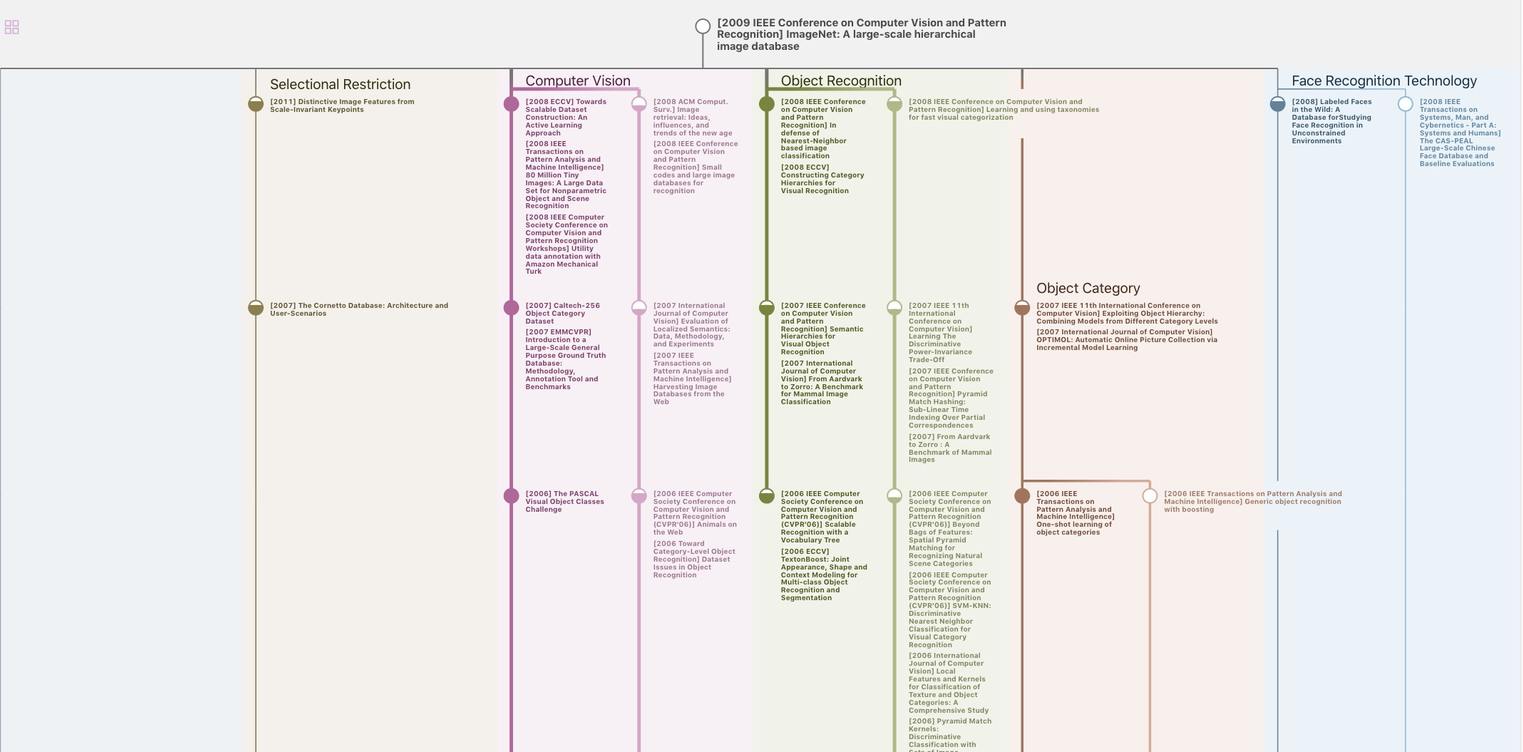
生成溯源树,研究论文发展脉络
Chat Paper
正在生成论文摘要