Dimension reduction method of high-dimensional fault datasets based on C_M_t-SNE under unsupervised background
MEASUREMENT(2023)
摘要
The unlabeled fault datasets often contain much non-sensitive redundant, and uncertain information. This study designs a novel interpretable and unsupervised dimension reduction method for unlabeled data containing redundancy and uncertainty. Firstly, a fuzzy-based way for pseudo-label generation is given, and feature cloud models under pseudo labels are established; Secondly, this study takes the expectation, entropy, and hyper entropy of the cloud models representing uncertainty in features as spatial vectors. The difference degree between vectors is treated as the evaluation standard to filter out non-sensitive features based on the maximum initial difference; Moreover, redundant elements are fused by t-SNE, and lower dimensional feature components conducive for fault classification are obtained; Finally, the effectiveness of the method is demonstrated by comparative experiments. The results show that this method has a higher factor, which means that the method can better mine the difference among different faults and improve the performance of fault identification.
更多查看译文
关键词
Dimension reduction, Cloud model, Unsupervised fault diagnosis, Mechanical equipment
AI 理解论文
溯源树
样例
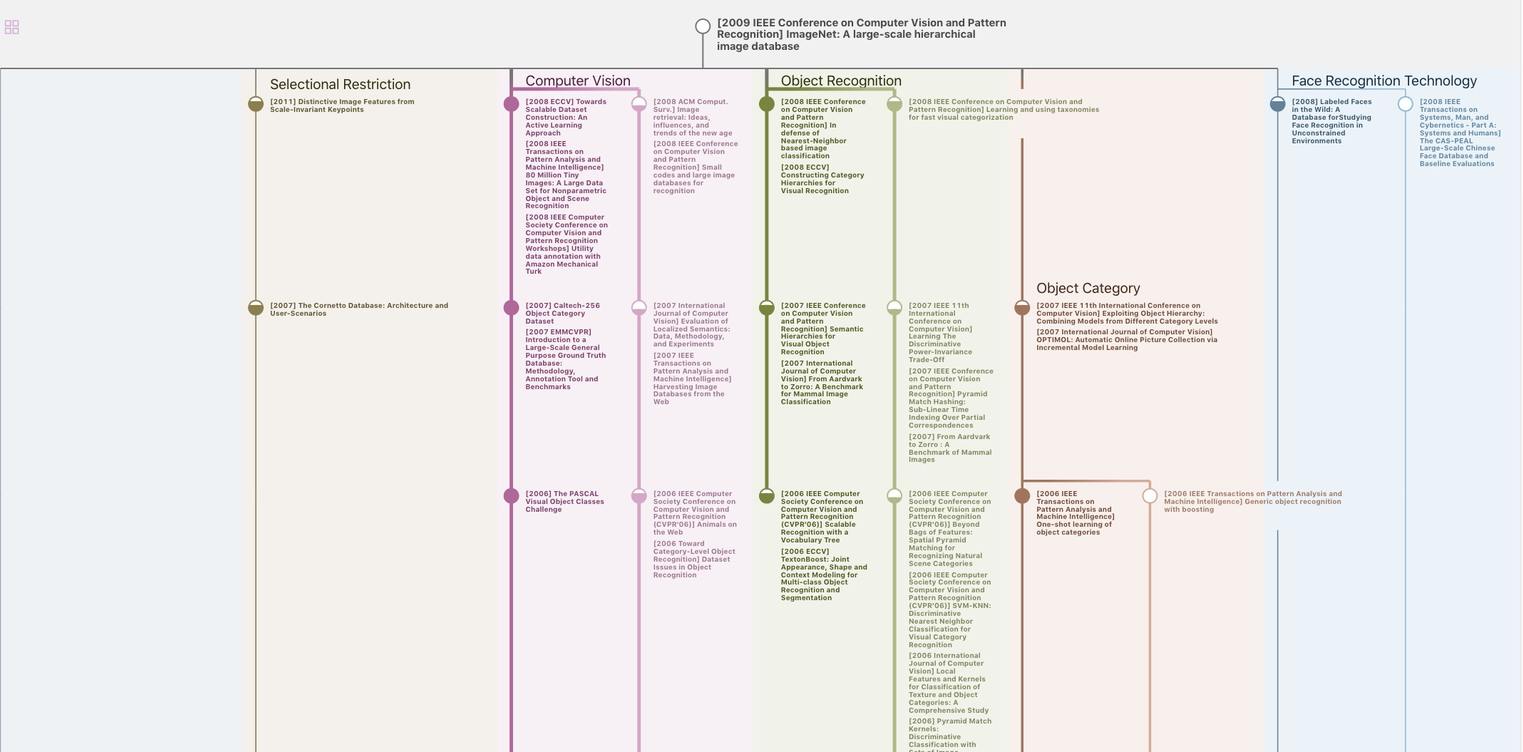
生成溯源树,研究论文发展脉络
Chat Paper
正在生成论文摘要