How Do Unobserved Confounding Mediators and Measurement Error Impact Estimated Mediation Effects and Corresponding Statistical Inferences? Introducing the R Package ConMed for Sensitivity Analysis
PSYCHOLOGICAL METHODS(2023)
摘要
Empirical studies often demonstrate multiple causal mechanisms potentially involving simultaneous or causally related mediators. However, researchers often use simple mediation models to understand the processes because they do not or cannot measure other theoretically relevant mediators. In such cases, another potentially relevant but unobserved mediator potentially confounds the observed mediator, thereby biasing the estimated direct and indirect effects associated with the observed mediator and threatening corresponding inferences. Additionally, researchers may not know the extent to which their measures are reliable, and accordingly, measurement error may bias estimated effects and mislead statistical inferences. Given these threats, we explore how the omission of an unobserved mediator and/or using variables with measurement error biases estimates and affects inferences associated with the observed mediator. Then, building off Frank's impact threshold for a confounding variable (ITCV), we propose a correlation-based sensitivity analysis. Lastly, we provide an R package ConMed to assess the robustness of mediation inferences given the omission of an unobserved, confounding mediator and/or measurement error.Translational Abstract Researchers across fields rely on mediation analyses to understand processes between the intervention and the outcome variable. Empirical studies regularly demonstrate the existence of multiple mediation pathways, such as through simultaneous or related mediators. However, many mediation analyses only include one mediator for various reasons and there are likely additional relevant mediators that could significantly bias estimates and inferences associated with the observed mediator. At the same time, valid statistical inferences also rely on the assumption that all the variables are measured without error, while researchers may not know the extent to which their measures are reliable. In practice, it is very likely that the omission of another confounding mediator and measurement error co-occurs. However, the extant literature on the combined effect of omitted confounders and measurement error does not evaluate cases where the confounder is a mediator; they focus on pretreatment confounders where the treatment has no impact on the omitted confounder. Therefore, we fill in the research gap by examining if/how omitting an alternative mediator and/or using variables with measurement error biases estimates and affects inferences associated with the observed mediator. We present analytical results as well as an illustrative example to demonstrate the potential consequences of omitting confounding mediator(s) given different reliability levels of observed variables. Additionally, we propose a correlation-based sensitivity analysis and provide an R package to help researchers assess the robustness of their mediation inferences given the omission of an unobserved, confounding mediator and/or measurement error.
更多查看译文
关键词
mediation model,posttreatment confounder,unobserved mediator,measurement error,sensitivity analysis,R package
AI 理解论文
溯源树
样例
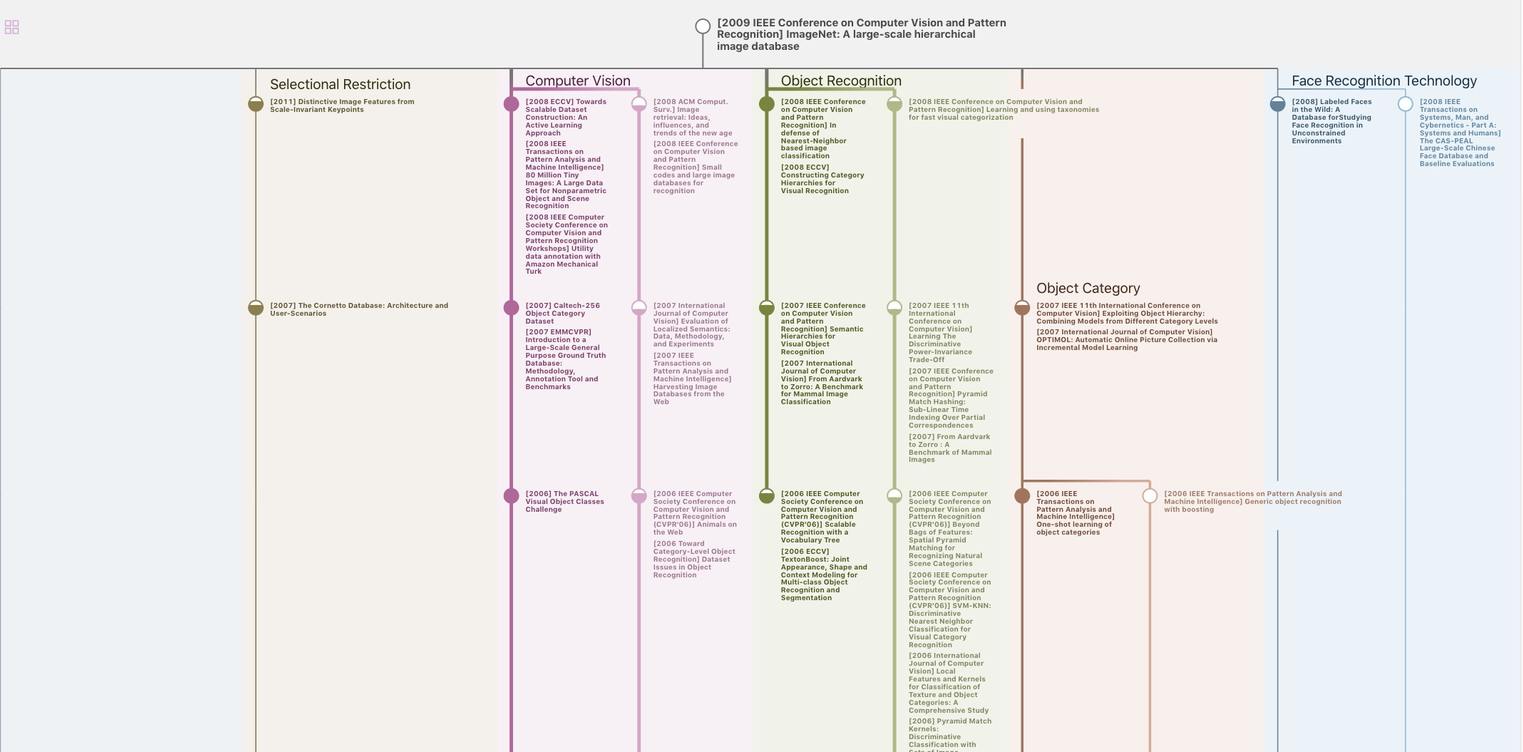
生成溯源树,研究论文发展脉络
Chat Paper
正在生成论文摘要