Quadratic upper bound algorithms for estimation under Cox model in case-cohort studies
STATISTICS AND ITS INTERFACE(2023)
摘要
A case-cohort design is a cost-effective biased-sampling scheme in large cohort studies. Implementation of parame-ter estimators for case-cohort data requires numerical ap-proaches. Using the minorization-maximization principle, which is a versatile tool for constructing optimization algo-rithms, we develop two quadratic-upper-bound algorithms for estimations in the Cox model under case-cohort design. The proposed algorithms are monotonic and reliably con-verge to the weighted estimators considered. These algo-rithms involve the inversion of the derived upper-bound ma-trix only one time in the whole process, and the upper-bound matrix is independent of both parameter and weight func-tions. These features make the proposed algorithms have simple update and low per-iterative cost, especially to large -dimensional problems. We conduct simulation studies and real data examples to illustrate the performance of the pro-posed algorithms, and compare them to Newton's method.
更多查看译文
关键词
cox model,estimation,case-cohort
AI 理解论文
溯源树
样例
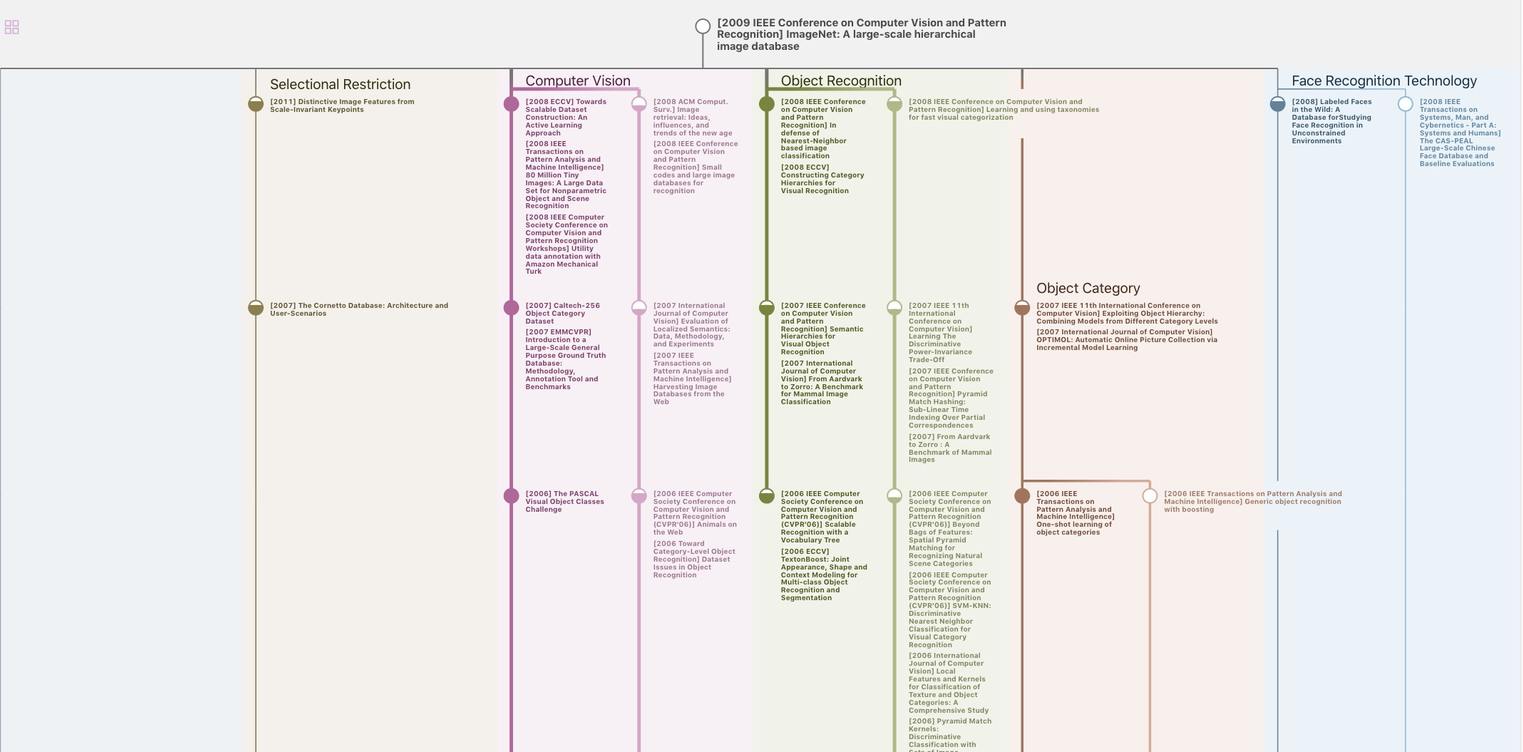
生成溯源树,研究论文发展脉络
Chat Paper
正在生成论文摘要