Confidence in the treatment decision for an individual patient: strategies for sequential assessment
STATISTICS AND ITS INTERFACE(2023)
摘要
Evolving medical technologies have motivated the devel-opment of treatment decision rules (TDRs) that incorporate complex, costly data (e.g., imaging). In clinical practice, we aim for TDRs to be valuable by reducing unnecessary test-ing while still identifying the best possible treatment for a patient. Regardless of how well any TDR performs in the target population, there is an associated degree of uncer-tainty about its optimality for a specific patient. In this paper, we aim to quantify, via a confidence measure, the uncertainty in a TDR as patient data from sequential pro-cedures accumulate in real-time. We first propose estimat-ing confidence using the distance of a patient's vector of covariates to a treatment decision boundary, with further distances corresponding to higher certainty. We further pro-pose measuring confidence through the conditional probabil-ities of ultimately (with all possible information available) being assigned a particular treatment, given that the same treatment is assigned with the patient's currently available data or given the treatment recommendation made using only the currently available patient data. As patient data accumulate, the treatment decision is updated and confi-dence reassessed until a sufficiently high confidence level is achieved. We present results from simulation studies and illustrate the methods using a motivating example from a depression clinical trial. Recommendations for practical use of the measures are proposed.
更多查看译文
关键词
sequential assessment,treatment decision,confidence,individual patient
AI 理解论文
溯源树
样例
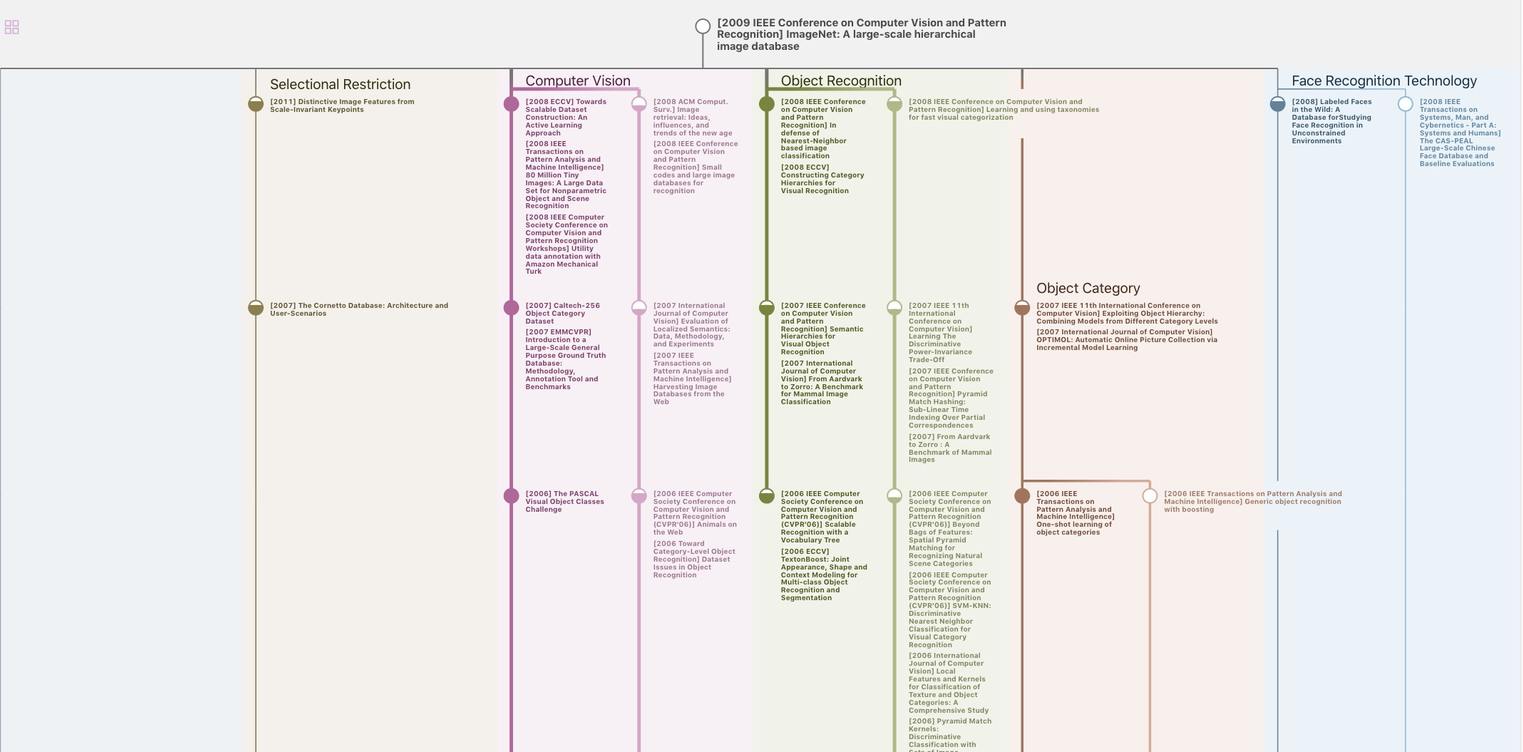
生成溯源树,研究论文发展脉络
Chat Paper
正在生成论文摘要