Reinforcement-Learning-Based Local Search Approach to Integrated Order Batching: Driving Growth for Logistics and Retail
IEEE ROBOTICS & AUTOMATION MAGAZINE(2023)
摘要
As an important part of Industry 4.0, a smart warehouse can offer smart tips and operational constraints for users. Improving its work efficiency is a promising growth driver for logistics companies and retailers. Therefore, a reinforcement-learning-based adaptive iterated local search (RAILS) approach is proposed to improve order-picking efficiency for a smart warehouse. A batching algorithm is proposed to deal with fluctuating orders efficiently and quickly obtain a high-quality initial solution. It can speed up the search for near-optimal solutions by extracting and using the features of the orders. Then, a perturbation mechanism is designed based on reinforcement learning that can adaptively select the perturbation type and determine the perturbation strength instead of a random way. Experimental results demonstrate that the proposed approach outperforms several existing ones, and its superiority becomes more significant as problems scale up.
更多查看译文
关键词
Rails, Perturbation methods, Heuristic algorithms, Feature extraction, Schedules, Mathematical models, Search problems
AI 理解论文
溯源树
样例
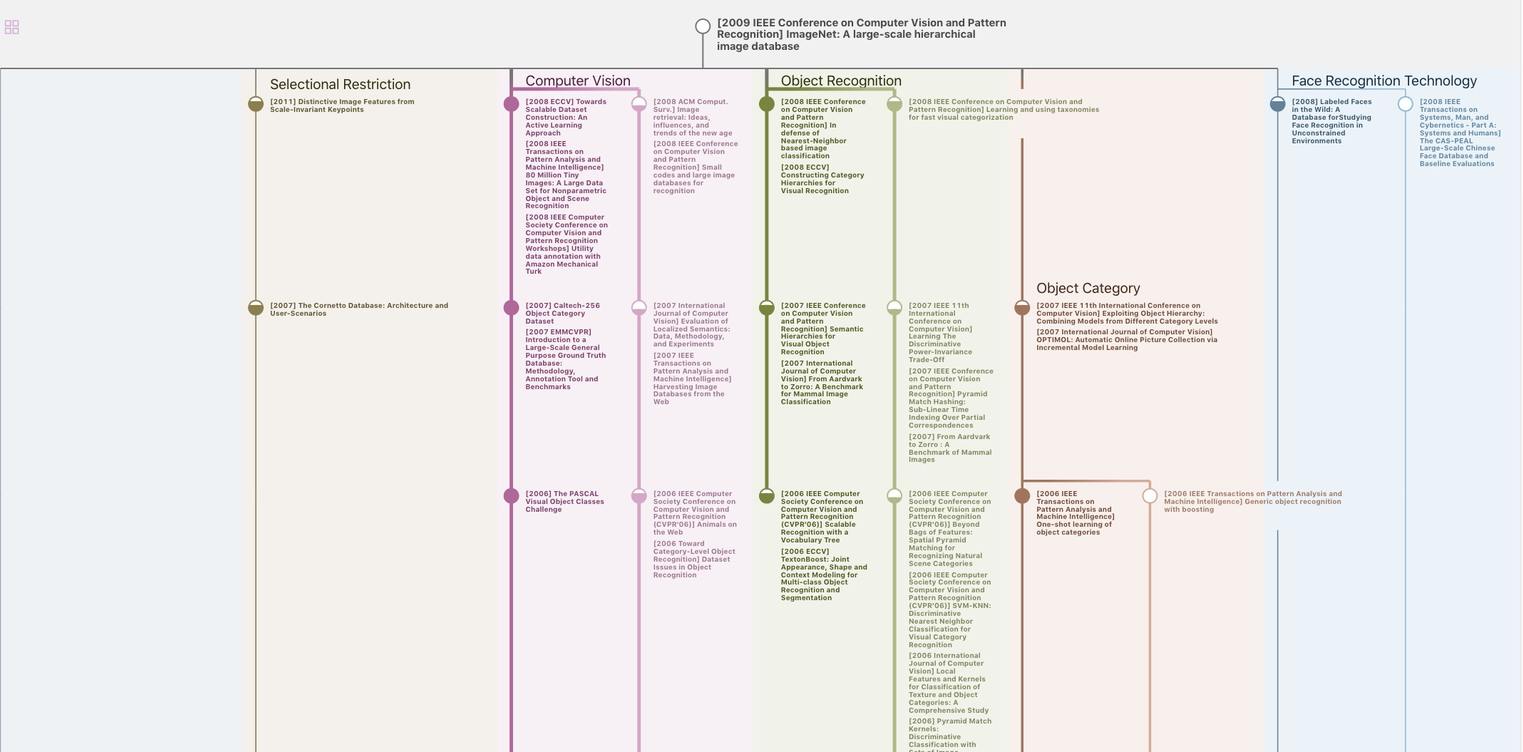
生成溯源树,研究论文发展脉络
Chat Paper
正在生成论文摘要