A methodology for transformer fault diagnosis based on the feature extraction from DGA data
INTERNATIONAL JOURNAL OF APPLIED ELECTROMAGNETICS AND MECHANICS(2023)
摘要
A methodology to diagnose transformer faults based on dissolved gas analysis (DGA) is proposed. Since a fault is more sensitive to the ratios of gas contents, a general ratio feature extraction framework is proposed to generate a feature subset as the input of the diagnosis model. The feature subset is evaluated by the improved case-based reasoning (CBR) and optimized by using a proposed new algorithm called the k-optimal algorithm (k-OA). The comparison results between the k-OA and the genetic algorithm (GA) show that the k-OA is more efficient in solving such a combinatorial optimization problem. The obtained optimal feature subset is used to diagnose a public transformer fault dataset, and a 92.6% diagnosis accuracy is observed as compared to that of only 85.1% diagnosis accuracy by using the original features.
更多查看译文
关键词
Case-based reasoning, combinatorial optimization, fault diagnosis, feature extraction, transformer
AI 理解论文
溯源树
样例
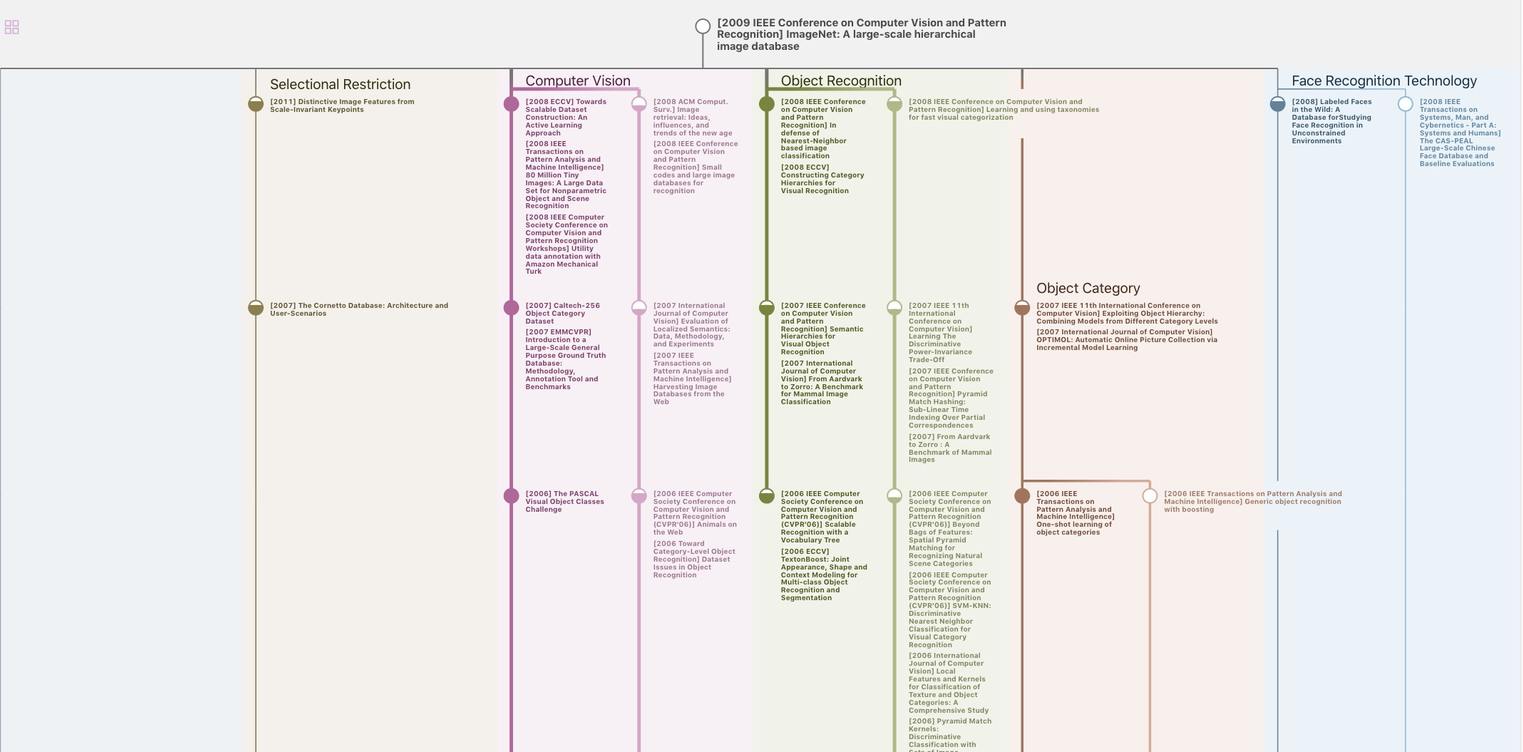
生成溯源树,研究论文发展脉络
Chat Paper
正在生成论文摘要