Weighted Vector Visibility based Graph Signal Processing (WVV-GSP) for Neural Decoding of Motor Imagery EEG signals
2022 IEEE 19TH INDIA COUNCIL INTERNATIONAL CONFERENCE, INDICON(2022)
摘要
The paper deals with weighted vector visibility-based graph signal processing (WVV-GSP) to decode motor imagery tasks from multi-channel EEG signals, which plays a key role in developing brain-computer interface (BCI) systems. Initially, multichannel EEG data at each time state is mapped into a vector which is defined as a node of the graph. Functional connectivity between different temporal states is determined by the visibility between the vector's norm and the norm of its projections onto subsequent time states. To create a weighted vector visibility graph using the connections among the nodes, it presents edge weights between the nodes using Gaussian kernels. The MI task-based WVV graph is then used to obtain GSP-based spectral features of Laplacian energy (LE) and Fiedler vector energy (FE). On a publicly accessible Physio-net database, the performance of the suggested technique is evaluated with a subject-specific and subject-independent basis using an SVM classifier. Average subject-specific accuracy of 98.99% with AUC of 99.8% and subject independent accuracy of 95.8% with AUC 96.4% is achieved for decoding left and right hand MI tasks. Extensive comparative analysis with the current literature exhibits the efficacy of the proposed WVV-GSP-based method for the neural decoding of multichannel MI EEG signals.
更多查看译文
关键词
Weighted Vector visibility (WVV), graph signal processing (GSP), brain computer interface (BCI), Motor Imagery (MI) EEG, Laplacian energy, Fiedler energy
AI 理解论文
溯源树
样例
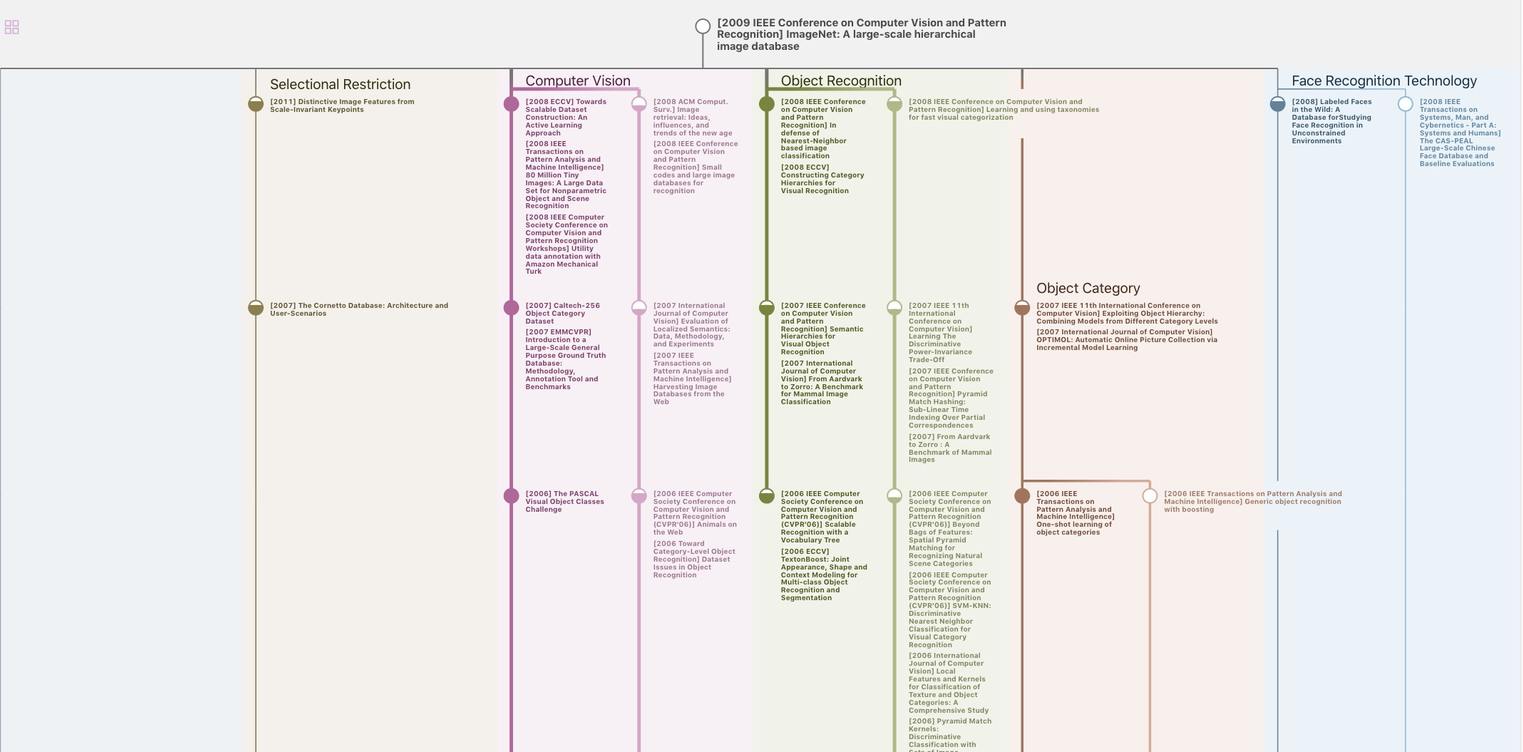
生成溯源树,研究论文发展脉络
Chat Paper
正在生成论文摘要