Research on Error Calibration Method for Power Big Data Based on K-Means Clustering
2022 9TH INTERNATIONAL FORUM ON ELECTRICAL ENGINEERING AND AUTOMATION, IFEEA(2022)
摘要
For heterogeneous data with large volume, the current error checking method has lower effective error correction and longer checking processing time. In view of the above problems, this paper studies the power big data error calibration method based on K-Means clustering. After processing the heterogeneous and heterogeneous power big data, the PSO algorithm is used to improve the k-means clustering process. After extracting the data error features in the sparse coding network, the improved k-means algorithm is used to achieve error checking. In the experimental study, the effective calibration ratio of the k-means-based calibration method is higher than 65%, and the calibration time is greatly reduced. When the method is applied to actual power big data management, the influence of errors on data analysis can be reduced to the greatest extent, and the data credibility can be improved.
更多查看译文
关键词
K-Means clustering, Power big data, Error checking, PSO algorithm, Sparse coding network
AI 理解论文
溯源树
样例
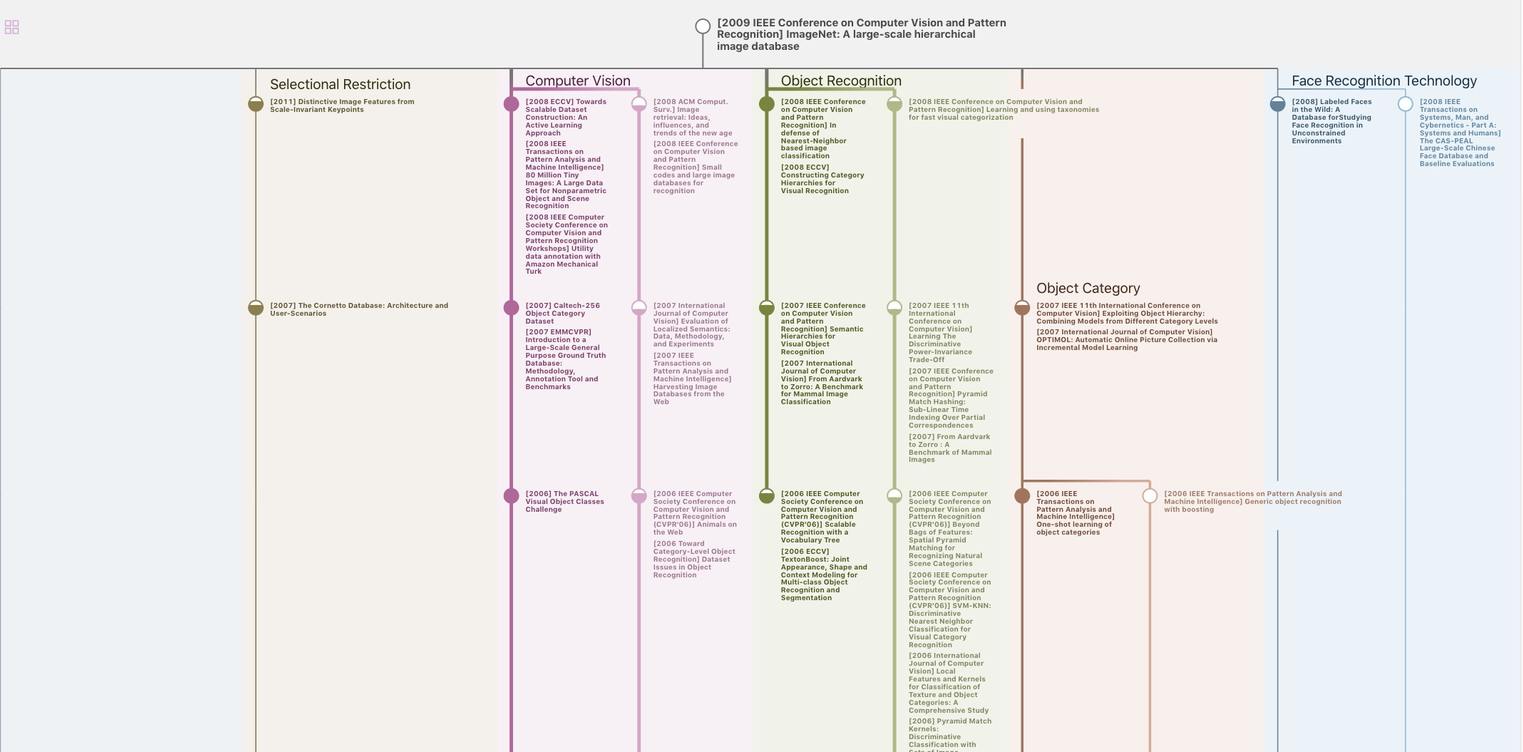
生成溯源树,研究论文发展脉络
Chat Paper
正在生成论文摘要