Label Correlation Embedding Guided Network for Multi-Label ECG Arrhythmia Diagnosis
Knowledge-based systems(2023)
摘要
In clinical practice, one patient may suffer from more than one arrhythmia simultaneously, that is, one ECG record may be associated with multiple types of arrhythmias. In fact, there are inherent dependencies between arrhythmias. However, previous studies have mainly focused on multi-class (single-label) ECG classification, which addresses each type of arrhythmia independently and ignores the multi-label correlation between different ECG abnormalities. To address the lack of ECG multi-label classification methods, we proposed a label correlation embedding guided network (LCEGNet) model to effectively recognize multi-label ECG arrhythmias and explore the correlation between ECG abnormalities. First, label correlation embedding was obtained based on the correlation matrix between different arrhythmias to guide feature extraction. Subsequently, the category-specific attention coefficient was obtained by calculating the cosine similarity coefficient between the label embedding and feature spaces. Experiments on public and self-collected ECG datasets were conducted. The LCEGNet achieved F1 scores of 0.777 and 0.872 and subset accuracy of 0.750 and 0.828 on the two datasets, respectively. A classification speed of 7.796ms was achieved. The experimental results demonstrate that the proposed LCEGNet achieved approximately a 11% and 9.1% improvement in the F1 score and subset accuracy, respectively, compared with traditional ResNet architecture and a 4.3% and 5.54% improvement in the F1 score and subset accuracy, respectively, compared with the state-of-the-art approaches.
更多查看译文
关键词
Deep learning,Electrocardiography (ECG),Multi-label classification,Label correlation,Convolutional neural network
AI 理解论文
溯源树
样例
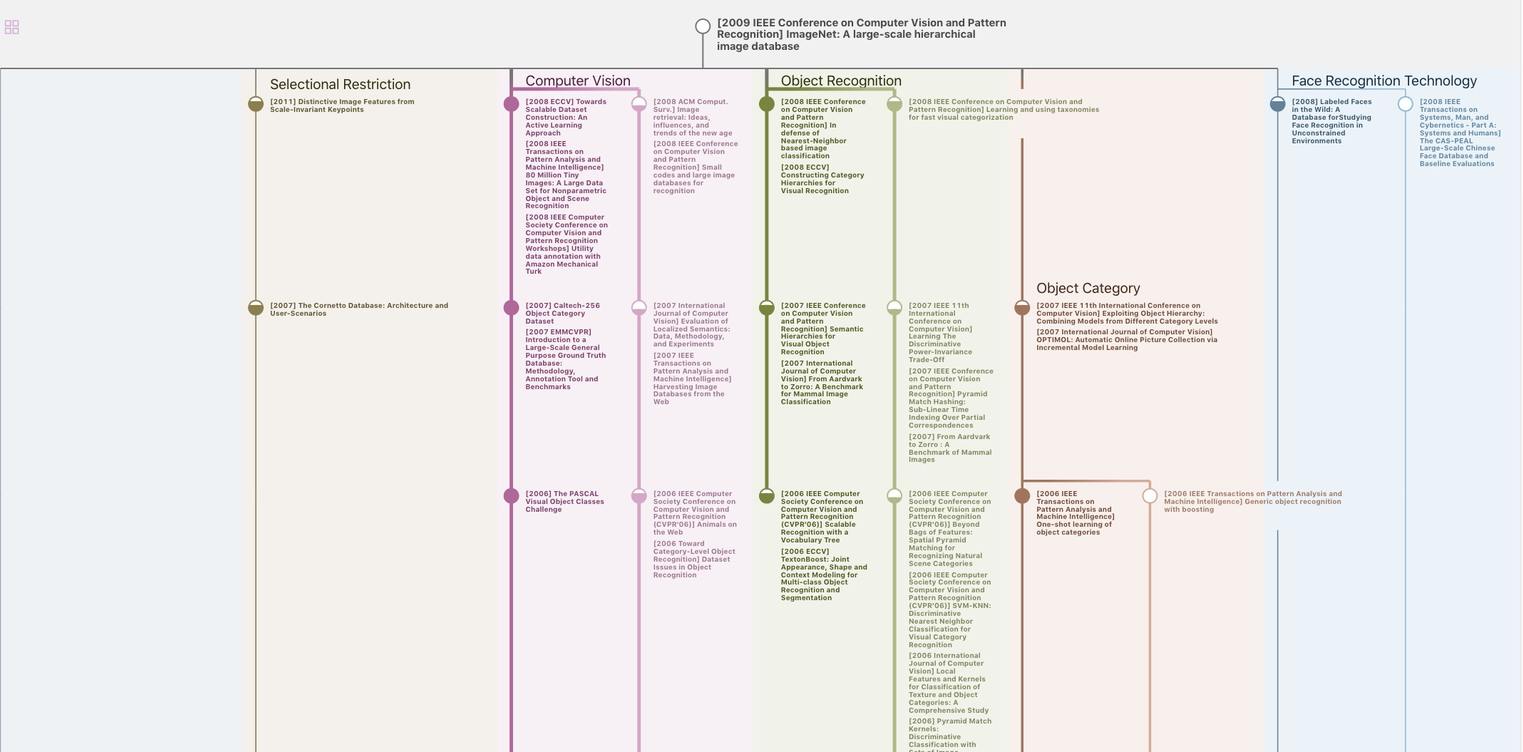
生成溯源树,研究论文发展脉络
Chat Paper
正在生成论文摘要