RKHS subspace domain adaption via minimum distribution gap
PATTERN ANALYSIS AND APPLICATIONS(2023)
摘要
Subspace learning of Reproducing Kernel Hilbert Space (RKHS) is most popular among domain adaption applications. The key goal is to embed the source and target domain samples into a common RKHS subspace where their distributions could match better. However, most existing domain adaption measures are either based on the first-order statistics that can’t accurately qualify the difference of distributions for non-Guassian distributions or complicated co-variance matrix that is difficult to be used and optimized. In this paper, we propose a neat and effective RKHS subspace domain adaption measure: Minimum Distribution Gap (MDG), where the rigorous mathematical formula can be derived to learn the weighting matrix of the optimized orthogonal Hilbert subspace basis via the Lagrange Multiplier Method. To show the efficiency of the proposed MDG measure, extensive numerical experiments with different datasets have been performed and the comparisons with four other state-of-the-art algorithms in the literature show that the proposed MDG measure is very promising.
更多查看译文
关键词
Domain adaption,RKHS,Maximum mean difference (MMD),Lagrange multiplier method (LMM) optimization
AI 理解论文
溯源树
样例
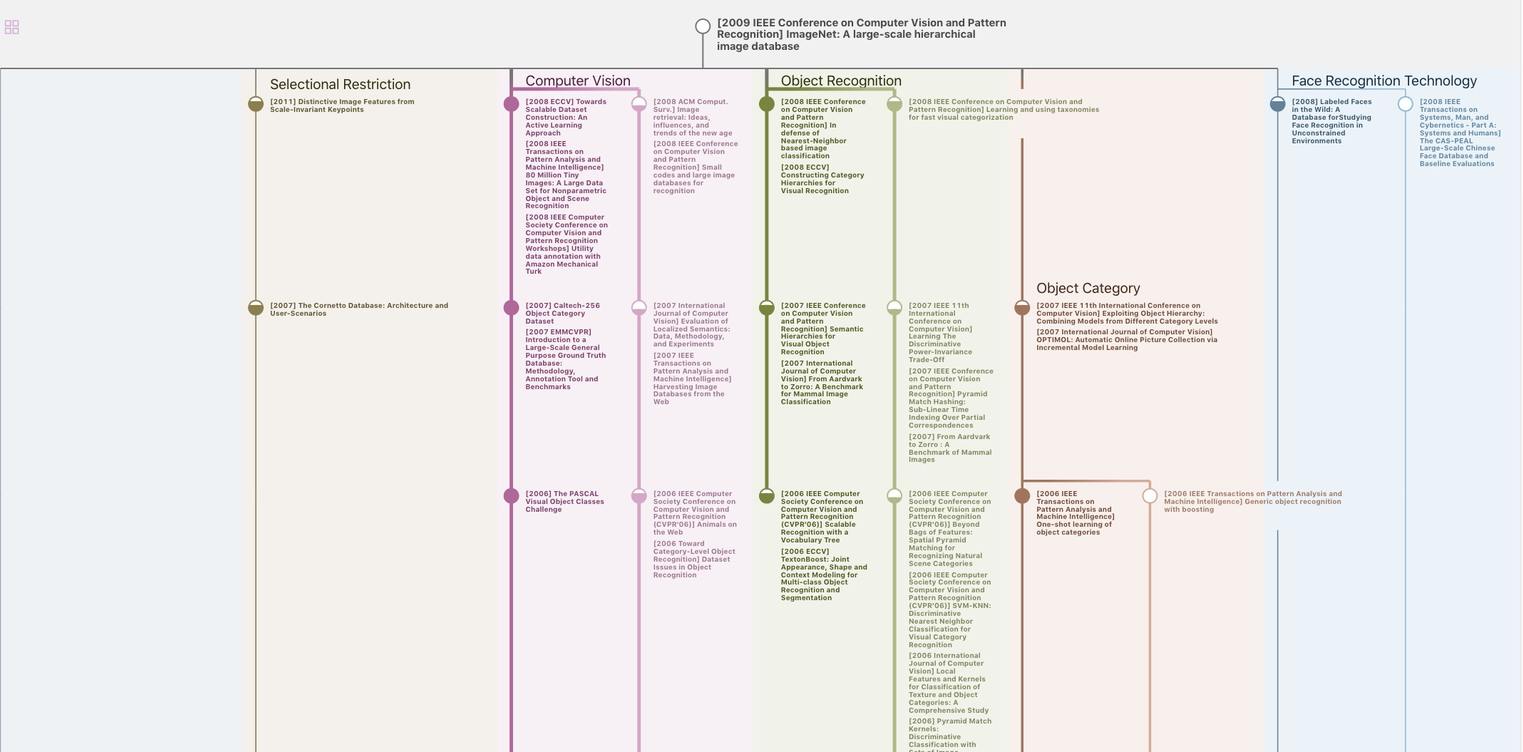
生成溯源树,研究论文发展脉络
Chat Paper
正在生成论文摘要