Integrating information by Kullback–Leibler constraint for text classification
NEURAL COMPUTING & APPLICATIONS(2023)
摘要
Text classification is an important assignment for various text-related downstream assignments, such as fake news detection, sentiment analysis, and question answering. In recent years, the graph-based method achieves excellent results in text classification tasks. Instead of regarding a text as a sequence structure, this method regards it as a co-occurrence set of words. The task of text classification is then accomplished by aggregating the data from nearby nodes using the graph neural network. However, existing corpus-level graph models are difficult to incorporate the local semantic information and classify new coming texts. To address these issues, we propose a Global–Local Text Classification (GLTC) model, based on the KL constraints to realize inductive learning for text classification. Firstly, a global structural feature extractor and a local semantic feature extractor are designed to capture the structural and semantic information of text comprehensively. Then, the KL divergence is introduced as a regularization term in the loss calculation process, which ensures that the global structural feature extractor can constrain the learning of the local semantic feature extractor to achieve inductive learning. The comprehensive experiments on benchmark datasets present that GLTC outperforms baseline methods in terms of accuracy.
更多查看译文
关键词
Text classification,Graph neural network,Kullback–Leibler divergence,Constraint
AI 理解论文
溯源树
样例
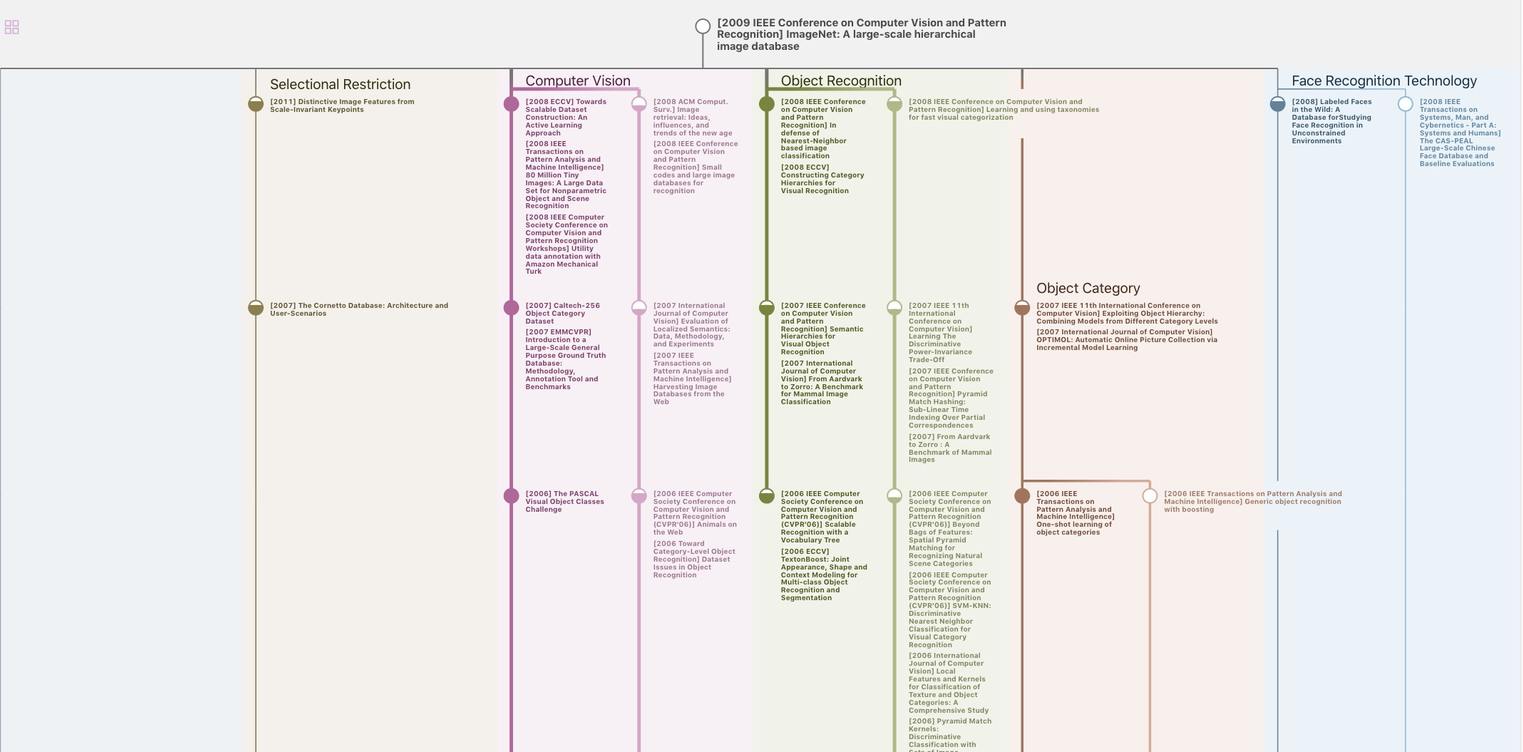
生成溯源树,研究论文发展脉络
Chat Paper
正在生成论文摘要