Optimizing traffic efficiency via a reinforcement learning approach based on time allocation
INTERNATIONAL JOURNAL OF MACHINE LEARNING AND CYBERNETICS(2023)
摘要
With the increasing scale of urbanization, traffic congestion has caused a severe negative impact on the efficiency of social development. To this end, a series of intelligent traffic light control methods based on reinforcement learning are proposed. They get superior performance compared with conventional control methods under certain conditions. However, because of the usage of actions based on switching phase, almost all of these methods cannot provide a countdown function, for the switching phase actions need to be executed immediately. So they are difficult to be applied in most real-world scenarios from the practical consideration of traffic safety and efficiency. For example, without the countdown function, it cannot inform pedestrians how many seconds the green light remains to cross the road. This paper proposes a novel method that can naturally provide a countdown function by adopting a new action design. Specifically, this action design achieves control in the manner of time allocation, and the model figures out the duration of each phase at the beginning of every signal cycle. In this way, our method is more practical for real-world traffic applications. Plenty of simulation experiments show that our method eases congestion substantially in single intersection environments with the countdown requirement, e.g., our model cuts down 74% waiting time compared with a competitive baseline in the experiment with 2 phases and mix flow.
更多查看译文
关键词
Reinforcement learning,Traffic light control,Time allocation,Countdown function
AI 理解论文
溯源树
样例
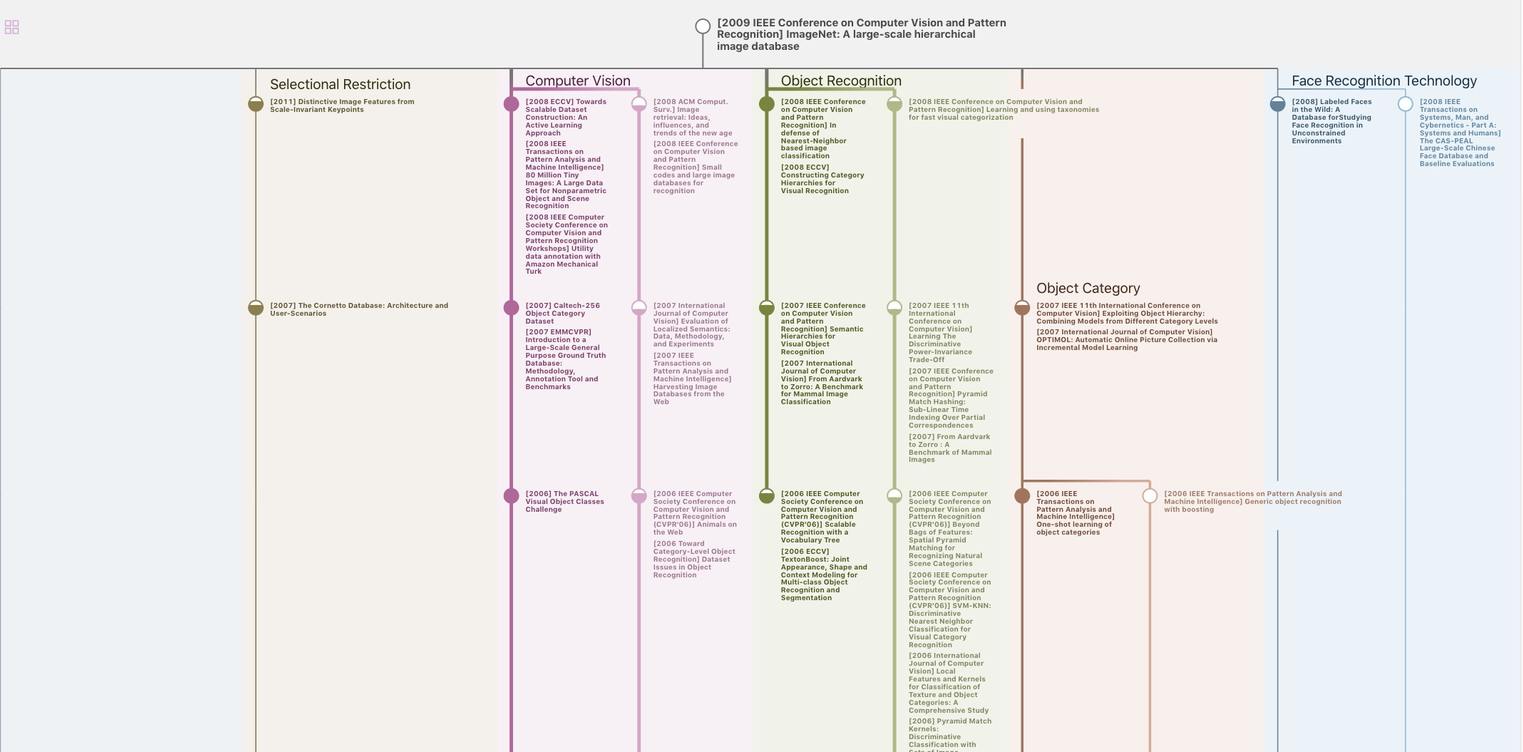
生成溯源树,研究论文发展脉络
Chat Paper
正在生成论文摘要