SGMA: a Novel Adversarial Attack Approach with Improved Transferability
COMPLEX & INTELLIGENT SYSTEMS(2023)
摘要
Deep learning models are easily deceived by adversarial examples, and transferable attacks are crucial because of the inaccessibility of model information. Existing SOTA attack approaches tend to destroy important features of objects to generate adversarial examples. This paper proposes the split grid mask attack (SGMA), which reduces the intensity of model-specific features by split grid mask transformation, effectively highlighting the important features of the input image. Perturbing these important features can guide the development of adversarial examples in a more transferable direction. Specifically, we introduce the split grid mask transformation into the input image. Due to the vulnerability of model-specific features to image transformations, the intensity of model-specific features decreases after aggregation while the intensities of important features remain. The generated adversarial examples guided by destroying important features have excellent transferability. Extensive experimental results demonstrate the effectiveness of the proposed SGMA. Compared to the SOTA attack approaches, our method improves the black-box attack success rates by an average of 6.4% and 8.2% against the normally trained models and the defense ones respectively.
更多查看译文
关键词
Deep neural networks,Feature-level attack,Adversarial examples,Transferable attack
AI 理解论文
溯源树
样例
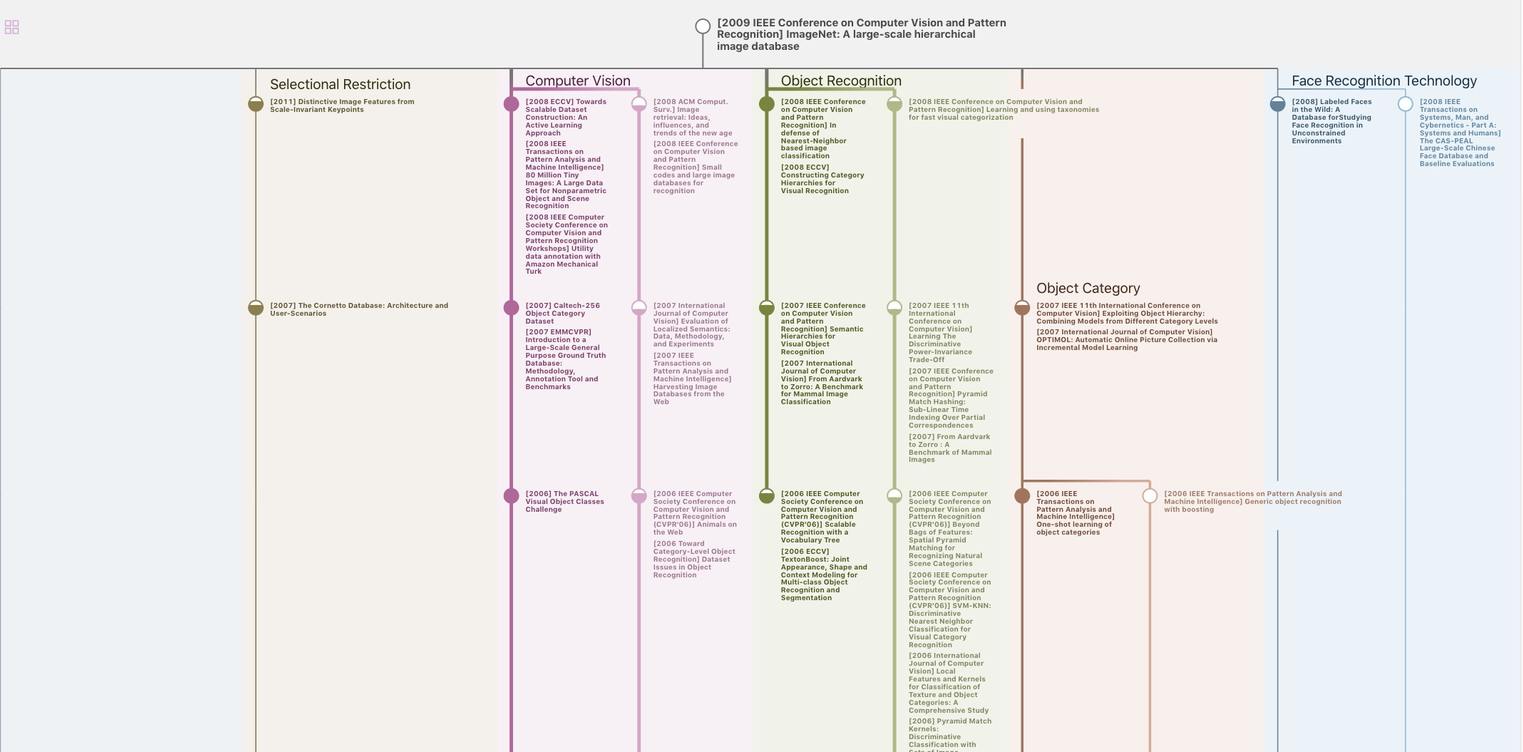
生成溯源树,研究论文发展脉络
Chat Paper
正在生成论文摘要