Improving Regularization in Deep Neural Networks by Co-adaptation Trace Detection
NEURAL PROCESSING LETTERS(2023)
摘要
Co-adaptation of units is one of the most critical concerns in deep neural networks (DNNs), which leads to overfitting. Dropout has been an exciting research subject in recent years to prevent overfitting. In previous studies, the dropout probability keeps fixed or changes simply during training epochs. However, we have no evidence which proves that the co-adaptation is uniformly distributed among units of models. Therefore, Dropout with identical probability for all units leads to an imbalance regularization and the under-/over-dropping problem. This paper proposes DropCT, a variant of Dropout that can detect co-adaptation traces (CTs) among units using the label propagation algorithm in community detection. It determines the DropCT probability of units in a CT according to its co-adaptation pressure. Therefore, DropCT applies a dynamic regularization to avoid under-/over-dropping. DropCT can integrate with different architectures as a general regularization method. Experimental results confirm that DropCT improves generalization and is comparatively simple to apply without tuning regularization hyperparameters.
更多查看译文
关键词
Deep neural networks,Regularization,Dropout,Co-adaptation,Under-,over-dropping
AI 理解论文
溯源树
样例
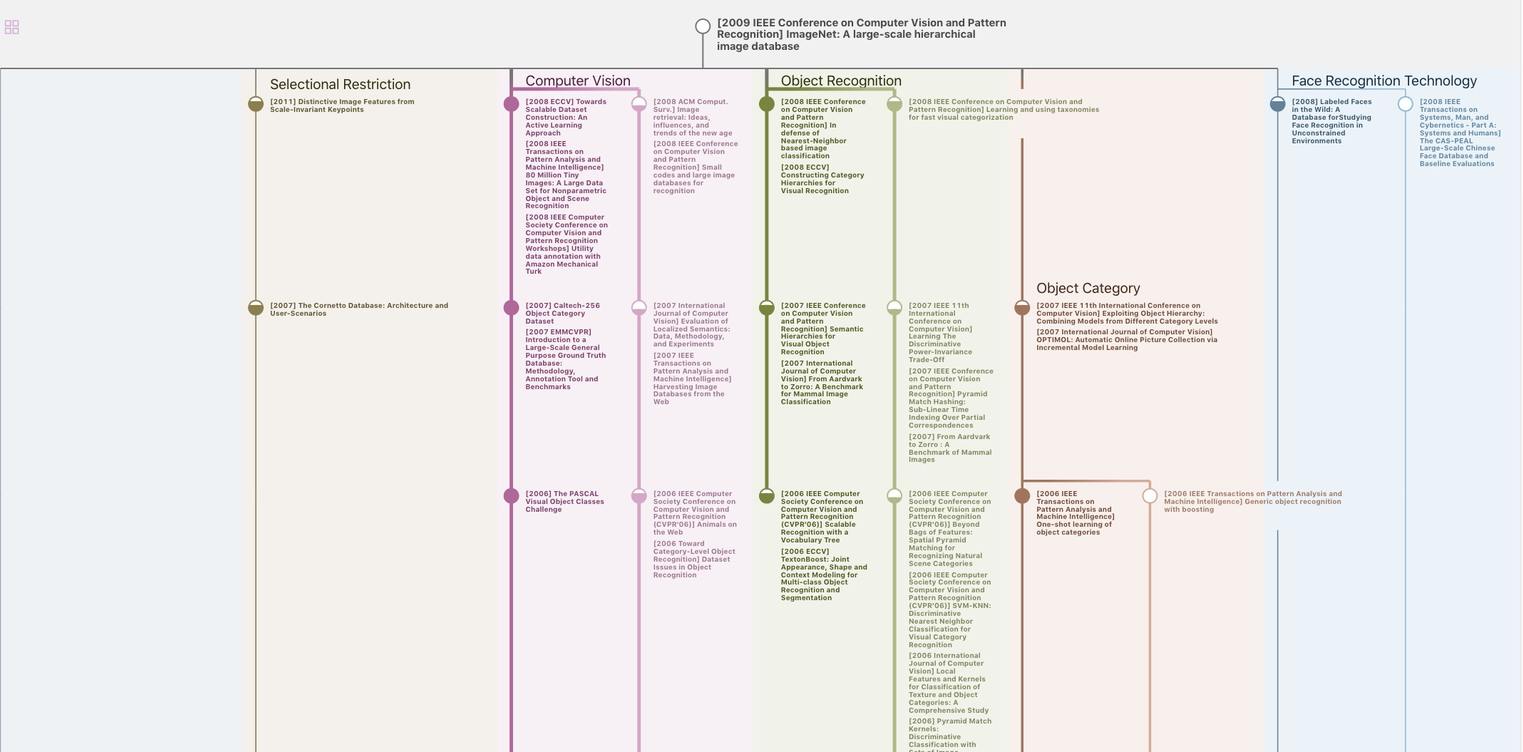
生成溯源树,研究论文发展脉络
Chat Paper
正在生成论文摘要