Analytic Approaches to Handle Missing Data in Simple Matrix Sampling Planned Missing Designs
JOURNAL OF EXPERIMENTAL EDUCATION(2023)
摘要
Simple matrix sampling planned missing (SMS PD) design, introduce missing data patterns that lead to covariances between variables that are not jointly observed, and create difficulties for analyses other than mean and variance estimations. Based on prior research, we adopted a new multigroup confirmatory factor analysis (CFA) approach to handle missing data in such designs, in comparison to a regular CFA with full information maximum likelihood estimator. In Study 1, we tested the two approaches in 36 scenarios (4 sample sizes x 3 inter-item correlations x 3 numbers of x-set items) given a total of 20 items. We found that, the multigroup CFA approach performed with acceptable convergence rates, power to recover population values, acceptable standard errors and model fit in certain scenarios by larger sample size, higher bivariate correlation, and more items in the x-set. We found a few scenarios where regular CFA with FIML performed well. These findings suggested that the approaches can be implemented to handle the special missing data introduced by the SMS PM designs, and, thereby, enhance the utility of SMS PM data. In Study 2, we applied the multigroup CFA approach in real-world data to demonstrate the feasibility and analytic value of this approach.
更多查看译文
关键词
Full information maximum likelihood, missing data, multigroup confirmatory factor analysis, planned missing designs, simple matrix sampling
AI 理解论文
溯源树
样例
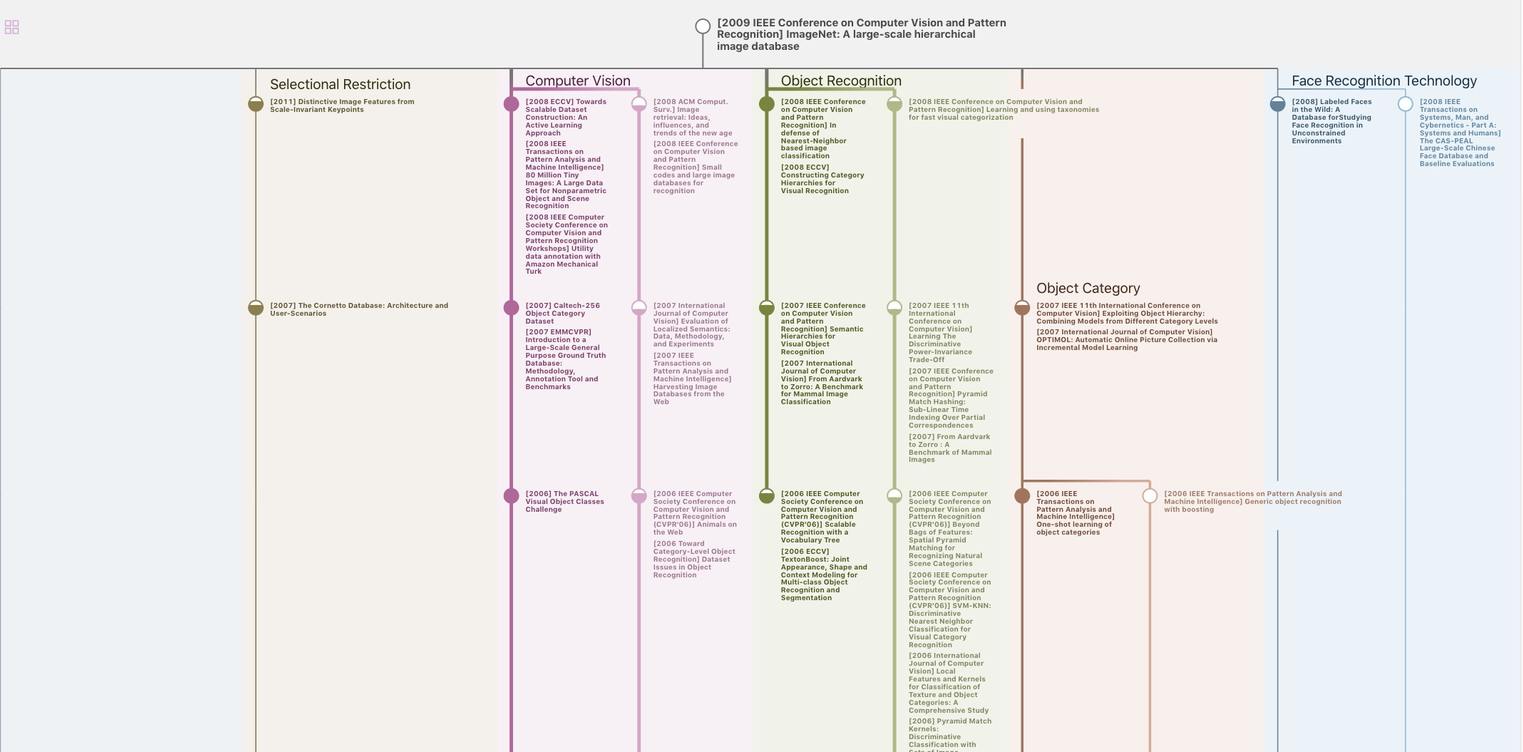
生成溯源树,研究论文发展脉络
Chat Paper
正在生成论文摘要