DeepApp: characterizing dynamic user interests for mobile application recommendation
WORLD WIDE WEB-INTERNET AND WEB INFORMATION SYSTEMS(2023)
摘要
It is extremely difficult to find one app in app stores that exactly meets the needs of users with the boom in mobile applications nowadays. Although numerous app recommendation services are available, they mainly employ static data (e.g., information of installed apps) and rarely consider the dynamics of user interests. In this paper, we assume that user interest consists of two components: short-term temporal interests and long-term preferences, and we propose one general framework, namely DeepApp, to enhance app recommendation performance. In DeepApp, on the one hand, we use one linear model to characterize the stable user-app associations; on the other hand, we employ the Long Short-Term Memory (LSTM) model to capture the evolution of interests based on the usage patterns of mobile apps. Finally, the Wide &Deep model is applied to fuse the effects of these two types of interests, long-term preferences and short-term temporal interests, by learning the latent interaction between linear and nonlinear features. DeepApp was evaluated on a large-scale dataset, with 4,775,293 users and 238,206 mobile apps. DeepApp achieves a significant performance gain compared with baselines (more than 6% in terms of NDCG@6 over probability matrix factorization (PMF), neural collaborative filtering (NCF), and neural tensor factorization (NTF)). This demonstrates that the integration of dynamic user interests is beneficial for mobile app recommendations.
更多查看译文
关键词
Mobile application recommendation, Dynamic interests, Wide& Deep
AI 理解论文
溯源树
样例
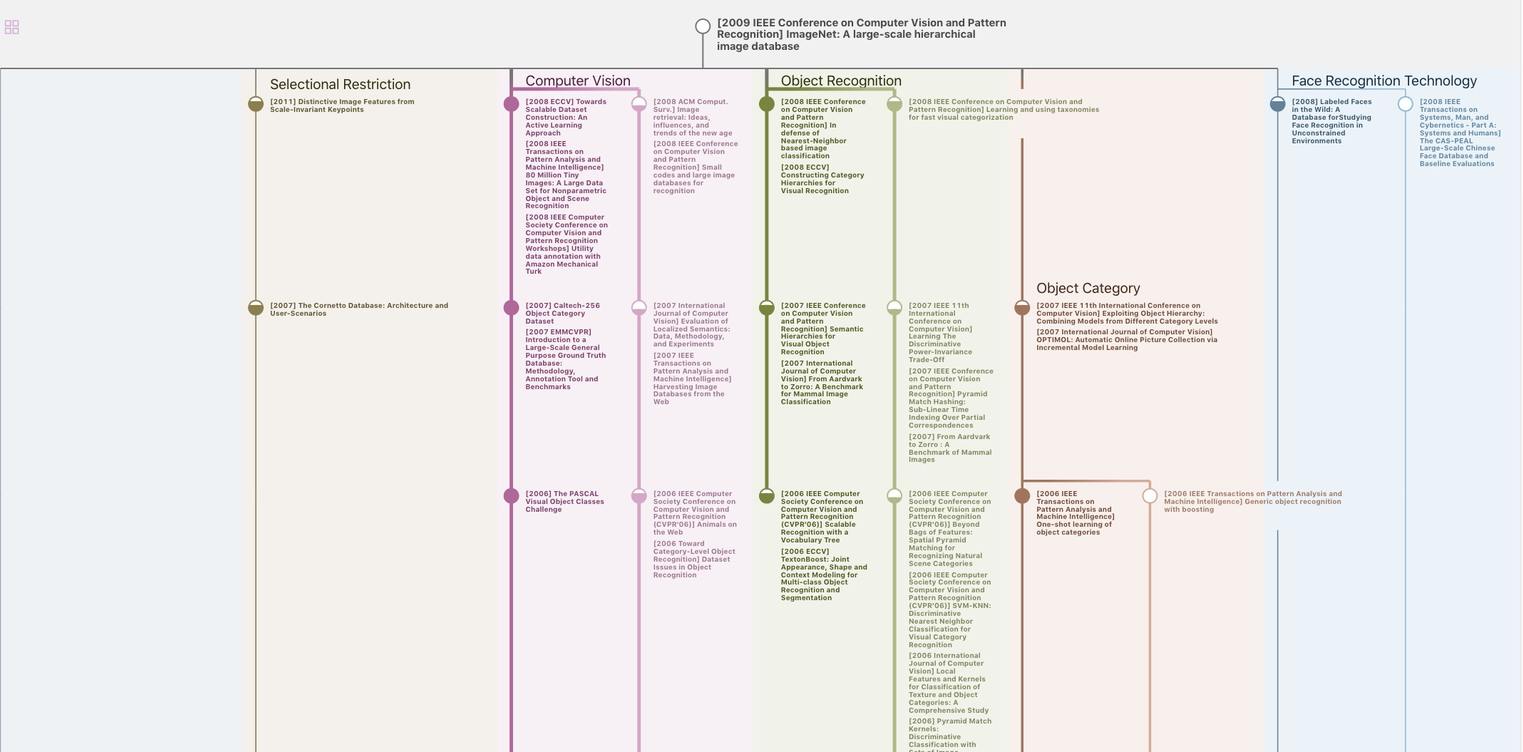
生成溯源树,研究论文发展脉络
Chat Paper
正在生成论文摘要