Global-guided weakly-supervised learning for multi-label image classification*
JOURNAL OF VISUAL COMMUNICATION AND IMAGE REPRESENTATION(2023)
摘要
Multi-label classification with region-free labels is attracting increasing attention compared to that with region-based labels due to the time-consuming manual region-labeling process. Existing methods usually employ attention-based technology to discover the conspicuous label-related regions in a weakly-supervised manner with only image-level region-free labels, while the region covering is not precise without exploring global clues of multi-level features. To address this issue, a novel Global-guided Weakly-Supervised Learning (GWSL) method for multi-label classification is proposed. The GWSL first extracts the multi-level features to estimate their global correlation map which is further utilized to guide feature disentanglement in the proposed Feature Disentanglement and Localization (FDL) networks. Specifically, the FDL networks then adaptively combine the different correlated features and localize the fine-grained features for identifying multiple labels. The proposed method is optimized in an end-to-end manner under weakly supervision with only image-level labels. Experimental results demonstrate that the proposed method outperforms the state-of-the-arts for multi-label learning problems on several publicly available image datasets. To facilitate similar researches in the future, the codes are directly available online at https://github.com/Yong-DAI/GWSL.
更多查看译文
关键词
Global correlation Feature disentanglement Label-related regions Weakly-supervised learning Multi-label classification
AI 理解论文
溯源树
样例
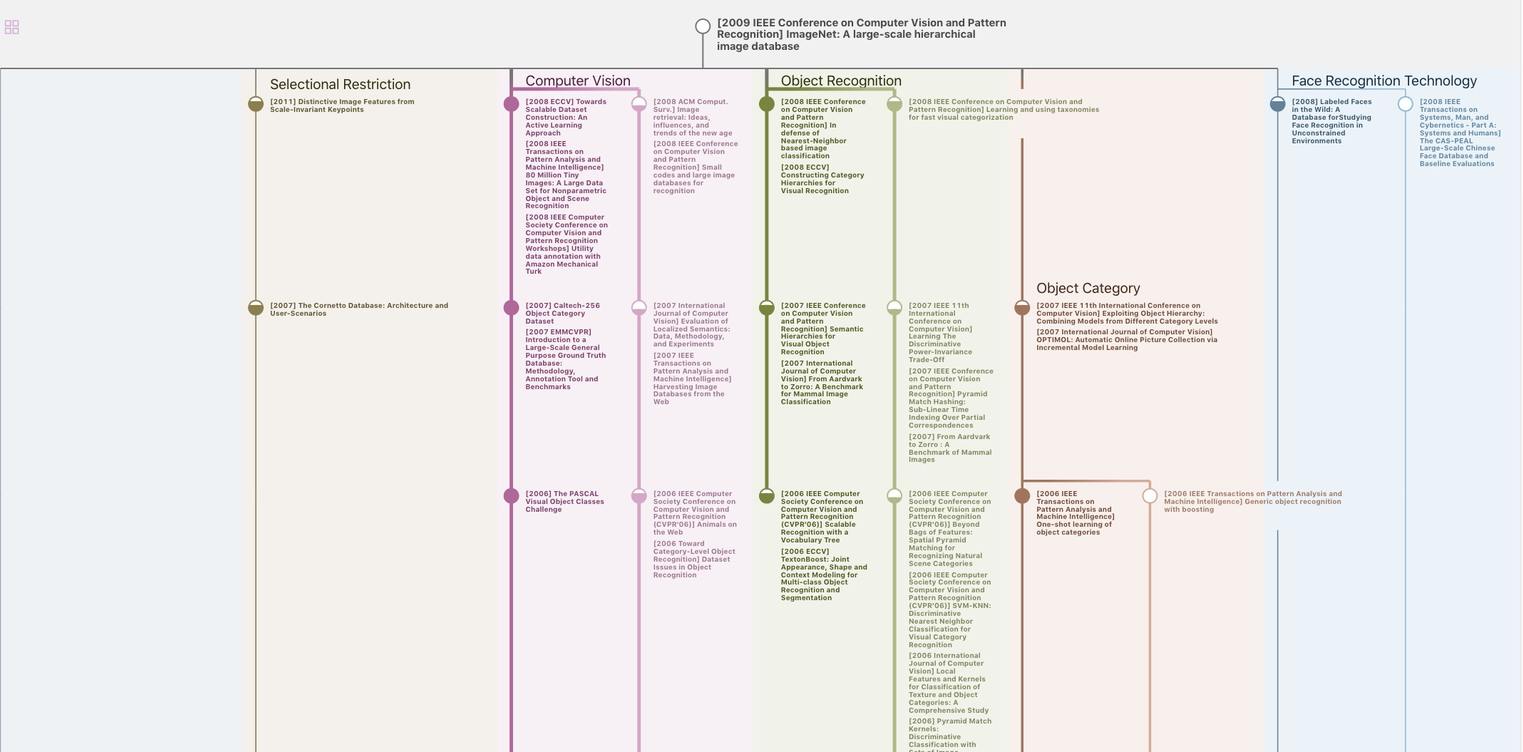
生成溯源树,研究论文发展脉络
Chat Paper
正在生成论文摘要