Robustness challenges in Reinforcement Learning based time-critical cloud resource scheduling: A Meta-Learning based solution
Future Generation Computer Systems(2023)
摘要
Cloud computing attracts increasing attention in processing dynamic computing tasks and automating the software development and operation pipeline. In many cases, the computing tasks have strict deadlines. The cloud resource manager (e.g., orchestrator) effectively manages the resources and provides tasks Quality of Service (QoS). Cloud task scheduling is tricky due to the dynamic nature of task workload and resource availability. Reinforcement Learning (RL) has attracted lots of research attention in scheduling. However, those RL-based approaches suffer from low scheduling performance robustness when the task workload and resource availability change, particularly when handling time-critical tasks. This paper focuses on both challenges of robustness and deadline guarantee among such RL, specifically Deep RL (DRL)-based scheduling approaches. We quantify the robustness measurements as the retraining time and investigate how to improve both robustness and deadline guarantee of DRL-based scheduling. We propose MLR-TC-DRLS, a practical, robust Meta Deep Reinforcement Learning-based scheduling solution to provide time-critical tasks deadline guarantee and fast adaptation under highly dynamic situations. We comprehensively evaluate MLR-TC-DRLS performance against RL-based and RL advanced variants-based scheduling approaches using real-world and synthetic data. The evaluations validate that our proposed approach improves the scheduling performance robustness of typical DRL variants scheduling approaches with 97%–98.5% deadline guarantees and 200%–500% faster adaptation.
更多查看译文
关键词
Robustness,Reinforcement Learning,Meta Learning,Resource management,Task scheduling,Cloud computing
AI 理解论文
溯源树
样例
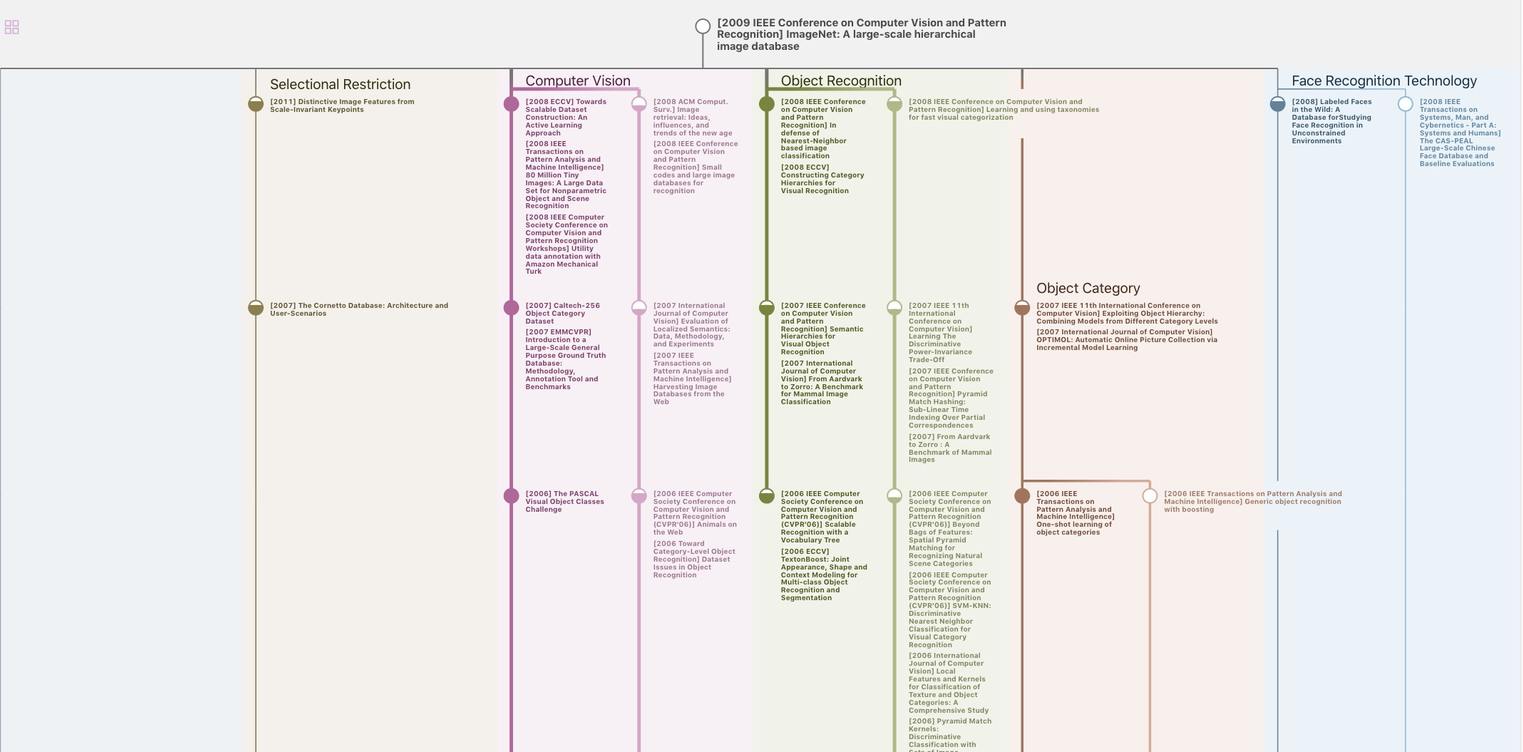
生成溯源树,研究论文发展脉络
Chat Paper
正在生成论文摘要