GM2NAS: multitask multiview graph neural architecture search
Knowledge and Information Systems(2023)
摘要
Graph neural network-based multitask learning models on multiview graphs have achieved acceptable results in different real-world applications. However, constructing and fine-tuning artificially designed architectures for various multiview graphs are time-consuming and require expert knowledge. To address this challenge, we propose a multitask multiview graph neural architecture search framework called GM2NAS to automatically design multitask multiview model (M2 model) architectures. Specifically, the GM2NAS framework builds M2 model architectures for multiview graph learning and multitask learning. Unlike traditional graph neural architecture search (GNAS) approaches developed for single-task single-view problems, we design a multitask multiview (M2) search space and an unsupervised evaluation strategy to fit GNAS for multitask multiview graph learning. In terms of the search space, we design an effective multitask multiview (M2) search space that precisely allows identifying the optimal operations for multiview graph learning, multiview representation fusion, task-specific attention, and loss weighting to capture informative representation and implicitly transfer and share information among multiple tasks. In terms of the unsupervised evaluation strategy, we introduce an unsupervised evaluation strategy based on unsupervised learning to guide the search algorithm and enable GNAS to deal with multitask multiview graph learning effectively. Then, we explore different search algorithms to identify the optimal combinations of M2 models for multitask multiview graph learning. To validate the effectiveness of GM2NAS, we apply it to node classification and link prediction tasks. Based on the extensive experiments, the results reveal that GM2NAS outperforms the state-of-the-art models on actual multiview graph data.
更多查看译文
关键词
Graph neural network,Graph neural architecture search,Multitask multiview learning,Automatic machine learning
AI 理解论文
溯源树
样例
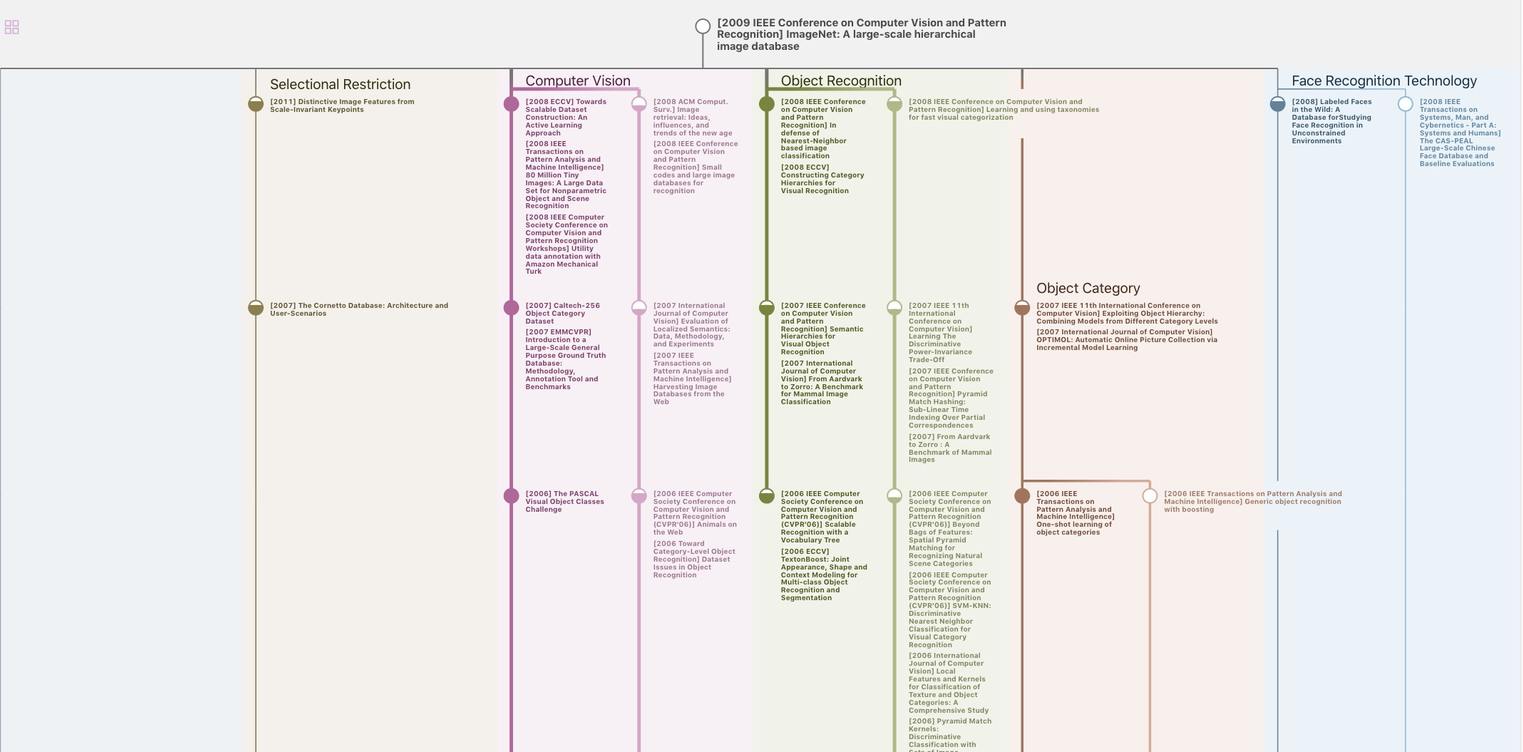
生成溯源树,研究论文发展脉络
Chat Paper
正在生成论文摘要