A fast spatio-temporal temperature predictor for vacuum assisted resin infusion molding process based on deep machine learning modeling
JOURNAL OF INTELLIGENT MANUFACTURING(2023)
摘要
The manufacture of large wind turbine blades requires well-controlled processing conditions to prevent defect formation and thus produce high-quality composite blades. While the physics-based models provide accurate computational capabilities for the resin infusion and curing process for the glass fiber composites, they suffer from high computational costs, making them infeasible for fast optimization computation and process control during manufacturing. In light of the limitations, we describe a machine learning (ML) approach that employs a deep convolutional and recurrent neural network model to predict the spatio-temporal temperature distribution during the vacuum assisted resin infusion molding (VARIM) process. The ML model is trained with the "big data" generated from the physics-based high-fidelity simulations. Once fully trained, it serves as a digital twin of the blade manufacturing process. Validation is made by comparing simulation results with experimental data on a unidirectional glass fiber composite laminate plate (44 plies, 2 m long and 0.5 m wide). The trained and validated ML model is then extended to evaluate the role of critical VARIM processing parameters on temperature distribution. With the predictive accuracy of 94%, at over 100 times faster computational speed than the physics-based simulations, the ML approach established herein provides a general framework for a digital twin for temperature distribution in the composite manufacturing process.
更多查看译文
关键词
Vacuum assisted resin infusion molding (VARIM),Machine learning (ML),Deep convolutional neural network (CNN),Recurrent neural network (RNN),Long short-term memory (LSTM),Physics-informed surrogate model
AI 理解论文
溯源树
样例
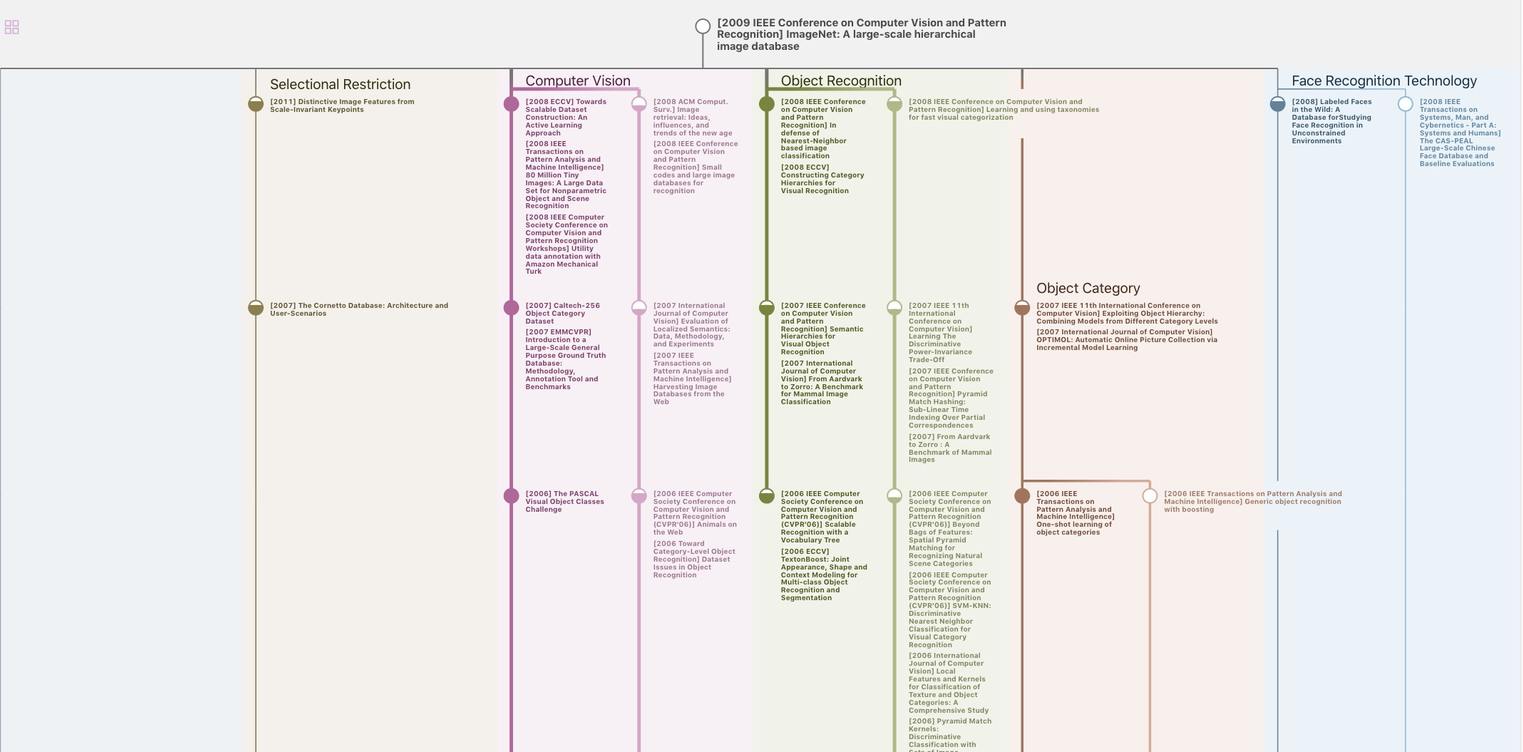
生成溯源树,研究论文发展脉络
Chat Paper
正在生成论文摘要